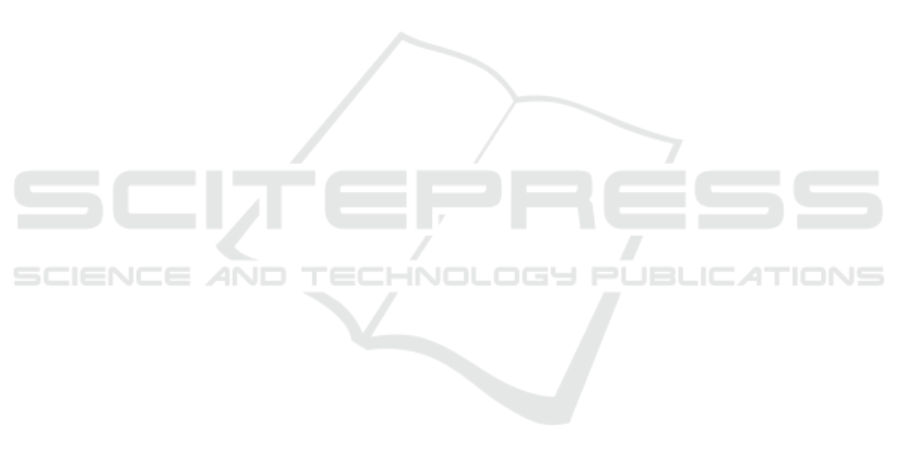
stages of the decision-making process. Defining and
simultaneously optimizing different combinations of
objectives can enrich the final set of the obtained al-
ternatives, which are eventually presented to the deci-
sion maker.
6 CONCLUSIONS
This study addressed the optimization of a WWTP
whose model parameters were estimated using real
Portuguese data. Two different approaches that can
be extended to any WWTP unit operation modelling
were considered. In the first approach, the WWTP de-
sign is addressed through a biobjective optimization
problem that consists of minimizing the total cost and
the quality index functions. The second approach is
more suitable for a draft project, when the exact loca-
tion and time where the WWTP is to be built is still
unknown. This approach involves the simultaneous
minimization of the influential variables, resulting in
a ten-objective optimization problem.
The results obtained in this study clearly show that
the WWTP design can be effectively approached by
simultaneously optimizing multiple objectives natu-
rally associated with the problem. The achieved opti-
mal solutions are meaningful in physical terms and
are economically attractive. Investment and opera-
tion costs are highly influenced by the optimized vari-
ables, stressing the importance of optimization. Both
approaches to WWTP design provide a set of non-
dominated solutions that can be used to gain valuable
insights about the problem and possible decision mak-
ing alternatives. This information can help to elabo-
rate a first version of the project and refine important
design choices when the specific location and moment
in time are defined.
ACKNOWLEDGEMENTS
This work has been supported by the Portuguese
Foundation for Science and Technology (FCT) in the
scope of the project UID/CEC/00319/2013 (ALGO-
RITMI R&D Center).
REFERENCES
Alex, J., Benedetti, L., Copp, J., Gernaey, K. V., Jepps-
son, U., Nopens, I., Pons, M. N., Rosen, C., Steyer,
J. P., and Vanrolleghem, P. (2008). Benchmark sim-
ulation model no. 1 (BSM1). Technical report, IWA
Taskgroup on Benchmarking of Control Strategies for
WWTPs.
Deb, K., Pratap, A., Agarwal, S., and Meyarivan, T. (2002).
A fast and elitist multiobjective genetic algorithm:
NSGA-II. IEEE Trans. Evol. Comput., 6(2):182–197.
Denysiuk, R., Costa, L., and Esp
´
ırito Santo, I. (2013).
Many-objective optimization using differential evolu-
tion with variable-wise mutation restriction. In Proc.
Genet. Evol. Comput. Conf., pages 591–598.
Denysiuk, R., Costa, L., and Esp
´
ırito Santo, I. (2014).
Clustering-based selection for evolutionary many-
objective optimization. In Bartz-Beielstein, T.,
Branke, J., Filipi
ˇ
c, B., and Smith, J., editors, PPSN
XIII, volume 8672 of LNCS, pages 538–547.
Denysiuk, R. and Gaspar-Cunha, A. (2017a). Multiob-
jective evolutionary algorithm based on vector angle
neighborhood. Swarm Evol. Comput., 37:45–57.
Denysiuk, R. and Gaspar-Cunha, A. (2017b). Weighted
stress function method for multiobjective evolutionary
algorithm based on decomposition. In Trautmann, H.,
Rudolph, G., Klamroth, K., Sch
¨
utze, O., Wiecek, M.,
Jin, Y., and Grimme, C., editors, EMO, volume 10173
of LNCS, pages 176–190.
Esp
´
ırito Santo, I. A. C. P., Costa, L., Denysiuk, R., and Fer-
nandes, E. M. G. P. (2010). Hybrid genetic pattern
search augmented Lagrangian algorithm: Application
to WWTP optimization. In Proc. Conf. on Applied
Oper. Res., pages 45–56.
Esp
´
ırito Santo, I. A. C. P., Fernandes, E. M. G. P., Ara
´
ujo,
M. M., and Ferreira, E. C. (2006). On the secondary
settler models robustness by simulation. WSEAS
Transactions on Information Science and Applica-
tions, 3:2323–2330.
Henze, M., Jr, C. P. L. G., Gujer, W., Marais, G. V. R.,
and Matsuo, T. (1986). Activated sludge model no. 1.
Technical report, IAWPRC Task Group on Mathemat-
ical Modelling for design and operation of biological
wastewater treatment.
Miettinen, K. (1999). Nonlinear multiobjective optimiza-
tion, volume 12 of International Series in Operations
Research and Management Science. Kluwer Aca-
demic Publishers.
Tyteca, D. (1976). Cost functions for wastewater con-
veyance systems. J. Water Pollut. Control Fed.,
48(9):2120–2130.
Zeng, G.-M., Zhang, S.-F., Qin, X.-S., Huang, G.-H., and
Li, J.-B. (2003). Application of numerical simulation
on optimum design of two-dimensional sedimentation
tanks in the wastewater treatment plant. J. Environ.
Sci., 15(3):346–350.
Zhang, Q. and Li, H. (2007). MOEA/D: A multiobjective
evolutionary algorithm based on decomposition. IEEE
Trans. Evol. Comput., 11(6):712–731.
Zitzler, E. and K
¨
unzli, S. (2004). Indicator-based selection
in multiobjective search. In Yao, E. K., Burke, J. A.,
Lozano, J., Smith, J. J., Merelo-Guerv
´
os, J. A., Bul-
linaria, J. E., Rowe, P., Ti
ˇ
no, Kab
´
an, A., and Schwe-
fel, H.-P., editors, PPSN VIII, volume 3242 of LNCS,
pages 832–842.
ICORES 2018 - 7th International Conference on Operations Research and Enterprise Systems
334