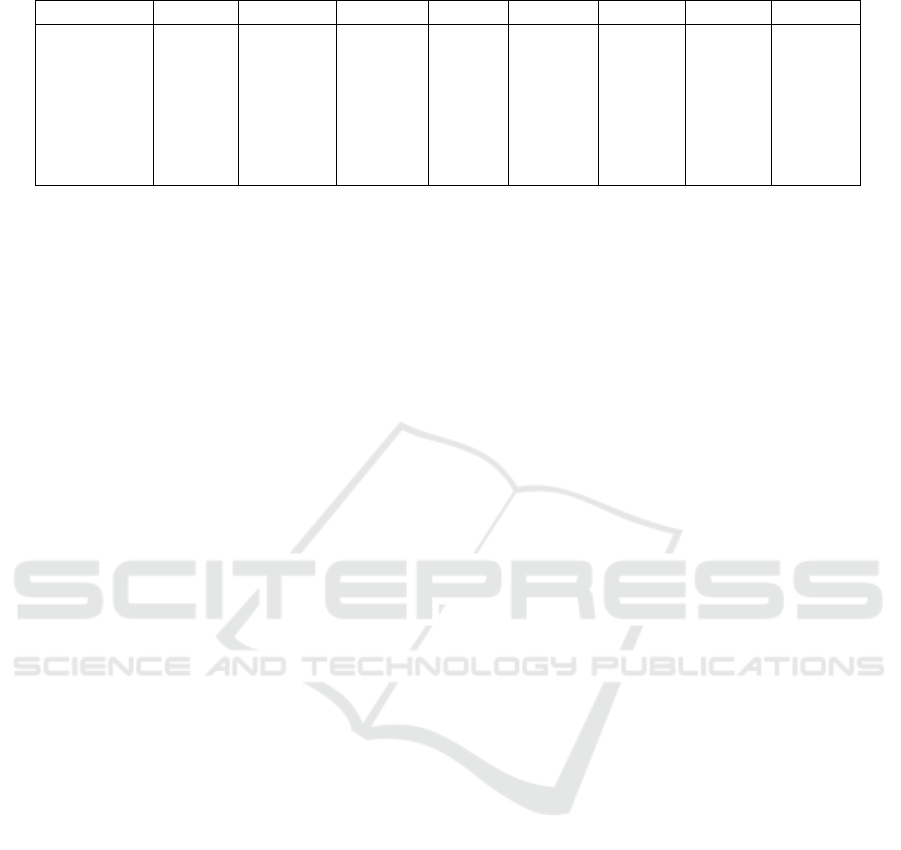
Table 7: State predictions results for Barbato et.al. approach (Barbato et al., 2011) on ECO2.
Device Correct Corr. Off Corr. on Wrong Dev. off Dev. on On cov. Off cov.
Dishwasher 0.9854 0.9854 0 0.0147 0.9854 0.0147 0 1
Air exhaust 0.962 0.9923 0.0155 0.0381 0.992 0.0081 0.0598 0.9693
Fridge 0.5004 0.6292 0.378 0.4997 0.6256 0.3745 0.5175 0.4902
Freezer 0.5146 0.4954 0.5281 0.4855 0.4817 0.5184 0.597 0.4259
Lamp 0.8987 0.9213 0.4768 0.1014 0.901 0.0991 0.2446 0.9706
TV 0.7156 0.835 0.4159 0.2845 0.7635 0.2366 0.501 0.7821
Stereo 0.6213 0.751 0.448 0.3788 0.6659 0.3342 0.5737 0.6452
age predictions of devices for which the sensor havew
failed. This is accurate for devices that have strong
sequential relationships amongst each other, that is,
these devices are often used together, or one after the
other. Cyclic and peak patterns, on the other hand, are
harder to predict with the proposed approach. This is
especially true when there is not a large quantity of
data available, therefore cyclic and peak patterns will
require a different set of techniques.
ACKNOWLEDGEMENT
Mathieu Kalksma thanks the Distributed Systems
Group at the University of Groningen for the opportu-
nity of and support while performing the presented re-
search. The work is partially supported by the Dutch
National Research Council Beijing Groningen Smart
Energy Cities Project, contract no. 467-14-037.
REFERENCES
Agrawal, R., Imielinski, T., and Swami, A. (1993). Min-
ing associations between sets of items in massive
databases. In Proceedings of the ACM-SIGMOD Int’l
Conference on Management of Data, pages 207–216.
Barbato, A., Capone, A., Rodolfi, M., and Tagliaferri, D.
(2011). Forecasting the usage of household appliances
through power meter sensors for demand management
in the smart grid. In 2011 IEEE International Con-
ference on Smart Grid Communications (SmartGrid-
Comm), pages 404–409.
Basu, K., Hawarah, L., Arghira, N., Joumaa, H., and Ploix,
S. (2013). A prediction system for home appliance
usage. Energy and Buildings, 67:668–679.
Beckel, C., Kleiminger, W., Cicchetti, R., Staake, T., and
Santini, S. (2014). The eco data set and the perfor-
mance of non-intrusive load monitoring algorithms. In
Proceedings of the 1st ACM International Conference
on Embedded Systems for Energy-Efficient Buildings
(BuildSys 2014). Memphis, TN, USA, pages 80–89.
ACM.
Cicchetti, R. (2014). Nilm-eval: Disaggregation of real-
world electricity consumption data. Master’s thesis,
Swiss Federal Institute of Technology Zurich.
De Almeida, A., Fonseca, P., Schlomann, B., Feilberg, N.,
and Ferreira, C. (2006). Residential monitoring to
decrease energy use and carbon emissions in europe.
In International Energy Efficiency in Domestic Appli-
ances & Lighting Conference.
Deshpande, M. and Karypis, G. (2004). Selective markov
models for predicting web page accesses. ACM Trans.
Internet Technol., 4(2):163–184.
EIA (2016). How is electricity used in u.s. homes?
https://www.eia.gov/tools/faqs/faq.php?id=96&t=3.
Accessed: 29-12-2017.
Georgievski, I., Degeler, V., Pagani, G. A., Nguyen, T. A.,
Lazovik, A., and Aiello, M. (2012). Optimizing en-
ergy costs for offices connected to the smart grid.
IEEE Trans. Smart Grid, 3(4):2273–2285.
Jung, H. C., Kim, J. S., and Heo, H. (2015). Prediction
of building energy consumption using an improved
real coded genetic algorithm based least squares sup-
port vector machine approach. Energy and Buildings,
90:76–84.
Kalksma, M. (2016). Mining household appliances patterns
by monitoring electric plug loads. Master’s thesis,
University of Groningen.
Li, K., Hu, C., Liu, G., and Xue, W. (2015). Building’s elec-
tricity consumption prediction using optimized artifi-
cial neural networks and principal component analy-
sis. Energy and Buildings, 108:106–113.
Pitkow, J. and Pirolli, P. (1999). Mining longest repeating
subsequences to predict world wide web surfing. Pro-
ceedings of USITS ’99: The 2nd USENIX Symposium
on Internet Technologies \& Systems, pages 139–150.
Pratama, A. R., Widyawan, Lazovik, A., and Aiello, M. (In
Press). Power-based device recognition for occupancy
detection. In Service-Oriented Computing - ICSOC
2017 Workshop.
Tang, G., Wu, K., Lei, J., and Tang, J. (2014). Plug and
play! A simple, universal model for energy disaggre-
gation. CoRR, abs/1404.1884.
Wang, Z. and Ding, Y. (2015). An occupant-based energy
consumption prediction model for office equipment.
Energy and Buildings, 109:12–22.
Zhang, F., Deb, C., Lee, S. E., Yang, J., and Shah, K. W.
(2016). Time series forecasting for building en-
ergy consumption using weighted Support Vector Re-
gression with differential evolution optimization tech-
nique. Energy and Buildings, 126:94–103.
Mining Sequential Patterns for Appliance Usage Prediction
33