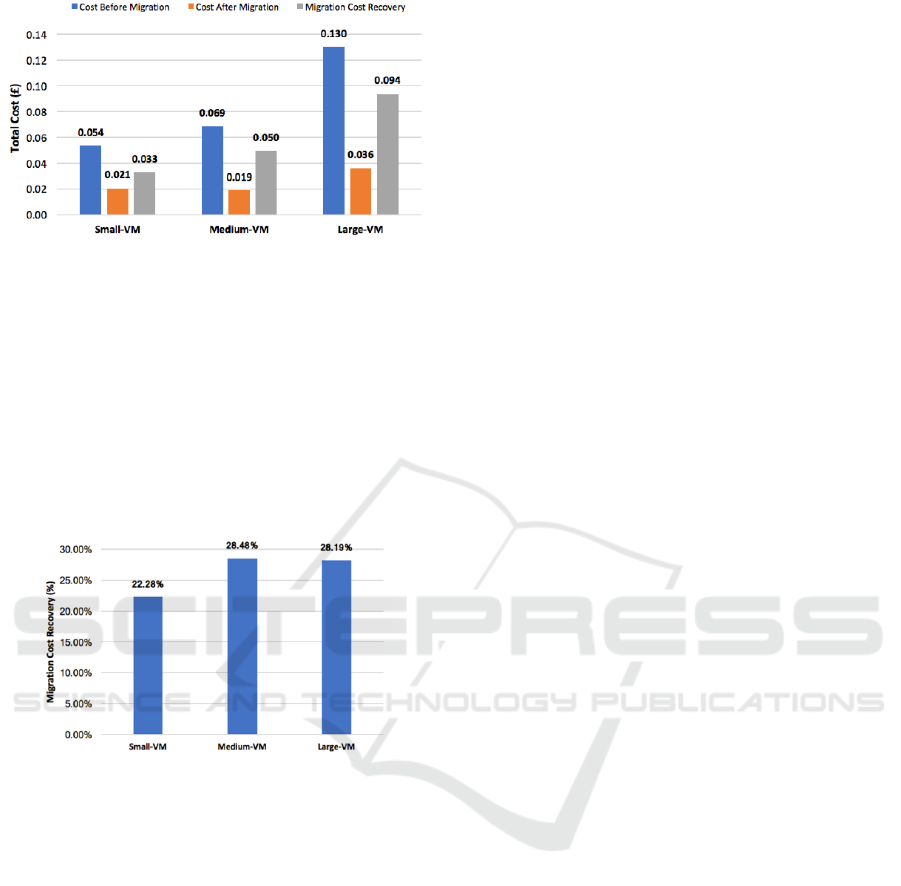
Figure 9: Predicted Total Cost Before vs After Migration
with The Migration Cost Recovery.
This framework is also capable of predicting the
total cost before and after live migration for a number
of VMs as shown in Figure 9, along with their
migration cost recovery based on Algorithm 3.
In addition, Figure 10 shows the results of the
predicted migration cost recovery for all VMs with
(the cost recovery percentage incurred by live
migration): 22.28% for the small VM, 28.48% for the
medium and 28.19% for the large one.
Figure 10: The Potential Migration Cost Recovery.
Despite the high variation of the workload
utilisation in the periodic pattern, the accuracy metrics
indicate that the predicted VMs workload and power
consumption achieve good prediction accuracy along
with the predicted live migration total cost.
6 CONCLUSION AND FUTURE
WORK
This paper has presented and evaluated a new
Performance and Energy-based Cost Prediction
Framework that dynamically supports VMs
reallocation, and demonstrates the trade-off between
cost, power consumption, and performance. This
framework predicts the total cost before and after live
migration by considering the resource usage, power
consumption and performance variation of
heterogeneous VMs based on their usage and size,
which reflect the physical resource usage and power
consumption by each VM. The results show that the
proposed framework can predict the resource usage,
power consumption, total migration cost and the
migration recovery cost for the VMs with a good
prediction accuracy based on periodic workload
patterns. As a part of future work, we intend to extend
our approach by considering the scalability aspects
(auto-scaling) to further understand the capability of
the proposed work.
REFERENCES
Aldossary, M., Alzamil, I. and Djemame, K. (2017)
‘Towards Virtual Machine Energy-Aware Cost
Prediction in Clouds’, in GECON 2017, pp. 119–131.
Alzamil, I. and Djemame, K. (2016) ‘Energy Prediction for
Cloud Workload Patterns’, in GECON 2016, pp.160–
174.
Box, G. E. P., Jenkins, G. M., Reinsel, G. C. and Ljung, G.
M. (2015) Time series analysis: forecasting and
control. John Wiley & Sons.
Dargie, W. (2015) ‘A stochastic model for estimating the
power consumption of a processor’, Computers, IEEE
Transactions on, pp. 1311–1322.
Fehling, C., Leymann, F., Retter, R., Schupeck, W. and
Arbitter, P. (2014) Cloud Computing Patterns.
Hyndman, R. J. and Athanasopoulos, G. (2013) Measuring
forecast accuracy, OTexts. Available at: at
www.otexts.org/fpp/2/5 (Accessed: 1 October 2017).
Liu, H., Jin, H., Xu, C.-Z. and Liao, X. (2013) ‘Performance
and energy modeling for live migration of virtual
machines’, Cluster Computing, 16(2), pp. 249–264.
Melhem, S. B., Agarwal, A., Goel, N. and Zaman, M.
(2017) ‘Selection process approaches in live migration:
A comparative study’, in ICICS 2017, pp. 23–28.
Raghunath, B. R. and Annappa B. (2017) ‘Prediction based
dynamic resource provisioning in virtualized
environments’, in ICCE 2017, pp. 100–105.
Strunk, A. (2012) ‘Costs of virtual machine live migration:
A survey’, in Proceedings - 2012 IEEE 8th World
Congress on Services, pp. 323–329.
Strunk, A. (2013) ‘A lightweight model for estimating
energy cost of live migration of virtual machines’, in
IEEE International Conference on Cloud Computing,
CLOUD, pp. 510–517.
Voorsluys, W., Broberg, J., Venugopal, S. and Buyya, R.
(2009) ‘Cost of virtual machine live migration in
clouds: A performance evaluation’, in Lecture Notes in
Computer Science (including subseries Lecture Notes
in Artificial Intelligence and Lecture Notes in
Bioinformatics), pp. 254–265.
Xu, F., Liu, F. and Jin, H. (2016) ‘Heterogeneity and
Interference-Aware Virtual Machine Provisioning for
Predictable Performance in the Cloud’, IEEE
Transactions on Computers, 65(8), pp. 2470–2483.
Performance and Energy-based Cost Prediction of Virtual Machines Live Migration in Clouds
391