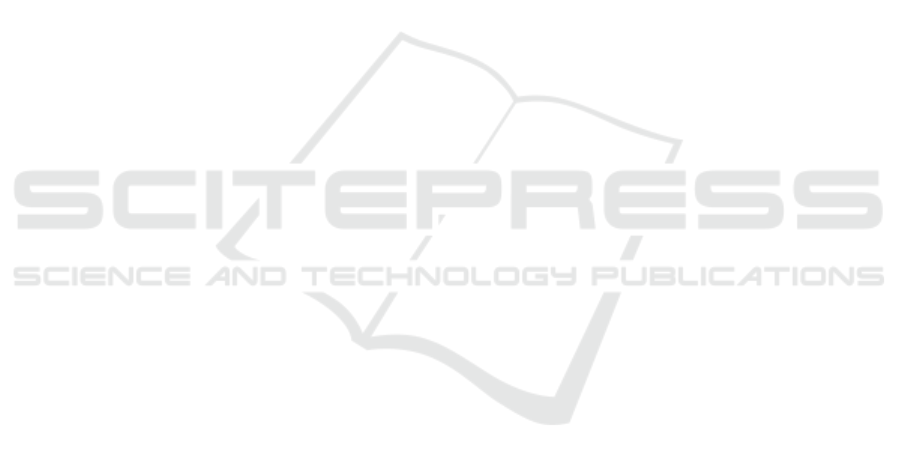
havior between the two connectors. So we have pro-
posed a new connector which allows a combination
of the variables taking into account the importance of
the variable as with weighted average. We used this
connector to merge the information about the consul-
tation of the Moodle resources to elaborate the partic-
ipation indicator. We have also proposed a connector
which takes into account the reinforcement of the in-
tensity of the variables. There are good results which
highlight the students with difficulties. But the main
problem is the performance limitation of the approach
for complex system modeling. We have to continue
the improvement of the performance and to find new
solutions to reduce the calculation time. We have to
complement the toolbox of Uncertain connectors with
new connectors which can be used for information fu-
sion. A vast experimentation is also needed to eval-
uate the pedagogical impact of this approach on stu-
dents and teachers but also on skill attainment. We
also have to perform comparative studies with other
approaches for further investigation.
REFERENCES
Amor, N. B. and Benferhat, S. (2005). Graphoid properties
of qualitative possiblistic independance relations. In
International Journal of Uncertainty, Fuzzyness and
Knowledge Based Systems, volume 13, pages 59–96.
Audigier, V., Husson, F., and Josse, J. (2015). A princi-
pal components method to impute missing values for
mixed data. In Advances in Data Analysis and Clas-
sification, volume 1, pages 5–26.
Baker, R. and Yacef, K. (2009). The state of educational
data mining in 2009: A review and future visions. In
Journal of Educational Data Mining, volume 1, pages
3–17.
Benferhat, S., Dubois, D., Garcia, L., and Prade, H. (1999).
Possibilistic logic bases and possibilistic graphs. In
In Proc. of the Conference on Uncertainty in Artificial
Intelligence, pages 57–64.
Benferhat, S. and Smaoui, S. (2004). Repr
´
esentation hy-
bride des r
´
eseaux causaux possibilistes. In Rencon-
tres francophones sur la Logique Floue et ses Appli-
cations, pages 43–50, Nantes, France. C
´
epadu
´
es Edi-
tions.
Borgelt, C., Gebhardt, J., and Kruse, R. (2000). Possibilistic
graphical models. In Computational Intelligence in
Data Mining, volume 26, pages 51–68. Springer.
Bousbia, N., Labat, J. M., Balla, A., and Reba, I. (2010).
Analyzing learning styles using behavioral indicators
in web based learning environments. In EDM 2010 In-
ternational Conference on Educational Data Mining,
pages 279–280, Pittsburgh, USA.
Caglioni, M., Dubois, D., Fusco, G., Moreno, D., Prade,
H., Scarella, F., and Tettamanzi, A. G. B. (2014).
Mise en oeuvre pratique de r
´
eseaux possibilistes pour
mod
´
eliser la sp
´
ecialisation sociale dans les espaces
m
´
etropolis
´
ees. In Rencontres Francophones sur la
Logique Floue et ses Applications (LFA 2014), pages
267–274, Carg
`
ese, France. C
´
epadu
´
es Editions.
D
`
ıez, F. and Drudzel, M. (2007). Canonical probabilistic
models for knowledge engineering. In Technical Re-
port CISIAD-06-01.
Dubois, D., Fusco, G., Prade, H., and Tettamanzi, A. G. B.
(2015). Uncertain logical gates in possibilistic net-
works. an application to human geography. In Scal-
able Uncertainty Management 2015, pages 249–263,
Qu
´
ebec, QC, Canada. Springer.
Dubois, D. and Prade, H. (1988). Possibility theory: An
Approach to Computerized Processing of Uncertainty.
Plenum Press, New York.
Heckerman, D. and Breese, J. (1994). A new look at causal
independence. In Proceedings of the Tenth Annual
Conference on Uncertainty in Artificial Intelligence
(UAI94), pages 286–292, San Francisco, USA. CA.
Morgan Kaufmann Publishers.
Huebner, R. A. (2013). A survey of educational data mining
research. In Research in Higher Education Journal,
volume 19.
Kjaerulff, U. (1994). Reduction of computational complex-
ity in bayesian networks through removal of week de-
pendences. In Proceeding of the 10th Conference on
Uncertainty in Artificial Intelligence. Morgan Kauf-
mann publishers.
Kruskal, J. B. (1956). On the shortest spanning subtree
of a graph and the travelling salesman problem. In
Proceedings of the American Mathematical Society,
pages 48–50.
Lauritzen, S. and Spiegelhalter, D. (1988). Local compu-
tation with probabilities on graphical structures and
their application to expert systems. In Journal of the
Royal Statistical Society.
Neapolitan, R. E. (1990). Probabilistic reasoning in expert
systems: theory and algorithms. John Wiley & Sons,
New-York, USA.
Pearl, J. (1988). Probabilistic reasoning in intelligent sys-
tems: networks of plausible inference. Morgan Kauf-
man Publishers, San Mateo, USA, 2nd edition.
van Gerven, M. A. J., Jurgelenaite, R., Taal, B. G., Heskes,
T., and Lucas, P. J. F. (2006). Predicting carcinoid
heart disease with the noisy-threshold classifier. In
Artificial Intelligence in Medicine, volume 40, pages
45–55.
Zadeh, L. A. (1978). Fuzzy sets as a basis for a theory
of possibility. In Fuzzy Sets and Systems, volume 1,
pages 3–28.
Zagorecki, A. and Druzdzel, M. J. (2006). Probabilistic
independence of causal influences. In Probabilistic
Graphical Models, pages 325–332.
ICEIS 2018 - 20th International Conference on Enterprise Information Systems
386