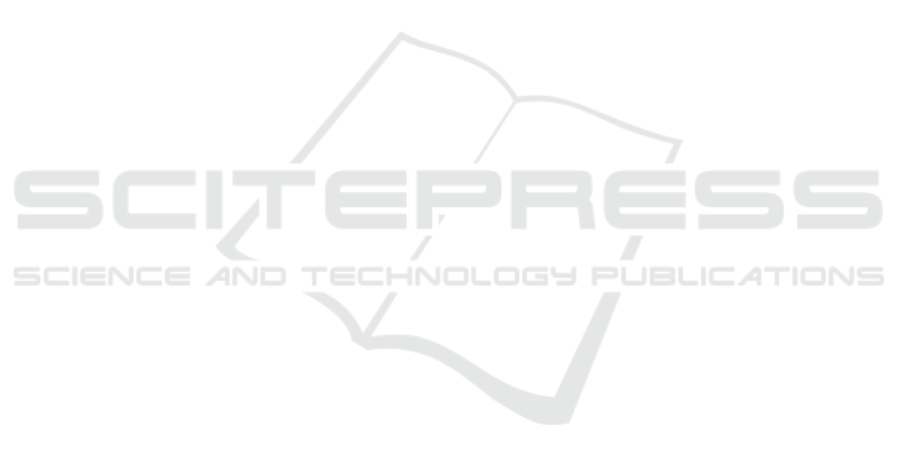
misconceptions.
Analyzing the results of the student id 6 presented
in the previous section it can be verified that
no ‘vicious-cycle’ could be detected nor repeated
occurrences or permanence in the Q2 or Q3
quadrants. Therefore, specific actions of the
educational software would not be necessary or
advisable for the students used as example.
Tutorial intervention strategies also could be
based on the results of ModHEmo. These intervention
strategies should not be applied to students who are
interested or focused on the activity, even if some
mistakes occurs. Furthermore, for students with
constant signs of frustration or annoyance (quadrants
Q2 and Q3) educational software could try strategies
such as a challenged or a game trying to alleviate the
effects of these negative states .
It is important to note that the construction of
this proposal was focused on the educational field,
but it is supposed that this approach, with some
adaptations, could be applied in other areas like
games, for example.
As a continuation of this work we intend to
improve the accuracy rate by tuning ModHEmo
parameters and implement and test others fusion
techniques. It is also intended to evaluate the results
obtained when teachers or specialists (psychologists)
perform the data labeling task.
Future works could be developed with the aim
of expanding the information contained in the
physical and cognitive components. Information like
head movements or fixation of the eyes in certain
components of the screen could be included in the
physical component. In the cognitive component
information such as interaction patterns with the
interface or previous knowledge of the student could
be considered.
ACKNOWLEDGMENT
The authors thank the Instituto Federal de Educac¸
˜
ao,
Ci
ˆ
encia e Tecnologia do RS - IFRS and the
Universidade Federal do Paran
´
a - UFPR for financial
support for this work.
REFERENCES
Alexander, S. T. V. (2008). An affect-sensitive intelligent
tutoring system with an animated pedagogical agent
that adapts to student emotion like a human tutor. PhD
thesis, Massey University, Albany, New Zealand.
Baker, R. S., Corbett, A. T., Koedinger, K. R., Evenson,
S., Roll, I., Wagner, A. Z., Naim, M., Raspat, J.,
Baker, D. J., and Beck, J. E. (2006). Adapting to
when students game an intelligent tutoring system.
In International Conference on Intelligent Tutoring
Systems, pages 392–401. Springer.
Baker, R. S., DMello, S., Rodrigo, M., and Graesser,
A. (2010). Better to be frustrated than bored:
The incidence and persistence of affect during
interactions with three different computer-based
learning environments. International Journal of
human-computer studies, 68(4):223–241.
Baker, R. S., Gowda, S., Wixon, M., Kalka, J., Wagner, A.,
Salvi, A., Aleven, V., Kusbit, G., Ocumpaugh, J., and
Rossi, L. (2012). Sensor-free automated detection of
affect in a cognitive tutor for algebra. In Educational
Data Mining 2012.
Becker-Asano, C. and Wachsmuth, I. (2010). Affective
computing with primary and secondary emotions in
a virtual human. Autonomous Agents and Multi-Agent
Systems, 20(1):32.
Calvo, R. A. and D’Mello, S. (2010). Affect detection:
An interdisciplinary review of models, methods, and
their applications. IEEE Transactions on affective
computing, 1(1):18–37.
Conati, C. (2011). Combining cognitive appraisal and
sensors for affect detection in a framework for
modeling user affect. In New perspectives on affect
and learning technologies, pages 71–84. Springer.
D’Mello, S. and Graesser, A. (2012). Dynamics of
affective states during complex learning. Learning
and Instruction, 22(2):145–157.
D’Mello, S., Picard, R. W., and Graesser, A. (2007). Toward
an affect-sensitive autotutor. IEEE Intelligent Systems,
22(4).
Ekman, P. (1992). An argument for basic emotions.
Cognition & emotion, 6(3-4):169–200.
Gebhard, P. (2005). Alma: a layered model of affect.
In Proceedings of the fourth international joint
conference on Autonomous agents and multiagent
systems, pages 29–36. ACM.
Grafsgaard, J. F., Wiggins, J. B., Boyer, K. E., Wiebe,
E. N., and Lester, J. C. (2013). Embodied affect
in tutorial dialogue: student gesture and posture. In
International Conference on Artificial Intelligence in
Education, pages 1–10. Springer.
Jaques, P. A., Pesty, S., and Vicari, R. (2003). An animated
pedagogical agent that interacts affectively with the
student. AIED 2003, shaping the future of learning
through intelligent technologies, pages 428–430.
Khan, F. A., Graf, S., Weippl, E. R., and Tjoa, A. M. (2010).
Identifying and incorporating affective states and
learning styles in web-based learning management
systems. IxD&A, 9:85–103.
Kort, B., Reilly, R., and Picard, R. W. (2001). An
affective model of interplay between emotions
and learning: Reengineering educational pedagogy-
building a learning companion. In Advanced Learning
Technologies, 2001. Proceedings. IEEE International
Conference on, pages 43–46. IEEE.
Hybrid Model of Emotions Inference
449