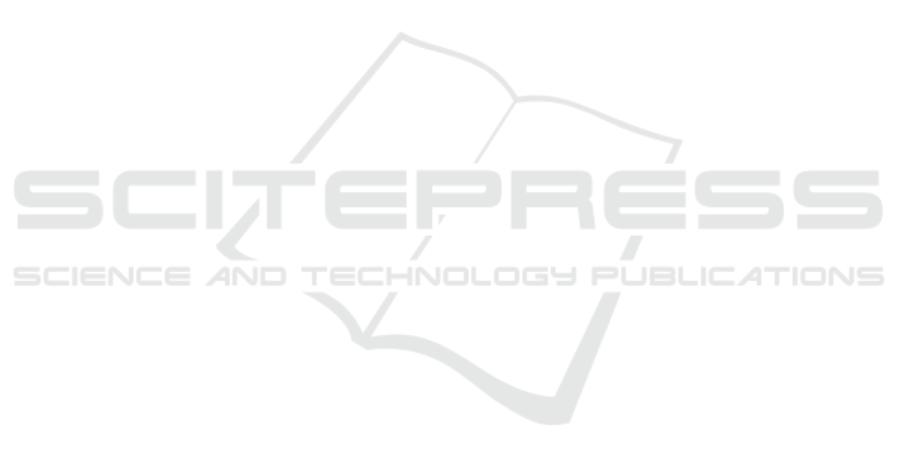
reported portray a positive picture or whether they
are negative. As a result sentiment analysis will be
performed. Sentiment analysis also known as
opinion mining involves the process of extracting
emotion or attitude of the writer, in order to
determine if the written piece is positive, negative or
even neutral.
REFERENCES
Ahlgren, P. and Colliander, C., 2009. Document–
document similarity approaches and science mapping:
Experimental comparison of five approaches. Journal
of informetrics, 3(1), pp.49-63.
Aly, M., 2005. Survey on multiclass classification
methods. Neural Netw, 19.
Andrienko, N. and Andrienko, G., 2006. Exploratory
analysis of spatial and temporal data: a systematic
approach. Springer Science & Business Media.
Bahl, L.R., Jelinek, F. and Mercer, R.L., 1983. A
maximum likelihood approach to continuous speech
recognition. IEEE transactions on pattern analysis and
machine intelligence, (2), pp.179-190.
Berenbaum, D., Deighan, D., Marlow, T., Lee, A., Frickel,
S. and Howison, M., 2016. Mining Spatio-temporal
Data on Industrialization from Historical Registries.
arXiv preprint arXiv:1612.00992.
Chasin, R., Woodward, D., Witmer, J. and Kalita, J., 2013.
Extracting and displaying temporal and geospatial
entities from articles on historical events. The
Computer Journal, 57(3), pp.403-426.
Chawla, N.V., Bowyer, K.W., Hall, L.O. and Kegelmeyer,
W.P., 2002. SMOTE: synthetic minority over-
sampling technique. Journal of artificial intelligence
research, 16, pp.321-357.
Dey, A.K., 2001. Understanding and using context.
Personal and ubiquitous computing, 5(1), pp.4-7.
Feldman, R. and Sanger, J., 2007. The text mining
handbook: advanced approaches in analyzing
unstructured data. Cambridge university press.
Fine, S., Singer, Y. and Tishby, N., 1998. The hierarchical
hidden Markov model: Analysis and applications.
Machine learning, 32(1), pp.41-62.
Jelinek, F., 1985. Markov source modeling of text
generation. In The impact of processing techniques on
communications (pp. 569-591). Springer Netherlands.
Joachims, T., 1998. Text categorization with support
vector machines: Learning with many relevant
features. Machine learning: ECML-98, pp.137-142.
Jones, CB. Purves, RS. 2008. (eds). Geographic
information retrieval. International Journal of
Geographical Information Sciences. Vol. 22, No. 3,
pp 219-228.
Keim, D., Andrienko, G., Fekete, J.D., Gorg, C.,
Kohlhammer, J. and Melançon, G., 2008. Visual
analytics: Definition, process, and challenges. Lecture
notes in computer science, 4950, pp.154-176.
Khan, A., Baharudin, B., Lee, L.H. and Khan, K., 2010. A
review of machine learning algorithms for text-
documents classification. Journal of advances in
information technology, 1(1), pp.4-20.
Kubat, M. and Matwin, S., 1997, July. Addressing the
curse of imbalanced training sets: one-sided selection.
In ICML (Vol. 97, pp. 179-186).
Li, Y.H. and Jain, A.K., 1998. Classification of text
documents. The Computer Journal, 41(8), pp.537-546.
Liu, B., Dai, Y., Li, X., Lee, W.S. and Yu, P.S., 2003,
November. Building text classifiers using positive and
unlabeled examples. In Data Mining, 2003. ICDM
2003. Third IEEE International Conference on (pp.
179-186). IEEE.
Manevitz, L. M., and Yousef, M. 2002. One-class svms
for document classification. Journal of Machine
Learning Research 2 (March 2002), pp. 139-154.
McCallum, A. and Nigam, K., 1998, July. A comparison
of event models for naive bayes text classification. In
AAAI-98 workshop on learning for text categorization
(Vol. 752, pp. 41-48).
Mehra, N. and Gupta, S., 2013. Survey on multiclass
classification methods.International Journal of
Computer Science and Information Technologies,
Vol.4 (4), pp. 572- 576.
Mei, Q. and Zhai, C., 2005, August. Discovering
evolutionary theme patterns from text: an exploration
of temporal text mining. In Proceedings of the
eleventh ACM SIGKDD international conference on
Knowledge discovery in data mining (pp. 198-207).
Mirrezaei, S.I., Martins, B. and Cruz, I.F., 2016, October.
A distantly supervised method for extracting spatio-
temporal information from text. In Proceedings of the
24th ACM SIGSPATIAL International Conference on
Advances in Geographic Information Systems (p. 74).
Nadeau, D. and Sekine, S., 2007. A survey of named
entity recognition and classification. Lingvisticae
Investigationes, 30(1), pp.3-26.
Nonnemaker, J. and Baird, H.S., 2009. Using synthetic
data safely in classification. Bold, 2, p.76.
Ramesh, B. and Sathiaseelan, J.G.R., 2015. An advanced
multi class instance selection based support vector
machine for text classification. Procedia Computer
Science, 57, pp.1124-1130.
Řehůřek, R. and Sojka, P., 2010. Software Framework for
Topic Modelling with Large Corpora. Proceedings of
the LREC 2010 Workshop on New Challenges for
NLP Frameworks, Malta: ELRA (pp. 45 - 50).
Rennie, Jason D. M., 2001. Improving Multi-class Text
Classification with Naive Bayes. Master of Science.
Cambridge, Massachusetts, USA: Massachusetts
Institute of Technology.
Robertson, C. and Horrocks, K., 2017. Spatial Context
from Open and Online Processing (SCOOP):
Geographic, Temporal, and Thematic Analysis of
Online Information Sources. ISPRS International
Journal of Geo-Information, 6(7), p.193.
Song, Y. and Roth, D., 2014, July. On Dataless
Hierarchical Text Classification. In AAAI (Vol. 7).
An Automated Approach to Mining and Visual Analytics of Spatiotemporal Context from Online Media Articles
221