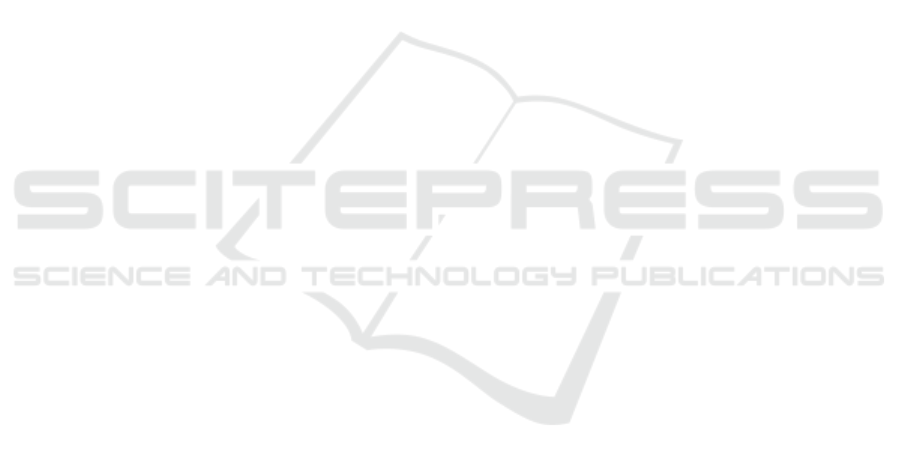
ios of data being described numerically and categor-
ically have been addressed. In future direction we
plan to analyze the efficiency of proposed approach
on benchmark dataset clustering to evaluate commu-
nication cost in reality. Moreover we plan to address
the problem when data is distributed among more than
two parties either horizontally or vertically.
REFERENCES
Artoisenet, C., Roland, M., and Closon, M. (2013). Health
networks: actors, professional relationships, and con-
troversies. In Collaborative Patient Centred eHealth,
volume 141. IOSPress.
Berkhin, P. (2006). A survey of clustering data mining tech-
niques. In Kogan, J., Nicholas, C., and Teboulle, M.,
editors, Grouping Multidimensional Data, pages 25–
71. Springer Berlin Heidelberg.
Bringer, J., Chabanne, H., Favre, M., Patey, A., Schneider,
T., and Zohner, M. (2014). Gshade: Faster privacy-
preserving distance computation and biometric identi-
fication. In Proceedings of the 2Nd ACM Workshop on
Information Hiding and Multimedia Security, pages
187–198, New York, NY, USA.
Bringer, J., Chabanne, H., and Patey, A. (2013). SHADE:
secure hamming distance computation from oblivious
transfer. In Financial Cryptography and Data Security
- FC 2013 Workshops, USEC and WAHC 2013, Oki-
nawa, Japan, April 1, 2013, Revised Selected Papers,
pages 164–176.
Bunn, P. and Ostrovsky, R. (2007). Secure two-party k-
means clustering. In Proceedings of the 14th ACM
Conference on Computer and Communications Secu-
rity, CCS ’07, pages 486–497, NY, USA. ACM.
Clifton, C., Kantarcioglu, M., Vaidya, J., Lin, X., and Zhu,
M. Y. (2002). Tools for privacy preserving distributed
data mining. SIGKDD Explor. Newsl., 4(2):28–34.
Day, W. H. E. and Edelsbrunner, H. (1984). Efficient algo-
rithms for agglomerative hierarchical clustering meth-
ods. Journal of Classification, 1(1):7–24.
De, I. and Tripathy, A. (2013). A secure two party hier-
archical clustering approach for vertically partitioned
data set with accuracy measure. In Recent Advances in
Intelligent Informatics - Proceedings of the Second In-
ternational Symposium on Intelligent Informatics, ISI
2013, August 23-24 2013, Mysore, India, pages 153–
162.
Faiella, M., Marra, A. L., Martinelli, F., Mercaldo, F., Sara-
cino, A., and Sheikhalishahi, M. (2017). A distributed
framework for collaborative and dynamic analysis of
android malware. In 25th Euromicro International
Conference on Parallel, Distributed and Network-
based Processing, PDP 2017, St. Petersburg, Russia,
March 6-8, 2017, pages 321–328.
Gan, G., Ma, C., and Wu, J. (2007). Data Clustering: The-
ory, Algorithms, and Applications (ASA-SIAM Series
on Statistics and Applied Probability). Society for In-
dustrial and Applied Mathematics, Philadelphia, PA,
USA.
Jagannathan, G. and Wright, R. N. (2005). Privacy-
preserving distributed k-means clustering over arbi-
trarily partitioned data. In Proceedings of the Eleventh
ACM SIGKDD International Conference on Knowl-
edge Discovery in Data Mining, KDD ’05, pages 593–
599, New York, NY, USA. ACM.
Jha, S., Kruger, L., and McDaniel, P. (2005). Privacy Pre-
serving Clustering, pages 397–417. Springer Berlin
Heidelberg, Berlin, Heidelberg.
Martinelli, F., Saracino, A., and Sheikhalishahi, M. (2016).
Modeling privacy aware information sharing systems:
A formal and general approach. In 15th IEEE Inter-
national Conference on Trust, Security and Privacy in
Computing and Communications.
Murtagh, F. and Contreras, P. (2012). Algorithms for hierar-
chical clustering: an overview. Wiley Interdisc. Rew.:
Data Mining and Knowledge Discovery, 2(1):86–97.
Oliveira, S. R. M. and Za
¨
ıane, O. R. (2002). Privacy pre-
serving frequent itemset mining. In Proceedings of
the IEEE International Conference on Privacy, Secu-
rity and Data Mining - Volume 14, CRPIT ’14, pages
43–54.
Sheikhalishahi, M. and Martinelli, F. (2017a). Privacy pre-
serving clustering over horizontal and vertical parti-
tioned data. In 2017 IEEE Symposium on Computers
and Communications, ISCC 2017, Heraklion, Greece,
July 3-6, 2017, pages 1237–1244.
Sheikhalishahi, M. and Martinelli, F. (2017b). Privacy-
utility feature selection as a privacy mechanism in
collaborative data classification. In The 26th IEEE
International Conference on Enabling Technologies:
Infrastructure for Collaborative Enterprises, Poznan,
Poland.
Su, C., Zhou, J., Bao, F., Takagi, T., and Sakurai, K.
(2007). Two-party privacy-preserving agglomerative
document clustering. In Proceedings of the 3rd Inter-
national Conference on Information Security Practice
and Experience, ISPEC’07, pages 193–208, Berlin,
Heidelberg. Springer-Verlag.
Vaidya, J. and Clifton, C. (2002). Privacy preserving associ-
ation rule mining in vertically partitioned data. In Pro-
ceedings of the Eighth ACM SIGKDD International
Conference on Knowledge Discovery and Data Min-
ing, KDD ’02, pages 639–644, New York, NY, USA.
ACM.
Secure Two-party Agglomerative Hierarchical Clustering Construction
437