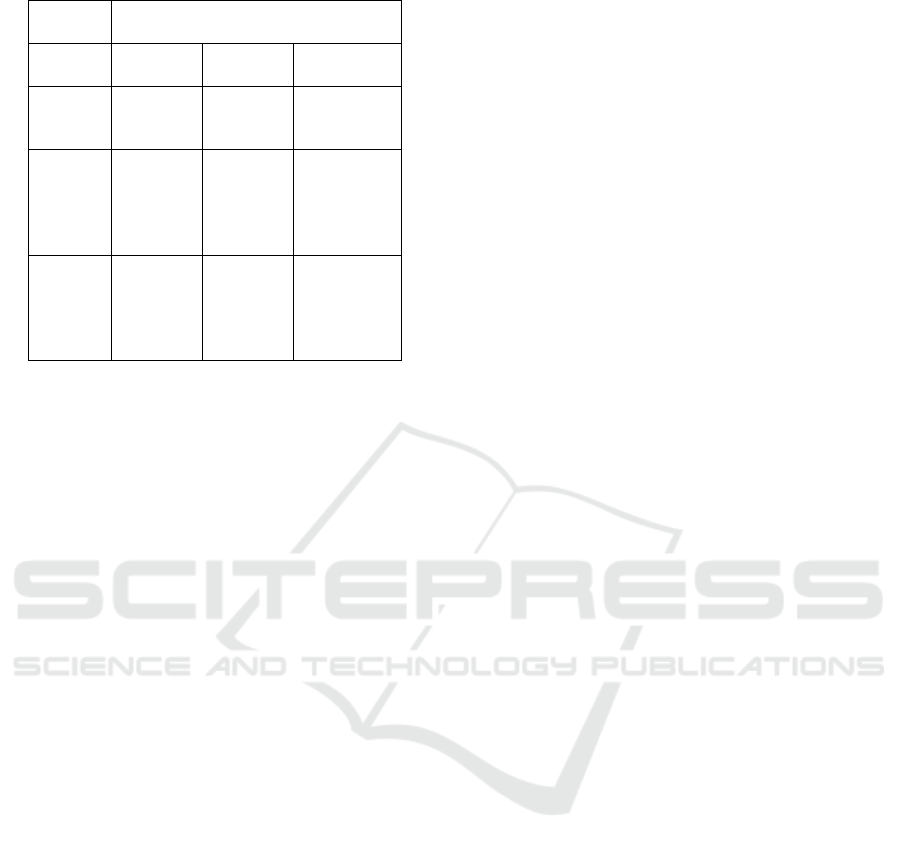
Table 2: Financial botnet traffic-based signatures.
Botnet
Periodic features
DNS HTTP TCP
Citadel domain content
flags
initial RTT
SpyEye
frame
— —TTL
response
Zeus —
content
—
request
response
cache
URL
Tinba —
content
flags
port
request
response
header
URL
5 CONCLUSIONS AND FUTURE
WORK
We considered four financial botnets and analyzed the
periodicity of their DNS, HTTP, and TCP traffic, ba-
sed on autocorrelation plots. In each case, we found
strong periodicity features in at least one of these
traffic types, while background traffic did not exhi-
bit any significant level of periodicity. Thus, autocor-
relation analysis of botnet traffic would enable us to
distinguish between the network activity of the four
financial botnets under consideration. That is, we can
construct highly discriminating signatures for each of
these four financial botnets based on periodicity fea-
tures, and their periods.
For future work, we will analyze the effectiveness
of the periodicity-based analysis presented in this pa-
per in a realistic networked environment. In such
a case, there will be a large volume of background
noise, and our goal is to determine how well we can
distinguish botnet traffic (based on periodicity featu-
res) in such a noisy environment. We believe the fea-
tures discussed here will prove strong by themselves
and, of course, we can consider combinations of peri-
odicity features with other aspects of botnet behavior.
This problem seems ideally suited to the application
of machine learning techniques and we plan to apply
a wide variety of such techniques. Hidden Markov
models (HMM) (Stamp, 2004), profile hidden Mar-
kov models (PHMM) (Durbin et al., 1998), support
vector machines (SVM) (Berwick, 2003), and neural
networks (Mukkamala et al., 2002) would appear to
be obvious candidates for application to this particu-
lar problem.
REFERENCES
Adamov, A., Hahanov, V., and Carlsson, A. (2014). Disco-
vering new indicators for botnet traffic detection. In
Proceedings of IEEE East-West Design Test Sympo-
sium (EWDTS 2014), pages 1–5.
Bach, O. (2015). Tinba: Worlds smallest malware has big
bag of nasty tricks. https://securityintelligence.com/
tinba-worlds-smallest-malware-has-big-bag-of-nasty-
tricks/. Accessed 2017-10-15.
Bailey, M., Cooke, E., Jahanian, F., Xu, Y., and Karir, M.
(2009). A survey of botnet technology and defenses.
In 2009 Cybersecurity Applications Technology Con-
ference for Homeland Security, pages 299–304.
BBC News (2013). FBI and Microsoft take down $500m-
theft botnet Citadel. BBC News, http://www.bbc.com/
news/technology-22795074. Accessed 2017-10-15.
Beigi, E. B., Jazi, H. H., Stakhanova, N., and Ghorbani,
A. A. (2014). Towards effective feature selection in
machine learning-based botnet detection approaches.
In 2014 IEEE Conference on Communications and
Network Security, pages 247–255.
Berwick, R. (2003). An idiots guide to support
vector machines (SVMs). http://www.svms.org/
tutorials/Berwick2003.pdf.
Bottazzi, G. and Me, G. (2015). A Survey on Financial Bot-
nets Threat. Springer International Publishing, Cham.
Butts, J. and Shenoi, S. (2011). Critical Infrastructure Pro-
tection V: 5th IFIP WG 11.10 International Confe-
rence on Critical Infrastructure Protection, Revised
Selected Papers. Springer Berlin Heidelberg.
Coogan, P. (2010). SpyEye bot versus Zeus bot. https://
www.symantec.com/connect/blogs/spyeye-bot-
versus-zeus-bot. Accessed 2017-10-15.
Durbin, R., Eddy, S., Krogh, A., and Mitchison, G. (1998).
Biological Sequence Analysis: Probabilistic Models
of Proteins and Nucleic Acids. Cambridge University
Press, Cambridge.
Eslahi, M., Rohmad, M. S., Nilsaz, H., Naseri, M. V., Ta-
hir, N., and Hashim, H. (2015). Periodicity classifi-
cation of http traffic to detect http botnets. In 2015
IEEE Symposium on Computer Applications & Indus-
trial Electronics (ISCAIE), pages 1–5.
Garcia, S., Grill, M., Stiborek, J., and Zunino, A. (2014).
An empirical comparison of botnet detection methods.
Computers & Security, 45:100–123.
Hachem, N., Mustapha, Y. B., Granadillo, G. G., and Debar,
H. (2011). Botnets: Lifecycle and taxonomy. In 2011
Conference on Network and Information Systems Se-
curity, pages 1–8.
He, X., Papadopoulos, C., Heidemann, J., Mitra, U., and
Riaz, U. (2009). Remote detection of bottleneck links
using spectral and statistical methods. Computer Net-
works, 53(3):279–298.
Jin, Y., Ichise, H., and Iida, K. (2015). Design of detecting
botnet communication by monitoring direct outbound
dns queries. In 2015 IEEE 2nd International Confe-
rence on Cyber Security and Cloud Computing, pages
37–41.