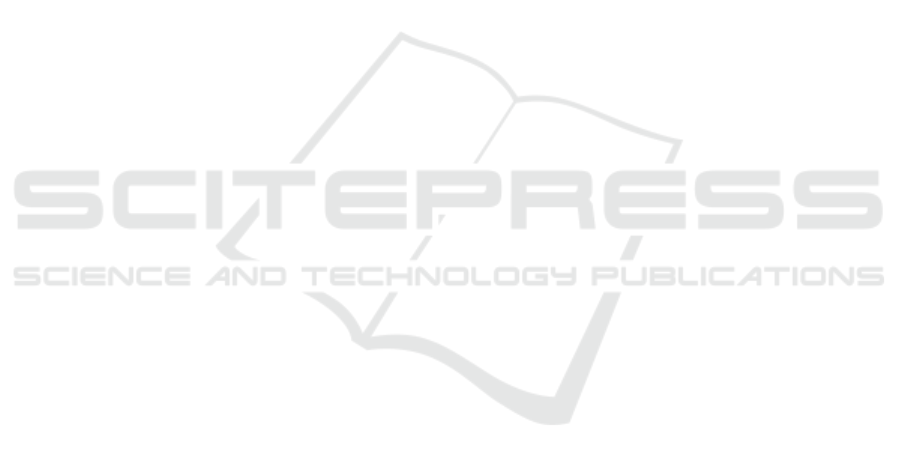
trol to anticipate and use electrical power ranges that
are recoverable due to a future regenerative braking.
The adaptive method, as well as any non predictive
method, would not have the necessary information to
anticipate. Thus, the obtained solution could some-
times be wrongly careful when a recoverable amount
of electrical energy is available.
The predictive strategy yields better fuel con-
sumption and CO
2
emissions on 5 different cycles, in
comparison to the CostBased adaptive method. How-
ever, the method relies on the prediction to be close
enough to the real power demand in order to be able
to approach the targeted state of charge.
Future works will improve the efficiency of the
proposed predictive solution with less simplifications
in the optimization modelling. A more representative
model of the 12V net can be considered. The low volt-
age net model could be introduced in the optimal con-
trol modelling and the state of charge of the 12V bat-
tery can be controlled to reach a targeted final value.
Dynamics such as temperature, and mechanical trans-
mission losses can also be introduced to enhance the
optimal control problem, and make the model closer
to a real vehicle.
ACKNOWLEDGEMENTS
This work was supported by the French Environ-
ment and Energy Management Agency (ADEME)
and Continental Automotive. We also thank our col-
leagues from Continental Automotive who provided
insight and expertise that greatly assisted the research.
REFERENCES
Caux, S., Wanderley-Honda, D., Hissel, D., and Fadel, M.
(2010). On-line energy management for hev based on
particle swarm optimization. In IEEE Vehicle Power
and Propulsion (VPP) Conference, Lille, France.
Delprat, S., Guerra, T., and Rimaux, J. (2003). Control
strategies for hybrid vehicles: Synthesis and evalua-
tion. In Vehicular Technology Conference, 2003. VTC
2003-Fall. 2003 IEEE 58th, Orlando, FL, USA.
He, H., Zhang, J., and Li, G. (2015). Model predictive con-
trol for energy management of a plug-in hybrid elec-
tric bus. In CUE2015-Applied Energy Symposium and
Summit 2015: Low carbon cities and urban energy
systems, Fuzhou, China.
Kermani, S., Trigui, R., Delprat, S., Jeanneret, B., and
Guerra, T. M. (march 2011). Phil implementation of
energy management optimization for a parallel hev on
a predefined trip. In IEEE Transactions on vehicular
technology, Vol. 60, No. 3.
Kim, N., Cha, S., and Peng, H. (september 2011). Optimal
control of hybrid electric vehicles based on pontryagin
minimum principles. In IEEE Transactions on control
systems technology, Vol. 19, No. 5.
Sciarretta, A. and Guzzella, L. (april 2007). Control of
hybrid electric vehicles. optimal energy management
strategies. In IEEE Control Systems Magazine.
Sciarretta, A., Guzzella, L., and Back, M. (2004). A real-
time optimal control strategy for parallel hybrid vehi-
cles with on-board estimation of control parameters.
In in Proc. IFAC Symp. Advances Automotive Control,
Paper 136, pp. 502507, Salerno, Italy.
Steinmauer, G. and del Re, L. (2001). Optimal control of
dual power sources. In Proc. 2001 IEEE Int. Conf.
Control Applications, pp. 422-442, Mexico City, Mex-
ico.
Tribioli, L. and Onori, S. (June 17-19, 2013). Analysis of
energy management strategies in plug-in hybrid elec-
tric vehicles: application to the gm chevrolet volt. In
2013 American Control Conference (ACC), Washing-
ton, DC, USA.
VEHITS 2018 - 4th International Conference on Vehicle Technology and Intelligent Transport Systems
268