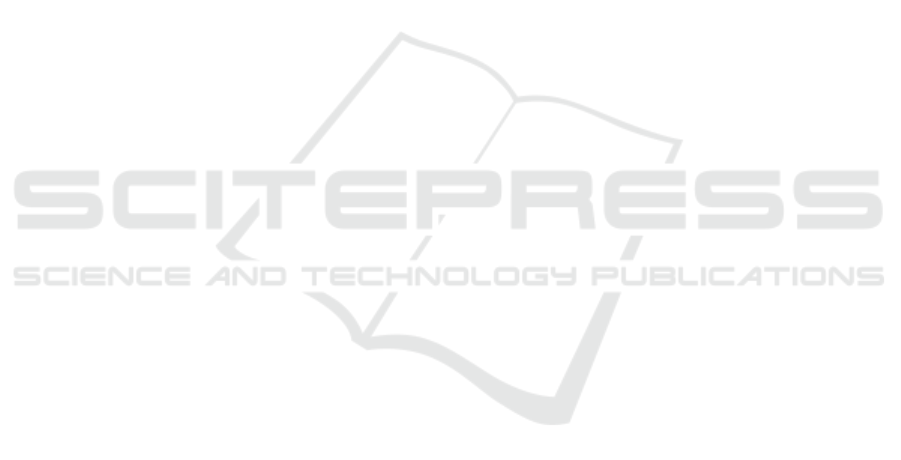
a substantial decrease in Vegetation and Bare land
cover due to the quarrying activity. Although Landsat
was able to measure land cover changes in the study
area, there were misclassifications due to spectral
similarities. Another limitation encountered during
the study was inability of Landsat to detect small
Water bodies within Granite quarries.
Recommendations that can address these limitations
in the future is the use of high spectral resolution data
such as hyperspectral remote sensing which is able to
distinguish between features with similar spectral
properties. Another recommendation is the use of
high spatial multispectral resolution data that is able
to detect small features such as water bodies within
granite quarries.
ACKNOWLEDGEMENTS
This study was sponsored by the University of
Johannesburg and Mintek.
REFERENCES
Abu, A. and Abdelall, S. (2014) ‘Sustainable development
of stone and marble sector in Palestine’, Journal of
Cleaner Production, 84, pp. 581–588.
Cawthorn, G. ., Eales, H. ., Walraven, F., Uken, R. and
Watkeys, M. . (2006) ‘The Bushveld Complex’, in
Johnson, M. ., Anhaeusser, C. ., and Thomas, R. . (eds)
The Geology of South Africa. Johannesburg: Geological
Society of South Africa, pp. 261–281.
Chander, G., Markham, B. L. and Helder, D. L. (2009)
‘Summary of current radiometric calibration
coefficients for Landsat MSS, TM, ETM+, and EO-1
ALI sensors’, Remote Sensing of Environment. Elsevier
Inc., 113(5), pp. 893–903.
Charou, E., Stefouli, M., Dimitrakopoulos, D., Vasiliou, E.
and Mavrantza, O. D. (2010) ‘Using remote sensing to
assess impact of mining activities on land and water
resources’, Mine Water and the Environment, 29(1), pp.
45–52.
Congalton, R. G. and Green, K. (2008) Assessing the
Accuracy of Remotely Sensed Data: Principles and
Practices. Second Edi. Boca Raton: CRC Press.
Deer, P. (1995) ‘Digital change detection techniques in
remote sensing.’, p. 53.
Demirel, N., Emil, M. K. and Duzgun, H. S. (2011) ‘Surface
coal mine area monitoring using multi-temporal high-
resolution satellite imagery’, International Journal of
Coal Geology. Elsevier B.V., 86(1), pp. 3–11.
Duncan, E. E. and Kuma, J. S. (2009) ‘Open pit mining and
land use changes: An example from Bogosu-Prestea,
South West Ghana’, The Electronic Journal of
Information Systems in Developing Countries, 36(3),
pp. 1–10.
Fleiss, J. F. and Cohen, J. (1973) ‘The equivalence of
weighted kappa and the intraclass correlation
coefficient as measure of reliability’, Educational and
Psychological Measurement, 33, pp. 613–619.
Foody, G. M. (2002) ‘Status of land cover classification
accuracy assessment’, Remote Sensing of Environment,
80(1), pp. 185–201.
Gandhi, G. M., Parthiban, S., Thummalu, N. and Christy,
A. (2015) ‘Ndvi: Vegetation Change Detection Using
Remote Sensing and Gis - A Case Study of Vellore
District’, Procedia Computer Science. Elsevier Masson
SAS, 57, pp. 1199–1210.
Gonzalez, E. P., Lopez, J. S., Martin, J. D., Burgos, A. J.
and Agudo, J. P. (2006) ‘Analysis of the mobilization
of solid loads and heavy metals in runoff waters from
granite quarries’, Environmental Geology, 50(6), pp.
823–834.
Günther, O., Radermacher, F. J. and Riekert, W.-F. (1995)
‘Environmental Monitoring : Models , Methods , and
Systems’, in Environmental Informatics. Netherlands:
Springer, pp. 13–38.
Jackson, R. D. and Huete, A. R. (1991) ‘Interpreting
vegetation indices’, Preventive Veterinary Medicine,
11, pp. 185–200.
Jain, P. K. (2015) ‘Environmental Degradation Due to
Open Cast Mining Activities in Bundelkhand and
Gwalior Region of M. P., India’, 4(3), pp. 725–731.
Koppe, J. C. (1997) ‘impacts and mining reclamation of
ornamental stone quarries’, in Marinos, P. G., Koukis,
G. C., Tsiambaos, G. C., and Stournaras, G. C. (eds)
Engineering Geology and the Environment.
Netherlands: CRC Press, pp. 2441–2446.
Koruyan, K., Deliormanli, a. H., Karaca, Z., Momayez, M.,
Lu, H. and Yalçin, E. (2012) ‘Remote sensing in
management of mining land and proximate habitat’,
Journal of the Southern African Institute of Mining and
Metallurgy, 112(7), pp. 667–672.
Lam, N., Zhou, G. and Ju, W. (2008) ‘Relating Visual
Changes in Images with Spatial Metrics’, in Yuan, M.
and Hornsby, K. S. (eds) Understanding Dynamics of
Geographic Domains. Boca Raton, London: CRC
Press, pp. 115–132.
Lameed, G. and Ayodele, E. (2011) ‘Effect of quarrying
activity on biodiversity: Case study of Ogbere site,
Ogun State Nigeria’, African Journal of Environmental
Science and Technology, 4(11), pp. 740–750.
Latifovic, R. (2005) Satellite RemoteSensing in Assessing
the Environmental Impactsof Large-Scale Surface
Mining. PhD Thesis, Department De Genie Des Mines,
De La Metallurgie Et Des, Univerite Laval Quebec.
Latifovic, R., Fytas, K., Chen, J. and Paraszczak, J. (2005)
‘Assessing land cover change resulting from large
surface mining development’, International Journal of
Applied Earth Observation and Geoinformation, 7(1),
pp. 29–48.
Lein, J. K. (2014) ‘Toward a remote sensing solution for
regional sustainability assessment and monitoring’,
Sustainability (Switzerland), 6(4), pp. 2067–2086.
Quantifying Land Cover Changes Caused by Granite Quarries from 1973-2015 using Landsat Data
203