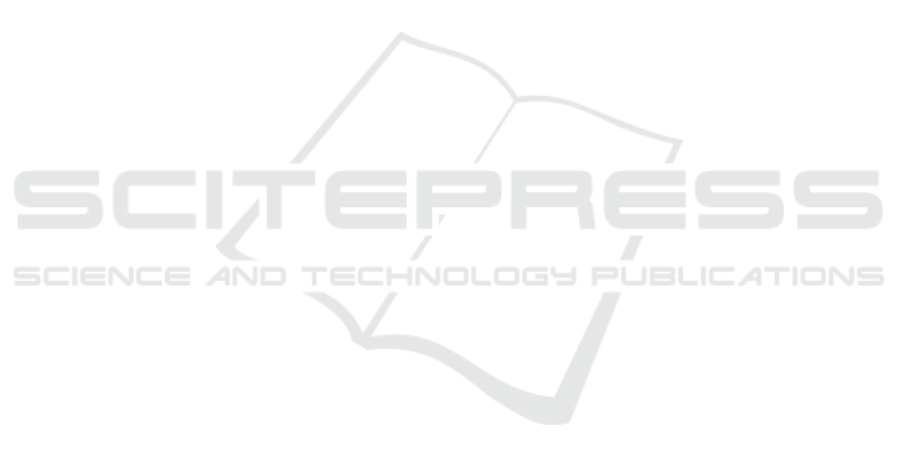
REFERENCES
Calegari, M. d., & Gemignani, O. H. (2006). Temperamento
e Carreira. São Paulo: Summus Editorial.
Feldman, R., & Sanger, J. (2007). The Text Mining
Handbook: Advanced approaches in Analyzing
Unstructured Data. Cambridge university press.
Gundecha, P., & Liu, H. (2012). Mining social media: a
brief introduction. New Directions in Informatics,
Optimization, Logistics, and Production. Informs, pp.
1-17.
Haddi, E., Liu, X., & Shi, Y. (2013). The role of text pre-
processing in sentiment analysis. Procedia Computer
Science, 17, 26-32.
Hall, C. S., Lindzey, G., & Campbell, J. B. (2000). Teorias
da Personalidade. Porto Alegre: Artmed.
Ito, P. d., & Guzzo, R. S. (2002). Diferenças individuais:
temperamento e personalidade; importância da teoria.
Estudos de Psicologia, pp. 91-100.
Keirsey, D. (1998). Please Undestand Me II: Temperament,
Character, Intelligence. Prometheus Nemesis Book
Company.
Keirsey, D. M. (1996). Keirsey.com. (Corporate Offices)
Acesso em 12/10/2017 de 10 de 2017, disponível em
://www.keirsey.com/4temps/overview_temperaments.
asp
Komisin, M. C., & Guinn, C. I. (2012). Identifying
personality types using document classification
methods. In: FLAIRS Conference.
Kwak, H., Lee, C., Park, H., & Moon, S. (2010). What is
Twitter, a social network or a news media? Proceedings
of the 19th international conference on World wide
web. ACM., 591-600.
Lima, A. C. (2016). Mineração de Mídias Sociais como
Ferramenta para a Análise da Tríade da Persona
Virtual. São Paulo.
Lima, A. C., & de Castro, L. N. (2016). Predicting
Temperament from Twitter Data. Advanced Applied
Informatics (IIAI-AAI), 2016 5th IIAI International
Congress on. IEEE.
Nor Rahayu, N., Zainol, Z., & Yoong, T. L. (2016). A
comparative study of different classifiers for automatic
personality prediction. Control System, Computing and
Engineering (ICCSCE), 2016 6th IEEE International
Conference on. IEE, 435-440.
Pedregosa, F., Varoquaux, G., Gramfort, A., Michel, V.,
Thirion, B., Grisel, O., . . . Perro, M. (2011). Scikit-
learn: Machine learning in Python. Journal of Machine
Learning Research 12.Oct, 12, 2825-2830.
Pennebaker, J. W., & King, L. A. (1999). Linguistic styles:
language use as an individual difference. Journal of
personality and social psychology, 77(6), 1296.
Pennebaker, J. W., Boyd, R. L., Jordan, K., & Blackburn,
K. (2015). The development and psychometric
properties of LIWC2015.
Plank, B., & Dirk, H. (2015). Personality Traits on Twitter-
or-How to Get 1, 500 Personality Tests in a Week.
Proceedigs of the 6th Workshop on Computational
Approaches to Subjectivity, Sentiment and Social
Media Analysis(WASSA 2015), pp. 92-98.
Spencer, J., & Uchyigit, G. (2012). Sentimentor: Sentiment
analysis of twitter data. Proceedings of European
conference on machine learning and principles and
practice of knowledge discovery in databases.
Verhoeven, B., Daelemans, W., & Plank, B. (2016).
Twisty: A Multilingual Twitter Stylometry Corpus for
Gender and Personality Profiling. Proceedings of the
10th International Conference on Language Resources
and Evaluation.
Xavier, O. C., & Carvalho, C. L. (2011). Desenvolvimento
de Aplicações Sociais A Partir de APIs em Redes
Sociais Online. UFG. Goiânia.
ICAART 2018 - 10th International Conference on Agents and Artificial Intelligence
256