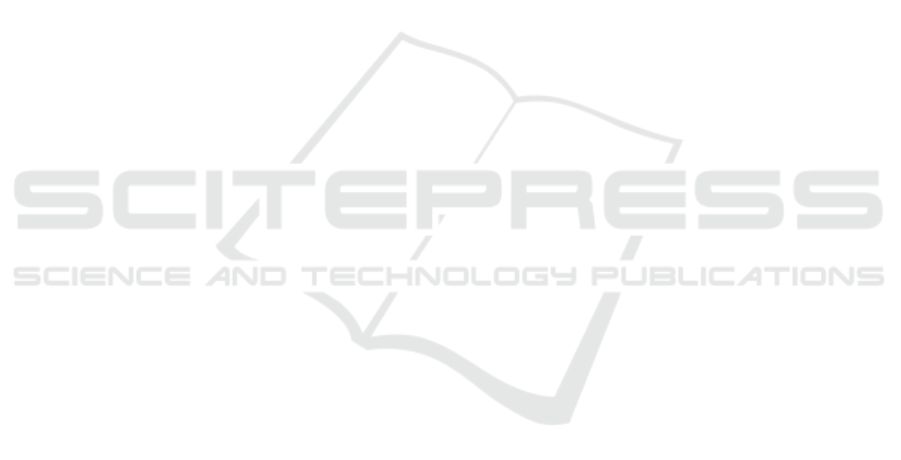
to give it meaning, b) View manipulation tools se-
lect items, navigate, explore, abstract/elaborate, en-
code the information, c) Process & Provenance tools
record, annotate, share visual configurations.
We propose to integrate the following interacti-
ons in our collaborative learning visualization : a) fil-
ter forums based on keywords, time stamps, pos-
ting/reading users, b) filter links based on quantitative
threshold, time stamps, users’ characteristics, c) sort
the nodes’ dispositions based on forum creation date,
most recent post, topics, or a hierarchy derived from
the users’ interactions, d) get detailed information
about a selected forum, e) see the path from forums
to forums for a selected user or cohort, f) zoom, pan,
scroll the forum network map, g) enable an overlay
instructor based concept map, h) annotate, comment
and share visualizations.
We understand that, as shown by Jivet et al.
(2017), awareness is not enough. With our inte-
ractive visualization, we hypothesize that the users
will engage in collaborative activities to find topics
of interest and collaborative partners, supporting their
learning goals without fostering competition. They
develop self-regulated competences.
5 CONCLUSION
In this paper we presented the challenges related to
modelling learning and collaborative learning. We
explained why the LA community focuses on desig-
ning and evaluating tools supporting and not repla-
cing human learning. We argued that visualizations
can be used to support some parts of learning if they
are well grounded in learning theories. We found that
static graph images of collaboration are not suitable
to support learners because they are only available at
the end of a course. Instead, we proposed a dyna-
mic visualization to support collaborative learning in
a self-regulated learning and socio-constructivist fra-
mework. Hence, our future challenge is to represent
in a real-time and intuitive way to the learners, the col-
laboration dynamics. We plan to achieve this using a
dynamic and interactive graph, which learners could
explore and derive insights from, about their learning
behaviour.
We are in the process of building a prototype to
evaluate how this will scale up to our final objective,
supporting MOOCs’ collaborative learning.
REFERENCES
Anaya, A. R., Luque, M., and Peinado, M. (2016). A vi-
sual recommender tool in a collaborative learning ex-
perience. Expert Systems with Applications, 45:248–
259.
Baker, R. S. (2016). Stupid Tutoring Systems, Intelligent
Humans. International Journal of Artificial Intelli-
gence in Education, 26(2):600–614.
Bandura, A. and McClelland, D. C. (1977). Social learning
theory. 35324.
Beck, F., Burch, M., Diehl, S., and Weiskopf, D. (2017). A
taxonomy and survey of dynamic graph visualization.
In Computer Graphics Forum, volume 36, pages 133–
159. Wiley Online Library.
Bertin, J. (1983). Semiology of Graphics: Diagrams, Net-
works, Maps. University of Wisconsin Press.
Boroujeni, M. S., Hecking, T., Hoppe, H. U., and Dillen-
bourg, P. (2017). Dynamics of MOOC discussion fo-
rums. In LAK, pages 128–137.
Bull, S. and Kay, J. (2016). SMILI: a framework for inter-
faces to learning data in open learner models, learning
analytics and related fields. International Journal of
Artificial Intelligence in Education, 26(1):293–331.
Dehaene, S. (2007). Neurones de la lecture (Les): La nou-
velle science de la lecture et de son apprentissage.
Odile Jacob.
Dillenbourg, P. (1999). What do you mean by collabora-
tive learning. Collaborative-learning: Cognitive and
computational approaches, 1:1–15. 02876.
Dillenbourg, P. (2002). Over-scripting CSCL: The risks of
blending collaborative learning with instructional de-
sign. Heerlen, Open Universiteit Nederland.
Duque, R., Gómez-Pérez, D., Nieto-Reyes, A., and Bravo,
C. (2015). Analyzing collaboration and interaction in
learning environments to form learner groups. Com-
puters in Human Behavior, 47:42–49.
Friedrich, C. and Eades, P. (2002). Graph drawing in mo-
tion. J. Graph Algorithms Appl., 6(3):353–370.
Gilbert, E. and Karahalios, K. (2009). Using social visuali-
zation to motivate social production. IEEE Transacti-
ons on Multimedia, 11(3):413–421.
Girshick, A. R., Landy, M. S., and Simoncelli, E. P. (2011).
Cardinal rules: visual orientation perception reflects
knowledge of environmental statistics. Nature Neu-
roscience, 14(7):926–932.
Govaerts, S., Verbert, K., Duval, E., and Pardo, A. (2012).
The student activity meter for awareness and self-
reflection. In CHI’12 Extended Abstracts on Human
Factors in Computing Systems, pages 869–884. ACM.
Heer, J. and Boyd, D. (2005). Vizster: Visualizing online
social networks. In Information Visualization, 2005.
INFOVIS 2005. IEEE Symposium on, pages 32–39.
IEEE.
Heer, J. and Shneiderman, B. (2012). Interactive dynamics
for visual analysis. Queue, 10(2):30.
Hommes, J., Rienties, B., de Grave, W., Bos, G., Schuwirth,
L., and Scherpbier, A. (2012). Visualising the invisi-
ble: a network approach to reveal the informal social
side of student learning. Advances in Health Sciences
Education, 17(5):743–757.
Towards a Dynamic Visualization of Online Collaborative Learning
211