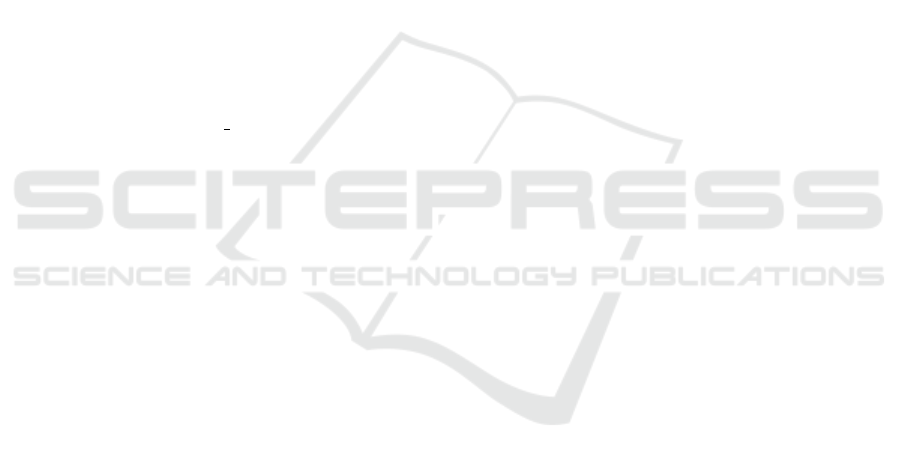
crossing was calculated by the proposed Bayesian
network. In this contribution, we focused on the rela-
tionship between the motion prediction and the inten-
tion estimation. We observed that these two phenom-
ena are dependent, however, the dependence is only
sub-linear. Despite the decreasing success of motion
prediction beyond 1st second, the intention estimation
was stable up to three seconds.
Our future work will aim at improvements
in the intention estimation independently on motion
prediction. We will test the success of the estimation.
We will also implement more sophisticated and com-
putationally intensive motion predictors for compari-
son.
ACKNOWLEDGEMENTS
This work was funded by the European Union H2020
Framework Programme for Research and Innovation
under the grant agreement No. 688652, UP-Drive.
Radoslav
ˇ
Skoviera and V
´
aclav Hlav
´
a
ˇ
c were also sup-
ported by the project R4I (Robotics for Industry 4.0,
No. CZ.02.1.01/0.0/0.0/15 003/0000470). The last
author received also funding from the project CAK,
Technology Agency of the Czech Republic grant No.
TE01020197.
REFERENCES
Ammoun, S. and Nashashibi, F. (2009). Real time trajec-
tory prediction for collision risk estimation between
vehicles. In Intelligent Computer Communication and
Processing, 2009. ICCP 2009. IEEE 5th International
Conference on, pages 417–422.
Armand, A., Filliat, D., and Ibaez-Guzman, J. (2014).
Ontology-based context awareness for driving assis-
tance systems. In 2014 IEEE Intelligent Vehicles Sym-
posium Proceedings, pages 227–233.
Br
¨
annstr
¨
om, M., Coelingh, E., and Sj
¨
oberg, J. (2010).
Model-based threat assessment for avoiding arbitrary
vehicle collisions. IEEE Trans. Intelligent Trans-
portation Systems, 11(3):658–669.
Gadepally, V., Krishnamurthy, A., and Ozguner, U. (2014).
A framework for estimating driver decisions near in-
tersections. IEEE Transactions on Intelligent Trans-
portation Systems, 15(2):637–646.
Gindele, T., Brechtel, S., and Dillmann, R. (2010). A prob-
abilistic model for estimating driver behaviors and ve-
hicle trajectories in traffic environments. In Intelli-
gent Transportation Systems (ITSC), 2010 13th Inter-
national IEEE Conference on, pages 1625–1631.
Gindele, T., Brechtel, S., and Dillmann, R. (2015). Learn-
ing driver behavior models from traffic observations
for decision making and planning. IEEE Intelligent
Transportation Systems Magazine, 7(1):69–79.
Hamdane, H., Serre, T., Masson, C., and Anderson, R.
(2015). Issues and challenges for pedestrian active
safety systems based on real world accidents. Acci-
dent Analysis & Prevention, 82:53–60.
Harnad, S. (1990). The symbol grounding problem. Phys-
ica, 44:335–446.
Kfer, E., Hermes, C., Whler, C., Ritter, H., and Kummert,
F. (2010). Recognition of situation classes at road in-
tersections. In Robotics and Automation (ICRA), 2010
IEEE International Conference on, pages 3960–3965.
Kooij, J. F. P., Schneider, N., Flohr, F., and Gavrila, D. M.
(2014). Context-Based Pedestrian Path Prediction,
pages 618–633. Springer International Publishing.
Kumar, P., Perrollaz, M., Lefvre, S., and Laugier, C. (2013).
Learning-based approach for online lane change in-
tention prediction. In Intelligent Vehicles Symposium
(IV), 2013 IEEE, pages 797–802.
Lef
`
evre, S., Laugier, C., and Iba
˜
nez-Guzm
´
an, J. (2013).
Intention-Aware Risk Estimation for General Traffic
Situations, and Application to Intersection Safety. Re-
search Report RR-8379, INRIA.
Lef
`
evre, S., Vasquez, D., and Laugier, C. (2014). A survey
on motion prediction and risk assessment for intelli-
gent vehicles. ROBOMECH Journal, 1(1):1–14.
Lefvre, S., Laugier, C., and Ibaez-Guzmn, J. (2012). Risk
assessment at road intersections: Comparing intention
and expectation. In Intelligent Vehicles Symposium
(IV), 2012 IEEE, pages 165–171.
M. Liebner, M. Baumann, F. K. and Stiller, C. (2012).
Driver intent inference at urban intersections using the
intelligent driver model. In Proc. IEEE Intelligent Ve-
hicles Symposium, page 11621167.
Morris, B., Doshi, A., and Trivedi, M. (2011). Lane change
intent prediction for driver assistance: On-road de-
sign and evaluation. In Intelligent Vehicles Symposium
(IV), 2011 IEEE, pages 895–901.
project-V-Charge (2011-2015). Automated Valet Parking
and Charging for e-Mobility (V-Charge). European
Commission funded project, FP7, No. 269916, 2011-
06-01 to 2015-09-30. Accessed: 2017-04-29.
Russell, S. (2015). Unifying logic and probability. Commu-
nication of ACM, 58(7):88–97.
Song, W., Xiong, G., and Chen, H. (2016). Intention-
aware autonomous driving decision-making in an un-
controlled intersection. Mathematical Problems in
Engineering, 25:775–807.
Tamke, A., Dang, T., and Breuel, G. (2011). A flexible
method for criticality assessment in driver assistance
systems. In Intelligent Vehicles Symposium (IV), 2011
IEEE, pages 697–702.
Tay, C. (2009). Analysis of Dynamic Scenes: Application
to Driving Assistance. Theses, Institut National Poly-
technique de Grenoble - INPG.
WHO (2013). Global status report on road safety: support-
ing a decade of action. World Health Organization
report.
VEHITS 2018 - 4th International Conference on Vehicle Technology and Intelligent Transport Systems
348