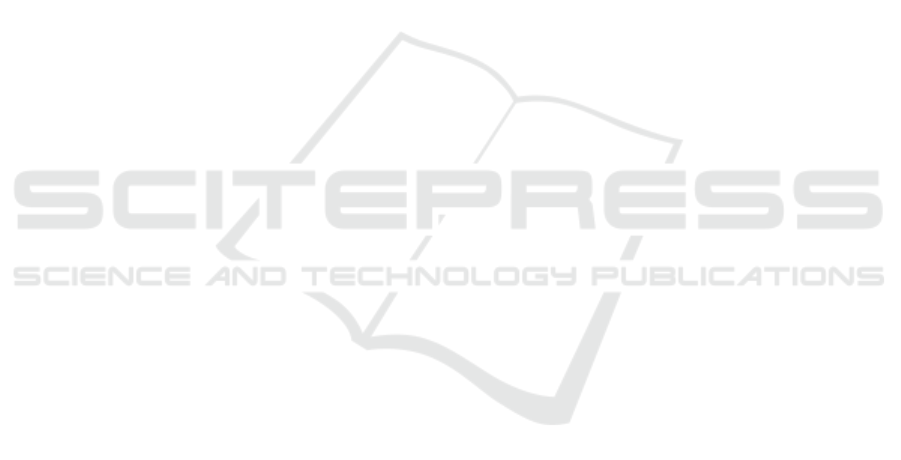
sor regeneration stage for acquiring the target mea-
surement information from sensor system with multi-
point sampling.
The analysis of measurement data was focused on
identifying the feature of sensor signal, which is lin-
early related to the number of people in the concert
hall. In the study, the following issues were taken into
consideration:
• sampling points, numbered with k ∈
{
1, 2, 3, 4
}
.
• advancement of the exposure phase for k
th
sam-
pling point (time which elapsed since it started),
i ∈
{
1, 2, . . . ..., 30
}
s;
• advancement of the concert (time which elapsed
since it started; We limited the period to 1 h), j ∈
{
1, 2, . . . ..., 60
}
min;
There were considered two kinds of features, raw
sensor response, R and corrected sensor response, R−
R
0
. Once properly indexed, they have the following
meaning:
• R
i, j,k
is the value of sensor signal recorded at time
i of sensor exposure to gas sample delivered from
sampling point k, while the exposure started a
time j since the beginning of the concert;
• R
0
j,k
is the last value of sensor signal recorded
during sensor exposure to purified air, prior to
switching to sampling point k, while the switch-
ing takes place at time j since the beginning of
the concert.
For each sampling point, k there were considered
the following relationships:
N
=
a
1
+
a
2
R
i
,
j
,
k
+
ε
(1)
N = b
1
+ b
2
(
R
i, j,k
− R
0
j,k
)
+ ε (2)
where N stands for the number of people in the con-
cert hall. The data utilized for regression fitting was
collected during 10 concerts, as listed in Table ??.
The performance of fitting was examined using co-
efficient of determination (R
2
) and root mean squared
error (RMSE).
4 RESULTS
In Figure ?? there is shown the raw signal of
TGS2600 sensor, recorded during a single measure-
ment cycle.
As indicated by the orange line in Figure ??, the sen-
sor system was first exposed to purified air (regen-
eration), followed by exposure to gas delivered from
sampling points 1, 2, 3 and 4 in turn, interchangeably
with exposure to purified air. As shown in Figure ??,
the exposure to gas from any sampling point caused
increase of sensor signal and the exposure to purified
air resulted in signal decrease. Changes of sensor sig-
nal at individual measurement points were different.
Also, the degree of sensor regeneration achieved after
the subsequent exposures was not the same. This fact
was responsible for the overall shift of sensor signal
in time.
The shift may be observed in Figure ??. It shows
the behavior of the raw sensor signal recorded dur-
ing an exemplary day. Four subplots display measure-
ment data which refer to individual sampling points.
More precisely, the data plotted in a single subplot
comes from 30 s of exposure to the air delivered from
a measurement point, once every 4 minutes. The re-
maining 3 min 30 s of measurement cycle are void of
data for the particular location. As shown, for each
considered location, the raw sensor signal varied in
time. Considerable part of the variation was caused
by the properties of sampled air. The hall was not oc-
cupied during the night. However, around 9:00 a.m.
there started numerous activities, namely rehearsals
and later, preparations immediately preceding con-
certs. In the afternoon, at 15:00 and in the evening,
at 19:00 there were concerts. All these events were
associated with considerable variations of room oc-
cupancy in terms of the number of people, their ac-
tivities and distribution in the hall. This was reflected
in the variation of sensor’s responses. However, some
part of the senor signal change has to be attributed to
the memory effect. If there was no memory effect,
the sensor signal would have approximately the same
value at the beginning of each subsequent exposure.
The incomplete regeneration results in baseline shift.
In Figure ??, there is shown the time series of raw
responses, R recorded during exposure of TGS2600
sensor to the gas delivered sequentially from four
sampling points, in the period of one day. However,
there was displayed just the data recorded during a
single second of each exposure. The middle, 15
th
sec-
ond of exposure was chosen. Figure ?? was prepared
as a reference to Figure ??.
In Figure ?? there is shown the time series of cor-
rected responses of sensor TGS2600, recorded dur-
ing 15
th
second of exposure. The correction R − R
0
consisted in subtracting the last response of sensor
recorded upon regeneration, prior to exposure, from
the raw sensor response recorded in 15
th
s of expo-
sure. The value of R
0
was not constant for all expo-
Sensor System with Multi-point Sampling Applied to IAQ Measurements
151