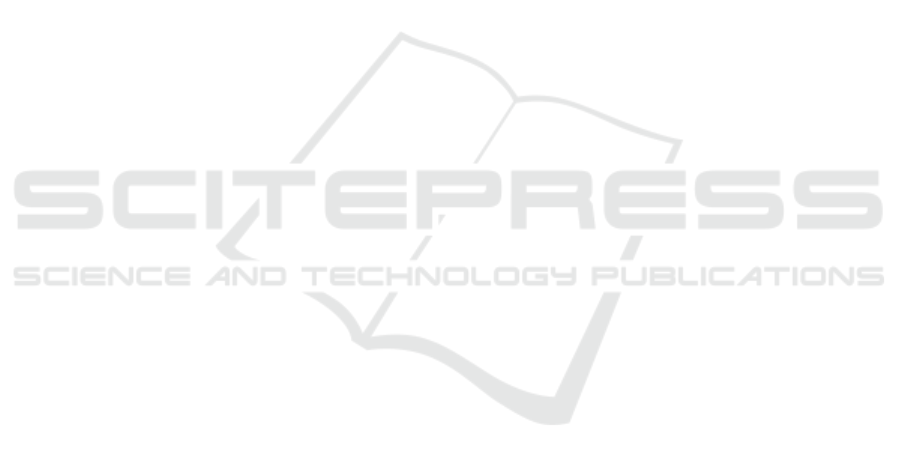
Conference, DASFAA 2013, International Workshops:
BDMA, SNSM, SeCoP, Wuhan, China, April 22-25,
2013. Proceedings, volume 7827 of Lecture Notes in
Computer Science, pages 1–15. Springer.
Crone, S. F. and Finlay, S. (2012). Instance sampling in
credit scoring: An empirical study of sample size
and balancing. International Journal of Forecasting,
28(1):224–238.
Donmez, P., Carbonell, J. G., and Bennett, P. N. (2007).
Dual strategy active learning. In ECML, volume 4701
of Lecture Notes in Computer Science, pages 116–
127. Springer.
Edge, M. E. and Sampaio, P. R. F. (2009). A survey of
signature based methods for financial fraud detection.
Computers & Security, 28(6):381–394.
Faraggi, D. and Reiser, B. (2002). Estimation of the area un-
der the roc curve. Statistics in medicine, 21(20):3093–
3106.
Gao, J., Fan, W., Han, J., and Yu, P. S. (2007). A general
framework for mining concept-drifting data streams
with skewed distributions. In Proceedings of the Sev-
enth SIAM International Conference on Data Min-
ing, April 26-28, 2007, Minneapolis, Minnesota, USA,
pages 3–14. SIAM.
Goldstein, M. and Uchida, S. (2016). A comparative eval-
uation of unsupervised anomaly detection algorithms
for multivariate data. PloS one, 11(4):e0152173.
Gopinathan, K. M., Biafore, L. S., Ferguson, W. M.,
Lazarus, M. A., Pathria, A. K., and Jost, A. (1998).
Fraud detection using predictive modeling. US Patent
5,819,226.
He, H. and Garcia, E. A. (2009). Learning from imbalanced
data. IEEE Trans. Knowl. Data Eng., 21(9):1263–
1284.
Hoffman, A. J. and Tessendorf, R. E. (2005). Artificial in-
telligence based fraud agent to identify supply chain
irregularities. In Hamza, M. H., editor, IASTED In-
ternational Conference on Artificial Intelligence and
Applications, part of the 23rd Multi-Conference on
Applied Informatics, Innsbruck, Austria, February 14-
16, 2005, pages 743–750. IASTED/ACTA Press.
Hooi, B., Shah, N., Beutel, A., G
¨
unnemann, S., Akoglu,
L., Kumar, M., Makhija, D., and Faloutsos, C. (2016).
BIRDNEST: bayesian inference for ratings-fraud de-
tection. In Venkatasubramanian, S. C. and Jr., W. M.,
editors, Proceedings of the 2016 SIAM International
Conference on Data Mining, Miami, Florida, USA,
May 5-7, 2016, pages 495–503. SIAM.
Japkowicz, N. and Stephen, S. (2002). The class imbal-
ance problem: A systematic study. Intell. Data Anal.,
6(5):429–449.
Lek, M., Anandarajah, B., Cerpa, N., and Jamieson, R.
(2001). Data mining prototype for detecting e-
commerce fraud. In Smithson, S., Gricar, J., Pod-
logar, M., and Avgerinou, S., editors, Proceedings of
the 9th European Conference on Information Systems,
Global Co-operation in the New Millennium, ECIS
2001, Bled, Slovenia, June 27-29, 2001, pages 160–
165.
Lenard, M. J. and Alam, P. (2005). Application of fuzzy
logic fraud detection. In Khosrow-Pour, M., editor,
Encyclopedia of Information Science and Technology
(5 Volumes), pages 135–139. Idea Group.
Marqu
´
es, A. I., Garc
´
ıa, V., and S
´
anchez, J. S. (2013). On the
suitability of resampling techniques for the class im-
balance problem in credit scoring. JORS, 64(7):1060–
1070.
Phua, C., Lee, V. C. S., Smith-Miles, K., and Gayler, R. W.
(2010). A comprehensive survey of data mining-based
fraud detection research. CoRR, abs/1009.6119.
Powers, D. M. (2011). Evaluation: from precision, recall
and f-measure to roc, informedness, markedness and
correlation.
Pozzolo, A. D., Caelen, O., Borgne, Y. L., Waterschoot, S.,
and Bontempi, G. (2014). Learned lessons in credit
card fraud detection from a practitioner perspective.
Expert Syst. Appl., 41(10):4915–4928.
Pozzolo, A. D., Caelen, O., Johnson, R. A., and Bontempi,
G. (2015). Calibrating probability with undersampling
for unbalanced classification. In IEEE Symposium Se-
ries on Computational Intelligence, SSCI 2015, Cape
Town, South Africa, December 7-10, 2015, pages
159–166. IEEE.
Sahin, Y., Bulkan, S., and Duman, E. (2013). A cost-
sensitive decision tree approach for fraud detection.
Expert Syst. Appl., 40(15):5916–5923.
Saia, R. (2017). A discrete wavelet transform approach to
fraud detection. In Yan, Z., Molva, R., Mazurczyk,
W., and Kantola, R., editors, Network and System
Security - 11th International Conference, NSS 2017,
Helsinki, Finland, August 21-23, 2017, Proceedings,
volume 10394 of Lecture Notes in Computer Science,
pages 464–474. Springer.
Saia, R., Boratto, L., and Carta, S. (2015). Multiple be-
havioral models: A divide and conquer strategy to
fraud detection in financial data streams. In Fred, A.
L. N., Dietz, J. L. G., Aveiro, D., Liu, K., and Fil-
ipe, J., editors, KDIR 2015 - Proceedings of the Inter-
national Conference on Knowledge Discovery and In-
formation Retrieval, part of the 7th International Joint
Conference on Knowledge Discovery, Knowledge En-
gineering and Knowledge Management (IC3K 2015),
Volume 1, Lisbon, Portugal, November 12-14, 2015,
pages 496–503. SciTePress.
Saia, R. and Carta, S. (2017a). Evaluating credit card trans-
actions in the frequency domain for a proactive fraud
detection approach. In Samarati, P., Obaidat, M. S.,
and Cabello, E., editors, Proceedings of the 14th Inter-
national Joint Conference on e-Business and Telecom-
munications (ICETE 2017) - Volume 4: SECRYPT,
Madrid, Spain, July 24-26, 2017., pages 335–342.
SciTePress.
Saia, R. and Carta, S. (2017b). A frequency-domain-based
pattern mining for credit card fraud detection. In Ra-
machandran, M., Mu
˜
noz, V. M., Kantere, V., Wills,
G., Walters, R. J., and Chang, V., editors, Proceed-
ings of the 2nd International Conference on Inter-
net of Things, Big Data and Security, IoTBDS 2017,
Porto, Portugal, April 24-26, 2017, pages 386–391.
SciTePress.
Unbalanced Data Classification in Fraud Detection by Introducing a Multidimensional Space Analysis
39