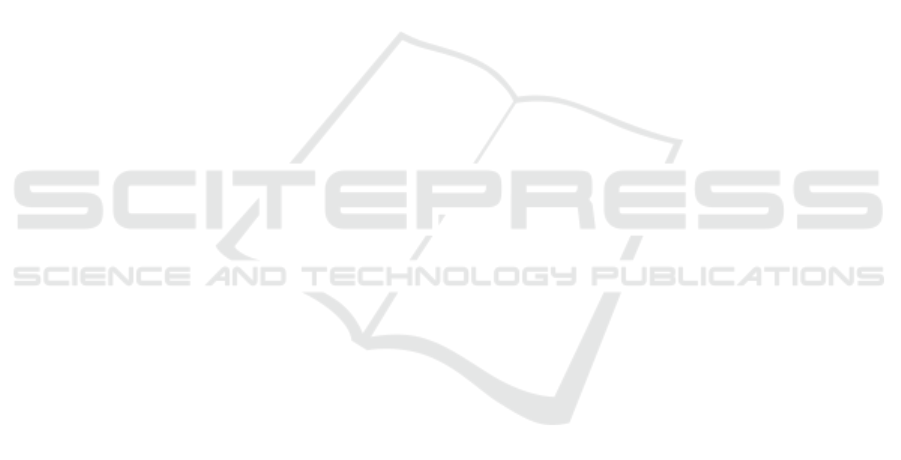
lowed by the reconstruction process. While simple
measures (like PSNR or SSIM) are sufficient here, it
is much more challenging to measure the similarity
between images that have been captured using differ-
ent sensors, at various spatial resolution. An example
of such images was shown earlier in Fig. 1b—I
(h)
and
I
(l)
i
were captured by different satellites, and certainly
the reconstructed image would be visually different
from I
(h)
. Importantly, the differences related to the
detail level may be negligible compared with those
incurred by the global variations. We have found the
keypoint detectors to be quite promising here (Kawu-
lok et al., 2017) and we will consider employing them
to evaluate the fitness. Overall, we currently explore
how to exploit the proposed framework to adapt an IM
alongside an SRR technique to images acquired by a
given sensor, which will be an important step towards
deploying SRR in real-life scenarios.
ACKNOWLEDGEMENTS
The reported work is a part of the SISPARE project
run by Future Processing and funded by European
Space Agency. In addition, the authors were partially
supported by Institute of Informatics funds no. BK-
230/RAu2/2017 (MK) and BKM-509/RAu2/2017
(DK).
REFERENCES
Akgun, T., Altunbasak, Y., and Mersereau, R. M. (2005).
Super-resolution reconstruction of hyperspectral im-
ages. IEEE Trans Image Process, 14(11):1860–1875.
Capel, D. and Zisserman, A. (2000). Super-resolution en-
hancement of text image sequences. In Proc. IEEE
ICPR, volume 1, pages 600–605.
Demirel, H. and Anbarjafari, G. (2011). Discrete wavelet
transform-based satellite image resolution enhance-
ment. IEEE Trans Geoscience and Remote Sensing,
49(6):1997–2004.
Dong, C., Loy, C. C., He, K., and Tang, X. (2016). Image
super-resolution using deep convolutional networks.
IEEE Trans Pattern Anal and Mach Intell, 38(2):295–
307.
Farsiu, S., Robinson, M. D., Elad, M., and Milanfar, P.
(2004). Fast and robust multiframe super resolution.
IEEE Trans Image Process, 13(10):1327–1344.
Hardie, R. (2007). A fast image super-resolution algorithm
using an adaptive wiener filter. IEEE Trans Image
Process, 16(12):2953–2964.
Jiang, J., Hu, R., Wang, Z., and Han, Z. (2014). Face super-
resolution via multilayer locality-constrained itera-
tive neighbor embedding and intermediate dictionary
learning. IEEE Trans Image Process, 23(10):4220–
4231.
Kawulok, M., Kostrzewa, D., Benecki, P., and Skonieczny,
L. (2017). Evaluating super-resolution reconstruction
of satellite images. In Proc. IAC 2017, pages 1–8. IAF.
Kim, J., Kwon Lee, J., and Mu Lee, K. (2016). Accurate
image super-resolution using very deep convolutional
networks. In Proc. IEEE CVPR, pages 1646–1654.
Li, F., Jia, X., and Fraser, D. (2008a). Universal HMT based
super resolution for remote sensing images. In Proc.
IEEE ICIP, pages 333–336.
Li, L., Zhang, Y., and Tian, Q. (2008b). Multi-face location
on embedded dsp image processing system. In Proc.
CISP, volume 4, pages 124–128.
Lukinavi
ˇ
cius, G., Umezawa, K., Olivier, N., et al.
(2013). A near-infrared fluorophore for live-cell
super-resolution microscopy of cellular proteins. Na-
ture Chemistry, 5(2):132–139.
Nasrollahi, K. and Moeslund, T. B. (2014). Super-
resolution: a comprehensive survey. Mach Vis and
App, 25(6):1423–1468.
Panagiotopoulou, A. and Anastassopoulos, V. (2012).
Super-resolution image reconstruction techniques:
Trade-offs between the data-fidelity and regularization
terms. Inform Fusion, 13(3):185–195.
Qian, S.-E. and Chen, G. (2012). Enhancing spatial resolu-
tion of hyperspectral imagery using sensor’s intrinsic
keystone distortion. IEEE Trans Geoscience and Re-
mote Sensing, 50(12):5033–5048.
Rudin, L. I., Osher, S., and Fatemi, E. (1992). Nonlinear
total variation based noise removal algorithms. Phys.
D, 60(1-4):259–268.
Timofte, R., De Smet, V., and Van Gool, L. (2014). A+:
Adjusted anchored neighborhood regression for fast
super-resolution. In Proc. ACCV, pages 111–126.
Springer.
Villena, S., Abad, J., Molina, R., and Katsaggelos, A. K.
(2004). Estimation of high resolution images and reg-
istration parameters from low resolution observations.
Proc. CIARP, pages 509–516.
Wang, Z., Bovik, A. C., Sheikh, H. R., and Simoncelli, E. P.
(2004). Image quality assessment: from error visibil-
ity to structural similarity. IEEE Trans Image Process,
pages 600–612.
Yang, F., Chen, Y., Wang, R., and Zhang, Q. (2015). Super-
resolution microwave imaging: Time-domain tomog-
raphy using highly accurate evolutionary optimization
method. In Proc. IEEE EuCAP, pages 1–4.
Yue, L., Shen, H., Li, J., Yuan, Q., Zhang, H., and Zhang, L.
(2016). Image super-resolution: The techniques, ap-
plications, and future. Signal Process, 128:389–408.
Zhu, H., Song, W., Tan, H., Wang, J., and Jia, D. (2016). Su-
per resolution reconstruction based on adaptive detail
enhancement for ZY-3 satellite images. Proc. ISPRS
Annals, pages 213–217.
Optimizing Super-resolution Reconstruction using a Genetic Algorithm
605