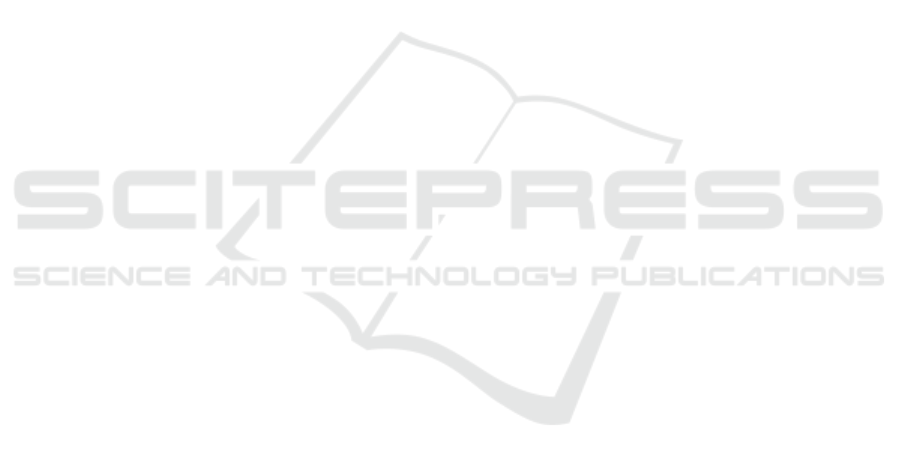
are available and filled in, the better the quality of rec-
ommended resources is. Nothing stands the use of our
system in other types of OLEs as long as the learner
and the MOOC profiles data can be extracted.
Our objective in the intermediate future is to carry out
the experiments as defined previously in this paper
in order to assess the adequacy of the recommended
resources with the learner and the MOOC profiles :
how much the resource we recommend to the learner
is adapted to his characteristics while respecting the
characteristics of the MOOC.
The results of these experiments will also allow us to
improve our system.
Then in the distant future we manage to assess our so-
lution in a more comprehensive way. More precisely
our objective is to integrate our recommender system
in an existing MOOC and to assess how it will be
working under real conditions. In this evaluation we
will be interested with the interactions between our
recommender system and learners with different pro-
files and how much the recommendations we offer to
learners support them during the MOOC. Another fu-
ture work is to use other characteristics of the learner
when computing recommendations to him. One of
these characteristics is the context of the learner that
can represent an important criteria to be taken into
consideration in the refinement module. In fact de-
pending on the nationality of the learner for example
several information can change as the teaching styles
or the educational levels.
REFERENCES
Agrawal, A., Venkatraman, J., Leonard, S., and Paepcke, A.
(2015). Youedu: addressing confusion in mooc dis-
cussion forums by recommending instructional video
clips.
Alario-Hoyos, C., Leony, D., Est
´
evez-Ayres, I., P
´
erez-
Sanagust
´
ın, M., Guti
´
errez-Rojas, I., and Kloos, C. D.
(2014). Adaptive planner for facilitating the manage-
ment of tasks in moocs. In V Congreso Internacional
sobre Calidad y Accesibilidad de la Formaci
´
on Vir-
tual, CAFVIR, pages 517–522.
Bansal, N. (2013). Adaptive recommendation system for
mooc. Indian Institute of Technology, pages 1–40.
Chowdhury, G. G. (2010). Introduction to modern informa-
tion retrieval. Facet publishing.
Fasihuddin, H. A., Skinner, G. D., and Athauda, R. I.
(2014). Personalizing open learning environments
through the adaptation to learning styles. ICITA.
Felder, R. M., Silverman, L. K., et al. (1988a). Learning and
teaching styles in engineering education. Engineering
education, 78(7):674–681.
Felder, R. M., Silverman, L. K., et al. (1988b). Learning and
teaching styles in engineering education. Engineering
education, 78(7):674–681.
Hajri, H., Bourda, Y., and Popineau, F. (2015). Querying
repositories of oer descriptions: The challenge of ed-
ucational metadata schemas diversity. In Design for
Teaching and Learning in a Networked World, pages
582–586. Springer.
Imran, H., Belghis-Zadeh, M., Chang, T.-W., Graf, S., et al.
(2016). Plors: a personalized learning object recom-
mender system. Vietnam Journal of Computer Sci-
ence, 3(1):3–13.
Kolb, A. Y. (2005). The kolb learning style inventory-
version 3.1 2005 technical specifications. Boston, MA:
Hay Resource Direct, 200:72.
Kolukuluri, S. (2014). XBlock-Courseware Component Ar-
chitecture. PhD thesis, Indian Institute of Technology,
Bombay Mumbai.
Li, S.-W. D. and Mitros, P. (2015). Learnersourced recom-
mendations for remediation. In Advanced Learning
Technologies (ICALT), 2015 IEEE 15th International
Conference on, pages 411–412. IEEE.
Maran, V., de Oliveira, J. P. M., Pietrobon, R., and Au-
gustin, I. (2015). Ontology network definition for
motivational interviewing learning driven by seman-
tic context-awareness. In Computer-Based Medical
Systems (CBMS), 2015 IEEE 28th International Sym-
posium on, pages 264–269. IEEE.
Paquette, G., Mari
˜
no, O., Rogozan, D., and L
´
eonard, M.
(2015). Competency-based personalization for mas-
sive online learning. Smart Learning Environments,
2(1):4.
Sahebi, S., Lin, Y.-R., and Brusilovsky, P. (2016). Tensor
factorization for student modeling and performance
prediction in unstructured domain. In Proceedings of
the 9th International Conference on Educational Data
Mining, pages 502–506. IEDMS.
Soloman, B. A. and Felder, R. M. (1999). Index of learning
styles questionnaire. Retrieved March, 26:2003.
Sunar, A. S., Abdullah, N. A., White, S., and Davis, H. C.
(2015). Personalisation of moocs: The state of the art.
A System to Recommend Open Educational Resources during an Online Course
109