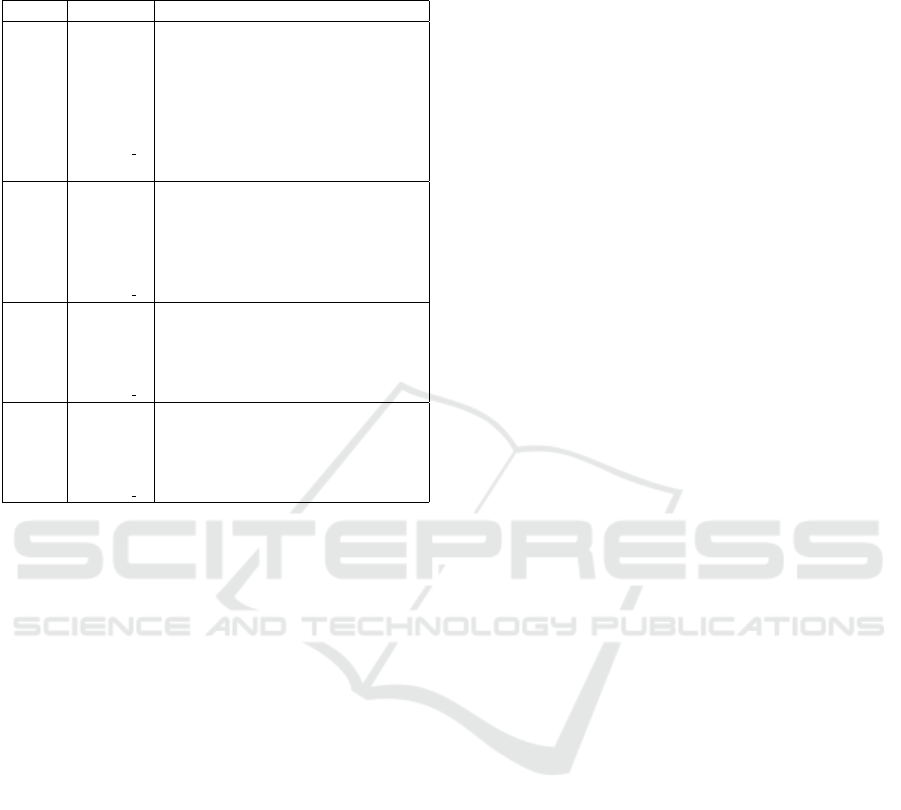
Table 6: Most popular smartphone models in the dataset
with dominant users and adjectives used to express a judg-
ment on each smartphone.
smartphoneuser keywords
Sony twandroid good, thin, comparative, immediate
Xperia Z3 magikstar29 beautiful, efficient, comparative, new, different,
the best, compact, thin, anti-overheating, advan-
tageous, good
phonandroid disponible,internationale,excessive,new,fast
kinghousse portable
droidtrackr fr beautiful, efficient, top, compact, good, top, bur-
ning, new, thin, the best, fast
Samsung twandroid good, vulnerable, attractive
Galaxy S5 magikstar29 compatible, huge, new, expensive, good, at-
tractive, superior, vulnerable
phonandroid good, superior, successful
kinghousse mini, rigid, expensive, portable, light, fine
droidtrackr fr compatible,good,vulnerable,attractive,superior
Sony twandroid good, bluetooth
Xperia Z3 magikstar29 compact, big
Compact phonandroid
kinghousse
droidtrackr fr compact, good
LG G3 twandroid good, available
magikstar29 the best, new, excellent, big, different, good
phonandroid small, good
kinghousse rigid, portable
droidtrackr fr the best, good, hard, super
are the top influencers and what characterizes them,
which products are generating more interest, which
opinions are associated with each product by the aut-
horitative users etc. These factors can help in the im-
plementation of effective techniques of viral marke-
ting and recommender systems.
The algorithms applied in the various steps of the
methodology can be extended and improved. For
instance, the product perception is based on opini-
ons expressed through simple extracted adjectives and
hashtags. In the future, we intend to apply more accu-
rate techniques of sentiment analysis and opinion mi-
ning (Medhat et al., 2014). In addition, we would
recognize accurate entity-targets of the opinions, i.e.,
not just the products but also features of them. In this
case, we could extend our model as a four-layer net-
work to analyze, for instance, which are the most re-
quired features discussed from users.
REFERENCES
Adomavicius, G. and Tuzhilin, A. (2005). Toward the next
generation of recommender systems: A survey of the
state-of-the-art and possible extensions. IEEE Trans.
on Knowl. and Data Eng., 17(6):734–749.
Almgren, K. and Lee, J. (2015). Who influences whom:
Content-based approach for predicting influential
users in social networks. In Proceedings of the Inter-
national Conference on Advances in Big Data Analy-
tics, pages 89–95.
Anger, I. and Kittl, C. (2011). Measuring influence on twit-
ter. In Proceedings of the 11th International Con-
ference on Knowledge Management and Knowledge
Technologies, pages 31–34. ACM.
Brin, S. and Page, L. (1998). The anatomy of a large-scale
hypertextual web search engine. Comput. Netw. ISDN
Syst., 30(1-7):107–117.
Cha, M., Haddadi, H., Benevenuto, F., and Gummadi, K.
(2010). Measuring user influence in twitter: The mil-
lion follower fallacy. In 4th International AAAI Confe-
rence on Weblogs and Social Media (ICWSM), pages
10–17.
Conover, W. (1980). Practical Non-Parametric Statistics,
2nd edn. John Wiley and Sons, New York.
Kleinberg, J. M. (1999). Authoritative sources in hyperlin-
ked environment. Journal of the ACM, 46(5):604–632.
Kolda, T. G., Bader, B. W., and Kenny, J. P. (2005). Higher-
order web link analysis using multilinear algebra. In
Proc. of the 5th International Conference on Data Mi-
ning (ICDM’05), pages 242–249.
Medhat, W., Hassan, A., and Korashy, H. (2014). Sentiment
analysis algorithms and applications: A survey. Ain
Shams Engineering Journal, 5(4):1093–1113.
Oro, E., Pizzuti, C., Procopio, N., and Ruffolo, M. (2017).
Detecting topic authoritative social media users: a
multilayer network approach. IEEE Transactions on
Multimedia.
Pawar, K. K., Shrishrimal, P. P., and Deshmukh, R. R.
(2015). Twitter sentiment analysis: A review. Interna-
tional Journal of Scientific and Engineering Research,
6(4):957–964.
Pirie, W. (1988). Spearman rank correlation coefficient. En-
cyclopedia of statistical sciences.
Probst, F., Grosswiele, L., and Pfleger, R. (2013). Who will
lead and who will follow: Identifying influential users
in online social networks - A critical review and future
research directions. Business & Information Systems
Engineering, 5(3):179–193.
Riquelme, F. and Cantergiani, P. G. (2016). Measuring user
influence on twitter: A survey. Inf. Process. Manage.,
52(5):949–975.
Weng, J., Lim, E.-P., Jiang, J., and He, Q. (2010). Twit-
terrank: Finding topic-sensitive influential twitterers.
In Proceedings of the Third ACM International Con-
ference on Web Search and Data Mining, WSDM ’10,
pages 261–270.
ICEIS 2018 - 20th International Conference on Enterprise Information Systems
584