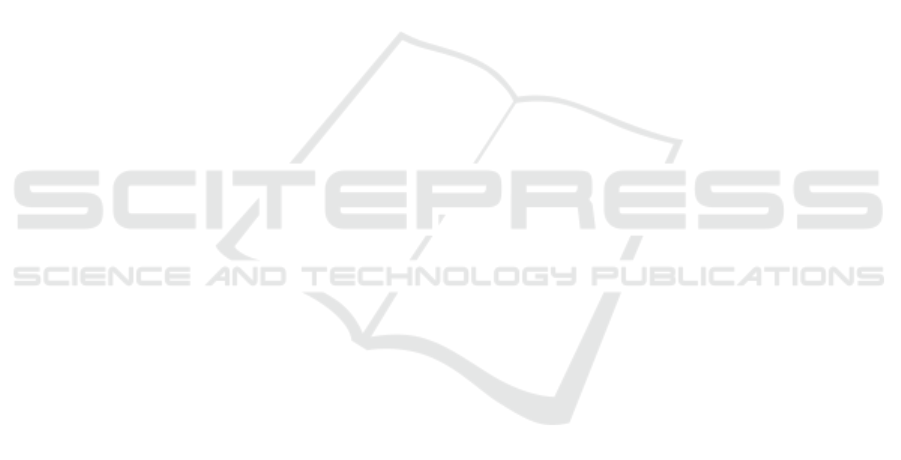
6 CONCLUSION
This study addresses the challenges for choosing suit-
able data analytics methods in the domain of low volt-
age smart grids. DSSE is an analytical method for
providing a reliable source of information related to
the state of the grid, by filtering the raw data and
detecting gross errors. Ideally, DSSE makes use of
near-real-time data to provide a successful estima-
tion. In many cases, this data is insufficient or non-
available, so pseudo-measurements generated from
historical data will fill in for the lack of information.
Traditional historic analytics can build predictive out-
puts useful for the DSSE, but there is a higher error
probability in the pseudo-measurements.
By this token, the data analytics module should
be built on a platform that can accommodate for both
historical and near-real-time analysis. The next step
in this research is to test the functionality of a DSSE
algorithm and analyze the capabilites of processing
large amounts of historical batch data. At the same
time, the test aims to characterize the performance
and bottlenecks of parallel processing of both stream
and batch data types, taking into account parame-
ters such as memory usage, processing time and in-
memory processing behavior.
ACKNOWLEDGEMENTS
This work is financially supported by the Danish
project RemoteGRID, which is a ForskEL program
under Energinet.dk with grant agreement no. 2016-1-
12399.
REFERENCES
Abreu, J. M., Pereira, F. C., and Ferr
˜
ao, P. (2012). Us-
ing pattern recognition to identify habitual behavior in
residential electricity consumption. Energy and build-
ings, 49:479–487.
Abu-El-Magd, M. A. and Findlay, R. D. (2003). A
new approach using artificial neural network and
time series models for short term load forecasting.
3:1723,1724,1725,1726.
Abur, A. and Exposito, A. G. (2004). Power system state
estimation: theory and implementation. CRC press.
Albert, A. and Rajagopal, R. (2013). Smart meter
driven segmentation: What your consumption says
about you. IEEE Transactions on Power Systems,
28(4):4019–4030.
Alimardani, A., Therrien, F., Atanackovic, D., Jatskevich,
J., and Vaahedi, E. (2015). Distribution system state
estimation based on nonsynchronized smart meters.
IEEE Transactions on Smart Grid, 6(6):2919–2928.
Ben
´
ıtez, I., Quijano, A., D
´
ıez, J.-L., and Delgado, I. (2014).
Dynamic clustering segmentation applied to load pro-
files of energy consumption from spanish customers.
International Journal of Electrical Power & Energy
Systems, 55:437–448.
CI and T (2014). The four types of analytics.
Commission, I. E. (2013). Application Integration at Elec-
tric Utilities - System Interfaces for Distribution Man-
agement - Part 100: Implementation Profiles. Techni-
cal Report 61968-100, International Electrotechnical
Commission.
Daki, H., El Hannani, A., Aqqal, A., Haidine, A., and
Dahbi, A. (2017). Big data management in smart grid:
concepts, requirements and implementation. Journal
of Big Data, 4(1):13.
Diamantoulakis, P. D., Kapinas, V. M., and Karagiannidis,
G. K. (2015). Big data analytics for dynamic energy
management in smart grids. 2:94–101.
Do Coutto Filho, M., Souza, J., Matos, R., and Schilling,
M. T. (1999). Preserving data redundancy in state es-
timation through a predictive database. In Electric
Power Engineering, 1999. PowerTech Budapest 99.
International Conference on, page 271. IEEE.
Energinet (2015). The Energinet.dk website.
Gartner Summits (2017). Gartner it glossary - advanced
analytics.
GJSissons (2014). Adaptive mapreduce: Part 1.
Gutierrez, D. D. (2016). InsideBIGDATA - Guide to
Streaming Analytics. Technical report, Impetus
Stream Analytix.
Halo Business Intelligence (2017). Descriptive, predictive,
and prescriptive analytics explained.
IEC/TC (2013). Application Integration at Electric Utili-
ties - System Interfaces for Distribution Management -
Part 9: Interfaces for meter reading and control. Tech-
nical Report 61968-9, International Electrotechnical
Commission.
Khodabakhshian, A., Hooshmand, R., and Raisee-
Gahrooyi, Y. (2017). A new pseudo load profile de-
termination approach in low voltage distribution net-
works. IEEE Transactions on Power Systems.
Krsman, V., Tesanovic, B., and Dojic, J. (2016). Pre-
processing of pseudo measurements based on ami data
for distribution system state estimation.
Liu, X., Golab, L., Golab, W., Ilyas, I. F., and Jin, S. (2016).
Smart meter data analytics. 42:1–39.
Martin-Loeches, R., Iov, F., Kemal, M., Stefan, M., and
Olsen, R. (2017). Observability of low voltage grids:
actual dsos challenges and research questions. In Pro-
ceedings of the 2017 52nd International Universities’
Power Engineering Conference (UPEC). IEEE Press.
McMorran, D. A. W. (2007). An introduction to iec 61970-
301 and 61968-11: The common information model.
Miceli, R., Favuzza, S., and Genduso, F. (2013). A perspec-
tive on the future of distribution: Smart grids, state of
the art, benefits and research plans. 5:36–42.
Neumann, S. A. and Nielsen, T. D. (2010). Cim interoper-
ability challenges. pages 1–5.
Data Analytics for Low Voltage Electrical Grids
227