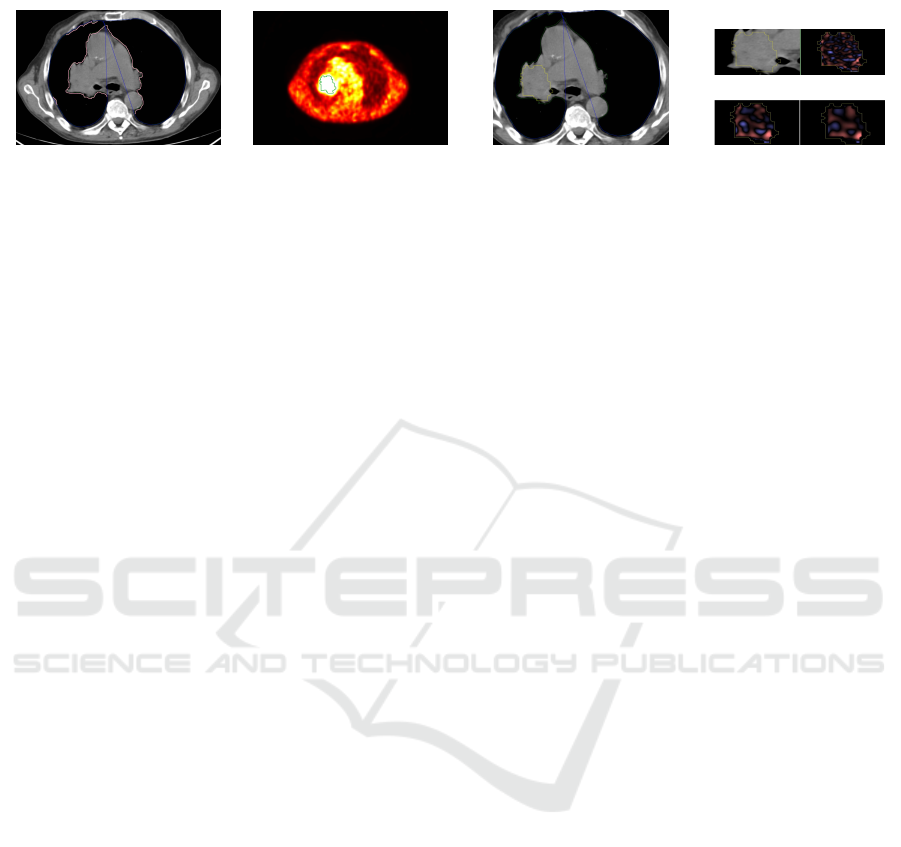
(a) Lungs in CT (b) Hot-spots in PET (c) Transfer (PET→CT) (d) Texture analysis
(i) Original
CT
(ii) Fine
texture
(iii) Medium
texture
(iv) Coarse
texture
Figure 1: Example images generated at the most important steps of the PET/CT analysis: (a) lungs segmented in CT (light
pink ROIs indicate lungs, whereas blue ROIs show their convex hulls), (b) hot-spot segmented in PET, (c) hot-spot transferred
from PET to CT (annotated in yellow), and (d) texture analysis at various scales (fine, medium and coarse) using the TexRAD
algorithm. We boldfaced the steps in which we benefit from transferring the information between two modalities.
pling complementary patient information (e.g., ana-
tomical and functional). It leads to extracting new in-
formation about the patient condition and treatment
response, which would not be revealed if the images
were processed separately.
ACKNOWLEDGEMENTS
This research was supported by the National
Centre for Research and Development under the
Innomed Research and Development Grant No.
POIR.01.02.00-00-0030/15.
REFERENCES
Deregnaucourt, T., Samir, C., and Yao, A.-F. (2016). A
regression model for registering multimodal images.
Procedia Computer Science (MIUA 2016), 90:42 – 47.
Estorch, M. and Carrio, I. (2013). Future challenges of mul-
timodality imaging. In Schober, O. and Riemann, B.,
editors, Mol. Imag. in Onc., pages 403–415, Heidel-
berg. Springer.
Greulich, S. and Sechtem, U. (2015). Multimodality ima-
ging in coronary artery disease – “the more the bet-
ter?”. Cor et Vasa, 57(6):e462 – e469.
Mart
´
ı-Bonmat
´
ı, L., Sopena, R., Bartumeus, P., and Sopena,
P. (2010). Multimodality imaging techniques. Con-
trast Media & Molecular Imaging, 5(4):180–189.
Miles, K., Ganeshan, B., and Hayball, M. (2013). CT
texture analysis using the filtration-histogram met-
hod: what do the measurements mean? Canc. Im.,
13(3):400–406.
Mokri, S. S., Saripan, M. I., Marhaban, M. H., and Nordin,
A. J. (2012). Lung segmentation in CT for thoracic
PET-CT registration through visual study. In Proc.
IEEE-EMBS, pages 550–554.
Nalepa, J., Szymanek, J., Mcquaid, S., Endozo, R., Pra-
kash, V., Ganeshan, B., Menys, A., Hayball, M. P.,
Ezhil, V., Bellamy, L., Crawshaw, J., Hall, J., Groves,
A. M., and Nisbet, A. (2016). PET/CT in lung cancer:
An automated imaging tool for decision support. In
Radiological Society of North America 2016 Scienti-
fic Assembly and Annual Meeting, pages 1–2, Chicago
IL, USA. RSNA.
Parikh, J., Selmi, M., Charles-Edwards, G., Glendenning,
J., Ganeshan, B., Verma, H., Mansi, J., Harries, M.,
Tutt, A., and Goh, V. (2014). Changes in primary bre-
ast cancer heterogeneity may augment midtreatment
MR imaging assessment of response to neoadjuvant
chemotherapy. Radiology, 272(1):100–112.
Pichler, B. J., Judenhofer, M. S., and Pfannenberg, C.
(2008). Multimodal imaging approaches: PET/CT
and PET/MRI. In Semmler, W. and Schwaiger, M.,
editors, Molecular Imaging I, pages 109–132, Heidel-
berg. Springer.
Radulescu, E., Ganeshan, B., Shergill, S. S., Medford,
N., Chatwin, C., Young, R. C., and Critchley, H. D.
(2014). Grey-matter texture abnormalities and redu-
ced hippocampal volume are distinguishing features
of schizophrenia. Psychiatry Research: Neuroima-
ging, 223(3):179 – 186.
Sui, J., Adali, T., Yu, Q., Chen, J., and Calhoun, V. D.
(2012). A review of multivariate methods for mul-
timodal fusion of brain data. J. of Neurosc. Met.,
204(1):68 – 81.
Vandenberghe, S. and Marsden, P. K. (2015). PET-MRI:
a review of challenges and solutions in the develop-
ment of integrated multimodality imaging. Physics in
Medicine and Biology, 60(4):R115.
Weiss, G. J., Ganeshan, B., Miles, K. A., Campbell, D. H.,
Cheung, P. Y., Frank, S., and Korn, R. L. (2014).
Noninvasive image texture analysis differentiates k-
ras mutation from pan-wildtype nsclc and is prognos-
tic. PLOS ONE, 9(7):1–9.
Win, T., Miles, K. A., Janes, S. M., Ganeshan, B., Shastry,
M., Endozo, R., Meagher, M., Shortman, R. I., Wan,
S., Kayani, I., Ell, P. J., and Groves, A. M. (2013).
Tumor heterogeneity and permeability as measured on
the ct component of PET/CT predict survival in pa-
tients with non–small cell lung cancer. Clin. Cancer
Res., 19(13):3591–3599.
Zhao, J., Ji, G., Qiang, Y., Han, X., Pei, B., and Shi, Z.
(2015). A new method of detecting pulmonary no-
dules with PET/CT based on an improved watershed
algorithm. PLOS ONE, 10(4):1–15.
Transferring Information Across Medical Images of Different Modalities
533