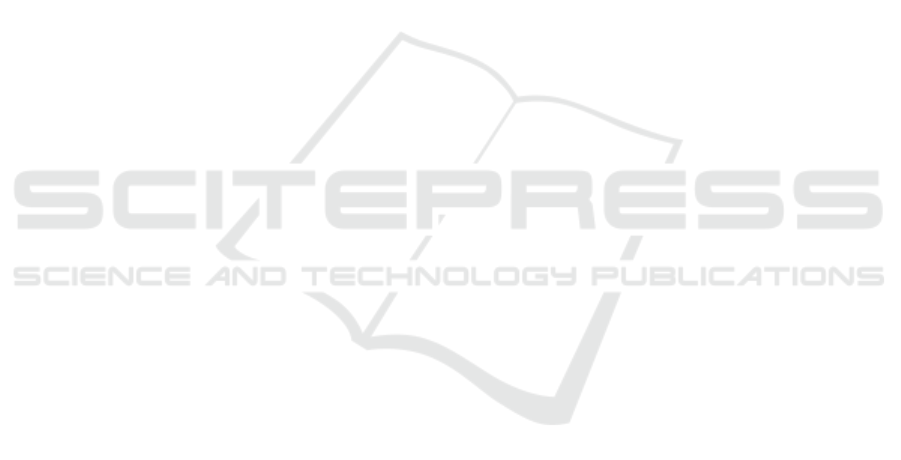
two stochastic diffusion parameters. Under the as-
sumption that the diffusion function is admissible and
that diffusion parameters are admissible for the dif-
fusion function, interesting asymptotic properties of
the multi-agent system were proved. In detail, the pa-
per showed proofs of the fact that diffusion does not
change the average opinion of the multi-agent system,
but that it influences its variance. In the last part of
the paper, a specific diffusion function is considered,
and expected properties of the average opinion and
of the variance of the opinion for the chosen diffu-
sion function were verified by independent simulati-
ons. Note that presented simulations do not depend
on the adopted kinetic approach. They are simple im-
plementations of the studied interaction rules.
The work reported in this paper can be extended
by considering multi-agent systems made of agents
with different propensity to change opinion because
of interactions. In (Bergenti and Monica, 2017), we
have already investigated the possibility of having dif-
ferent classes of agents in the same multi-agent sy-
stem, where different classes are associated with dif-
ferent parameters, such as different number of agents
and different values of the parameters of compromise.
Similar considerations are planned as future work for
the study of collective and asymptotic properties of
diffusion in multi-agent systems with multiple clas-
ses of agents. Finally, the model of diffusion stu-
died in this paper could be coupled with similar mo-
dels of other sociological phenomena, such as com-
promise and homophily, to study analytically the col-
lective and asymptotic properties of more complex sy-
stems. We have already studied the combined effects
of compromise and diffusion under specific assumpti-
ons in (Monica and Bergenti, 2015b), and we plan to
extend such results by modelling other phenomena.
REFERENCES
Bellouquid, A. and Delitala, M. (2006). Mathematical Mo-
deling of Complex Biological Systems. Modeling and
Simulation in Science, Engineering and Technology.
Birkh
¨
auser, Basel.
Bergenti, F. and Monica, S. (2016). Analytic study of opi-
nion dynamics in multi-agent systems with two clas-
ses of agents. In Santoro, C., Messina, F., and Be-
nedetti, M. D., editors, Proc. 17
th
Workshop “Dagli
Oggetti agli Agenti” (WOA 2016), volume 1664 of
CEUR Workshop Proceedings, pages 17–22, Catania,
Italy. RWTH Aachen University.
Bergenti, F. and Monica, S. (2017). An analytic study of
opinion dynamics in multi-agent systems. Compu-
ter and Mathematics with Applications, 73(10):2272–
2284.
Bonabeau, E. (2002). Agent-based modeling: Methods and
techniques for simulating human systems. Procee-
dings of the National Academy of Sciences, 99:7280–
7287.
Chakrabarti, B. K., Chakraborti, A., and Chatterjee, A.
(2006). Econophysics and sociophysics: Trends and
perspectives. Wiley, Berlin.
Cordier, S., Pareschi, L., and Toscani, G. (2005). On a ki-
netic model for simple market economy. Journal of
Statistical Physics, 120:253–277.
De Groot, M. H. (1974). Reaching a consensus. Journal
of the American Statistical Association, 69(345):118–
121.
Deffuant, G., Neau, D., Amblard, F., and Weisbuch, G.
(2000). Mixing beliefs among interacting agents. Ad-
vances in Complex Systems, 3:87–98.
D
¨
uring, B., Markowich, P., Pietschmann, J.-F., and Wolf-
ram, M.-T. (2009). Boltzmann and Fokker–Planck
equations modelling opinion formation in the pre-
sence of strong leaders. Proceedings of the Royal So-
ciety of London A: Mathematical, Physical and Engi-
neering Sciences, 465(2112):3687–3708.
Flache, A., M
¨
as, M., Feliciani, T., Chattoe-Brown, E., Def-
fuant, G., Huet, S., and Lorenz, J. (2017). Models of
social influence: Towards the next frontiers. Journal
of Artificial Societies and Social Simulation, 20(4).
Galam, S., Gefen, Y., and Shapir, Y. (1982). Sociophysics:
A new approach of sociological collective behaviour.
Journal of Mathematical Sociology, 9(1):1–13.
Hegselmann, R. and Krause, U. (2002). Opinion dynamics
and bounded confidence models, analysis, and simu-
lation. Journal of Artificial Societies and Social Simu-
lations, 5(3).
Mark, N. P. (2003). Culture and competition: Homophily
and distancing explainations for cultural niches. Ame-
rican Sociological Review, 68:319–345.
M
¨
as, M. and Flache, A. (2013). Differentiation without dis-
tancing: Explaining bi-polarization of opinions wit-
hout negative influence. PLOS One, 8(11).
M
¨
as, M., Flache, A., and Helbing, D. (2010). Individua-
lisazion as driving force of clustering phenomena in
humans. PLOS One, 6(10).
Monica, S. and Bergenti, F. (2014). A stochastic model
of self-stabilizing cellular automata for consensus for-
mation. In Santoro, C. and Bergenti, F., editors, Proc.
15
th
Workshop “Dagli Oggetti agli Agenti” (WOA
2014), volume 1260 of CEUR Workshop Proceedings,
Catania, Italy. RWTH Aachen University.
Monica, S. and Bergenti, F. (2015a). A kinetic study of opi-
nion dynamics in multi-agent systems. In Gavanelli,
M., Lamma, E., and Riguzzi, F., editors, AI*IA 2015
Advances in Artificial Intelligence, volume 9336 of
Lecture Notes in Computer Science, pages 116–127.
Springer.
Monica, S. and Bergenti, F. (2015b). Kinetic description
of opinion evolution in multi-agent systems: Analy-
tic model and simulations. In Chen, Q., Torroni, P.,
Villata, S., Hsu, J., and Omicini, A., editors, PRIMA
2015: Principles and Practice of Multi-Agent Sys-
tems, volume 9387 of Lecture Notes in Computer
Science, pages 483–491. Springer.
A Stochastic Model of Diffusion in Opinion Dynamics
123