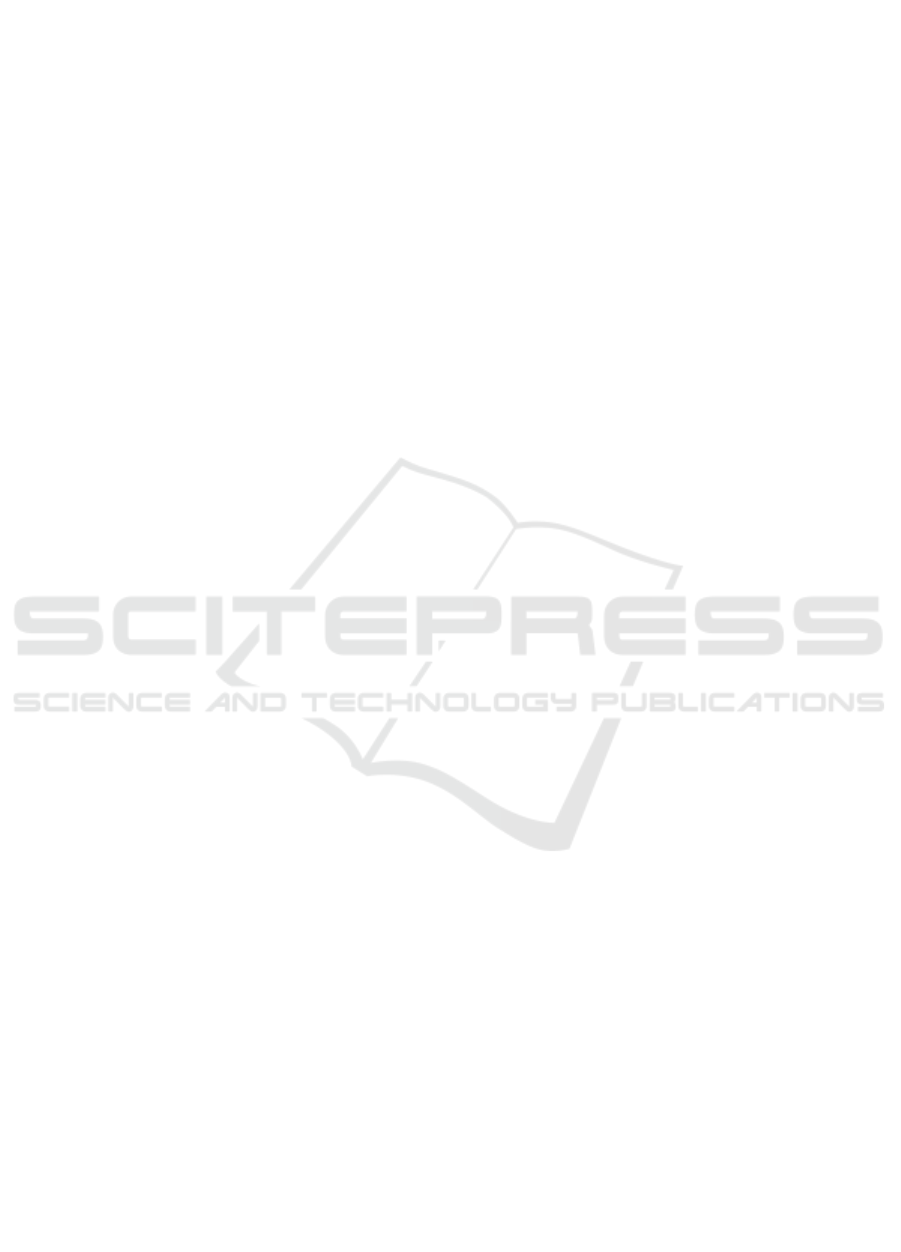
5 CONCLUSIONS
Aiming at the problem to utilize the spectral sparse
property in the hyperspectral CS remote sensing
imaging, this paper presents a new sampling and
reconstruction method based on the spectral sparse
representation. By learning the spectral sparse
dictionary to constrain the spectral region in the
reconstruction and optimizing the spatial precision
via total variation constraint, the AVIRIS
hyperspectral scene is reconstructed in very high
quality from 25% compressive measurements, which
provides a new idea to enhance the hyperspectral
sampling efficiency. Compared with other presented
hyperspectral CS reconstruction algorithms, the
reconstruction precision in spatial and spectral region
of our method has a significant superiority in the same
experimental condition.
However, there still exist some problems to be
further studied in order to better apply the new theory.
One is the method of the spectral training sample
construction and dictionary learning. In the
experiment it is found that if the training samples are
extracted from the sensor or the type of ground object
with a great difference from that of the reconstructed
area, the effect of the spectral sparse representation is
significantly affected and the reconstruction precision
decreases. The other one is the realization of spectral
random coding in hardware, for the spectral sampling
scheme is more difficult to realize than spatial
sampling.
ACKNOWLEDGEMENTS
This work is supported by the National Key Research
and Development Program of China under Grant
2016YFB0500402, CAS/SAFEA International
Partnership Program for Creative Research Teams
under Grant 2013AA1229 and the Strategic Priority
Research Program of the Chinese Academy of
Sciences under Grant XDA13030402.
REFERENCES
Donoho, David L, 2006. Compressed sensing. IEEE Trans.
Inform. Theory. 52.4, p.1289-1306.
Li, Sheng Liang, et al. 2014, Innovative remote sensing
imaging method based on compressed sensing. Optics
& Laser Technology. 63.4, p.83–89.
Ashwin, Wagadarikar, et al. 2008, Single disperser design
for coded aperture snapshot spectral imaging. Applied
Optics. 47.10, p.44-51.
Russell, Thomas A., et al. 2012, Compressive hyperspectral
sensor for LWIR gas detection. Proceedings of SPIE -
The International Society for Optical Engineering.
8365, p. 83650C-83650C-13.
Wu Jianrong, Shen Xia, Yu Hong, et al. 2014, Snapshot
compressive imaging by phase modulation. Acta Optica
Sinica. 10, p.113-120.
Combettes, Patrick L, and P. Jean-Christophe. 2004, Image
restoration subject to a total variation constraint. IEEE
Transactions on Image Processing a Publication of the
IEEE Signal Processing Society. 13.9, p.1213-22.
Zhang L, Zhang Y, Wei W, 2014, 3D total variation
hyperspectral compressive sensing using unmixing.
Geoscience and Remote Sensing Symposium (IGARSS)
2014, p.2961-2964.
Feng Yan, JiaYingbiao, Cao Yuming, et al. 2012,
Compressed sensing projection and compound
regularizer reconstruction for hyperspectral images.
Acta Aeronautica et Astronautica Sinica, 33(8), p.1466-
1473.
Liu Haiying, Wu Chengke, Lv Pei, et al. 2011, Compressed
hyperspectral image sensing reconstruction based
oninterband prediction and joint optimization. Journal
of Electronics & Information Technology, 33(9), p.
2248-2252.
Golbabaee, Mohammad, and P. Vandergheynst. 2012,
Compressed sensing of simultaneous low-rank and
joint-sparse matrices. IEEE Transactions on
Information Theory .
JiaYingbiao, Feng Yan, Wang Zhongliang, et al. 2014,
Hyperspectral compressive sensing recovery via
spectrum structure similarity. Journal of Electronics
and Information Technology, (6), p.1406-1412.
Zare, A., P. Gader, and K. S. Gurumoorthy. 2012, Directly
measuring material proportions using hyperspectral
compressive sensing. Geoscience & Remote Sensing
Letters IEEE. 9.3, p.323-327.
Charles, A. S., Olshausen, B.A., and C. J. Rozell. 2011,
Learning sparse codes for hyperspectral imagery.
Selected Topics in Signal Processing IEEE Journal
of. 5.5, p.963-978.
Wang Qi, Li Chuanrong, Ma Lingling, et al. 2013,
Compressive sensing spectral sparsification method
based on training dictionary. Remote Sensing
Technology and Application, 28.6, p.59-60.
Aharon, M., M. Elad, and A. Bruckstein. K-SVD: An
algorithm for designing overcomplete dictionaries for
sparse representation. IEEE Transactions on Signal
Processing, 54.11(2006):4311-4322.
Mairal, Julien, et al. 2009, Online dictionary learning for
sparse coding. Proceedings of the 26th Annual
International Conference on Machine Learning ACM,
p. 689-696.
Skretting, Karl, and K. Engan. 2010, Recursive Least
Squares Dictionary Learning Algorithm. IEEE
Transactions on Signal Processing. 58.4, p.2121 - 2130.
Azimi-Sadjadi M R, Kopacz J, Klausner N. 2015, K-SVD
dictionary learning using a fast OMP with applications.
IEEE International Conference on Image Processing. p.
1599-1603.
PHOTOPTICS 2018 - 6th International Conference on Photonics, Optics and Laser Technology
278