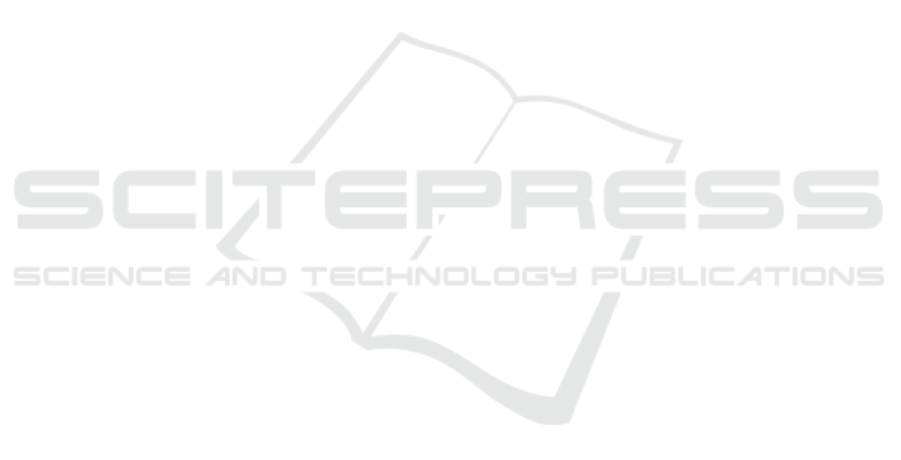
formance in predicting the solar radiation, especially
for the next 15, 30 and 45 minutes. As discussed, this
short-term forecast of solar radiation allows estima-
ting in advance the energy production of PV systems
with a good accuracy. This enables the design of more
accurate control policies for smart grids management,
such as Demand/Response.
ACKNOWLEDGEMENTS
This work was partially supported by the EU project
FLEXMETER and by the Italian project ”Edifici a
Zero Consumo Energetico in Distretti Urbani Intel-
ligenti”.
REFERENCES
Aghaei, J. and Alizadeh, M.-I. (2013). Demand response
in smart electricity grids equipped with renewable
energy sources: A review. Renewable and Sustainable
Energy Reviews, 18:64–72.
Badescu, V. (2014). Modeling solar radiation at the earth’s
surface. Springer.
Bottaccioli, L., Estebsari, A., Patti, E., Pons, E., and Acqua-
viva, A. (2017a). A novel integrated real-time simu-
lation platform for assessing photovoltaic penetration
impacts in smart grids. Energy Procedia, 111:780–
789.
Bottaccioli, L., Patti, E., Macii, E., and Acquaviva, A.
(2017b). Gis-based software infrastructure to model
pv generation in fine-grained spatio-temporal domain.
IEEE Systems Journal.
Box, G. E., Jenkins, G. M., Reinsel, G. C., and Ljung, G. M.
(2015). Time series analysis: forecasting and control.
John Wiley & Sons.
Brihmat, F. and Mekhtoub, S. (2014). Pv cell tempera-
ture/pv power output relationships homer methodo-
logy calculation. In International Journal of Scien-
tific Research & Engineering Technology, volume 1.
International Publisher &C. O.
Brockwell, P. J. and Davis, R. A. (2016). Introduction to
time series and forecasting. springer.
Chandrashekar, G. and Sahin, F. (2014). A survey on feature
selection methods. Computers & Electrical Engineer-
ing, 40(1):16–28.
Demuth, H. B., Beale, M. H., De Jess, O., and Hagan, M. T.
(2014). Neural network design. Martin Hagan.
Engerer, N. (2015). Minute resolution estimates of the dif-
fuse fraction of global irradiance for southeastern au-
stralia. Solar Energy, 116:215–237.
Gueymard, C. A. (2014). A review of validation methodo-
logies and statistical performance indicators for mo-
deled solar radiation data: Towards a better banka-
bility of solar projects. Renewable and Sustainable
Energy Reviews, 39:1024–1034.
Hamilton, J. D. (1994). Time series analysis, volume 2.
Princeton university press Princeton.
Han, S., Pool, J., Tran, J., and Dally, W. (2015). Learning
both weights and connections for efficient neural net-
work. In Advances in Neural Information Processing
Systems, pages 1135–1143.
Hansen, L. K. et al. (1994). Controlled growth of cascade
correlation nets. In ICANN94, pages 797–800. Sprin-
ger.
He, X. and Asada, H. (1993). A new method for identifying
orders of input-output models for nonlinear dynamic
systems. In American Control Conference, 1993, pa-
ges 2520–2523. IEEE.
Hofierka, J. and Ka
ˇ
nuk, J. (2009). Assessment of photo-
voltaic potential in urban areas using open-source so-
lar radiation tools. Renewable Energy, 34(10):2206–
2214.
Kaplanis, S. and Kaplani, E. (2016). Stochastic prediction
of hourly global solar radiation profiles.
Kubat, M. (2017). Artificial neural networks. In An Intro-
duction to Machine Learning, pages 91–111. Sprin-
ger.
Legates, D. R. and McCabe, G. J. (2013). A refined in-
dex of model performance: a rejoinder. International
Journal of Climatology, 33(4):1053–1056.
Linke, F. (1922). Transmissions-koeffizient und
tr
¨
ubungsfaktor. Beitr. Phys. Fr. Atmos, 10:91–103.
Ljung, L. (1998). System identification. In Signal analysis
and prediction, pages 163–173. Springer.
Madanchi, A., Absalan, M., Lohmann, G., Anvari, M.,
and Tabar, M. R. R. (2017). Strong short-term non-
linearity of solar irradiance fluctuations. Solar Energy,
144:1–9.
Miller, G. F., Todd, P. M., and Hegde, S. U. (1989). De-
signing neural networks using genetic algorithms. In
ICGA, volume 89, pages 379–384.
Montgomery, D. C., Jennings, C. L., and Kulahci, M.
(2015). Introduction to time series analysis and fo-
recasting. John Wiley & Sons.
Nazaripouya, H., Wang, B., Wang, Y., Chu, P., Pota, H.,
and Gadh, R. (2016). Univariate time series prediction
of solar power using a hybrid wavelet-arma-narx pre-
diction method. In Transmission and Distribution
Conference and Exposition (T&D), 2016 IEEE/PES,
pages 1–5. IEEE.
Norgaard, M., Ravn, O., and Poulsen, N. K. l. (2002).
Nnsysid-toolbox for system identification with neural
networks. Mathematical and computer modelling of
dynamical systems, 8(1):1–20.
Norgaard, P. M., Ravn, O., Poulsen, N. K., and Hansen,
L. K. (2000). Neural networks for modelling and con-
trol of dynamic systems-a practitioner’s handbook.
Oancea, B. and Ciucu, S¸. C. (2014). Time series fo-
recasting using neural networks. arXiv preprint
arXiv:1401.1333.
Qazi, A., Fayaz, H., Wadi, A., Raj, R. G., Rahim, N., and
Khan, W. A. (2015). The artificial neural network for
solar radiation prediction and designing solar systems:
a systematic literature review. Journal of Cleaner Pro-
duction, 104:1–12.
SMARTGREENS 2018 - 7th International Conference on Smart Cities and Green ICT Systems
52