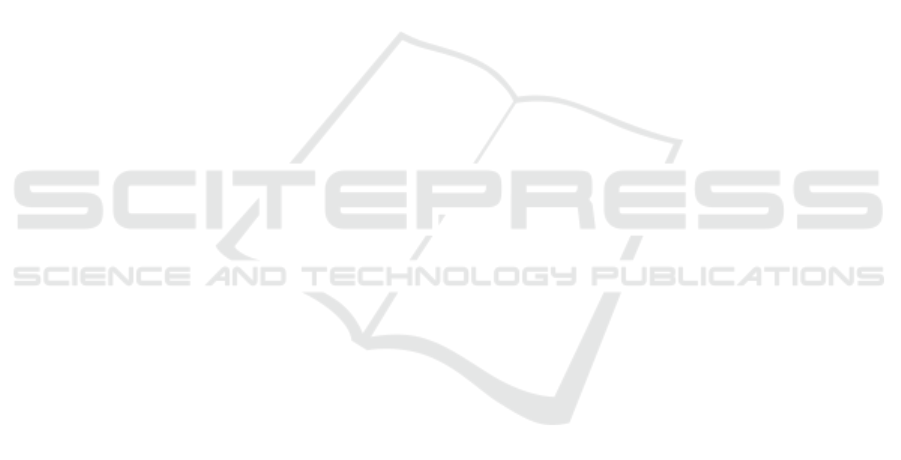
REFERENCES
Boykov, Y. Y. and Jolly, M. P. (2001). Interactive graph
cuts for optimal boundary amp; region segmentation
of objects in n-d images. In Proceedings Eighth IEEE
International Conference on Computer Vision. ICCV
2001, volume 1, pages 105–112 vol.1.
Chauhan, A. S., Silakari, S., and Dixit, M. (2014). Image
segmentation methods: A survey approach. In 2014
Fourth International Conference on Communication
Systems and Network Technologies, pages 929–933.
Chen, H., Ding, H., He, X., and Zhuang, H. (2014). Color
image segmentation based on seeded region growing
with canny edge detection. In 12th International Con-
ference on Signal Processing (ICSP), pages 683–686.
Dragon, T., Mavrikis, M., McLaren, B. M., Harrer, A.,
Kynigos, C., Wegerif, R., and Yang, Y. (2013).
Metafora: A web-based platform for learning to learn
together in science and mathematics. IEEE Transac-
tions on Learning Technologies, 6(3):197–207.
Freire, M. and Fern
´
andez-Manj
´
on, B. (2016). Metadata for
serious games in learning object repositories. IEEE
Revista Iberoamericana de Tecnologias del Apren-
dizaje, 11(2):95–100.
Garrido, A. and Onaindia, E. (2013). Assembling learning
objects for personalized learning: An ai planning per-
spective. IEEE Intelligent Systems, 28(2):64–73.
Hassan, H., Martinez-Rubio, J. M., Perles, A., Capella,
J. V., Dominguez, C., and Albaladejo, J. (2013).
Smartphone-based industrial informatics projects and
laboratories. IEEE Transactions on Industrial Infor-
matics, 9(1):557–566.
Huang, G. B., Zhou, H., Ding, X., and Zhang, R. (2012).
Extreme learning machine for regression and mul-
ticlass classification. IEEE Transactions on Sys-
tems, Man, and Cybernetics, Part B (Cybernetics),
42(2):513–529.
Hung, I.-C., Kinshuk, and Chen, N.-S. (2017). Embodied
interactive video lectures for improving learning com-
prehension and retention. Computers and Education.
Jo, J. H. and Lee, S. G. (2012). Sobel mask operations
using shared memory in cuda environment. In 2012
6th International Conference on New Trends in In-
formation Science, Service Science and Data Mining
(ISSDM2012), pages 289–292.
Koukopoulos, Z. and Koukopoulos, D. (2017). Integrat-
ing educational theories into a feasible digital envi-
ronment. Applied Computing and Informatics.
Kramida, G. (2016). Resolving the vergence-
accommodation conflict in head-mounted displays.
IEEE Transactions on Visualization and Computer
Graphics, 22(7):1912–1931.
Lantz, E. (2007). A survey of large-scale immersive dis-
plays. In Proceedings of the 2007 Workshop on
Emerging Displays Technologies: Images and Be-
yond: The Future of Displays and Interacton, EDT
’07, New York, NY, USA. ACM.
Lei, J., Wu, M., Zhang, C., Wu, F., Ling, N., and Hou,
C. (2017). Depth-preserving stereo image retargeting
based on pixel fusion. IEEE Transactions on Multi-
media, 19(7):1442–1453.
Liu, Z., Yang, C., Rho, S., Liu, S., and Jiang, F. (2017).
Structured entropy of primitive: big data-based stereo-
scopic image quality assessment. IET Image Process-
ing, 11(10):854–860.
M
´
endez, N. D. D., Morales, V. T., and Vicari, R. M.
(2016). Learning object metadata mapping with
learning styles as a strategy for improving usabil-
ity of educational resource repositories. IEEE Re-
vista Iberoamericana de Tecnologias del Aprendizaje,
11(2):101–106.
Oh, H., Kim, J., Kim, J., Kim, T., Lee, S., and Bovik, A. C.
(2017). Enhancement of visual comfort and sense of
presence on stereoscopic 3d images. IEEE Transac-
tions on Image Processing, 26(8):3789–3801.
Pedro, R. W. D., Nunes, F. L. S., and Machado-Lima, A.
(2013). Using grammars for pattern recognition in
images: A systematic review. ACM Comput. Surv.,
46(2):26:1–26:34.
Rossi, D. (2015). A hand-held 3d-printed box projector
study for a souvenir from a mixed-reality experience.
In 2015 Digital Heritage, volume 1, pages 313–316.
Santiago, R. and Raabe, A. (2010). Architecture for learn-
ing objects sharing among learning institutions 2014
lop2p. IEEE Transactions on Learning Technologies,
3(2):91–95.
Sanz-Rodriguez, J., Dodero, J. M. M., and Sanchez-Alonso,
S. (2010). Ranking learning objects through integra-
tion of different quality indicators. IEEE Transactions
on Learning Technologies, 3(4):358–363.
Sidharta, R., Hiyama, A., Tanikawa, T., and Hirose, M.
(2007). Volumetric display for augmented reality.
In 17th International Conference on Artificial Reality
and Telexistence (ICAT 2007), pages 55–62.
Sinclair, J., Joy, M., Yau, J. Y.-K., and Hagan, S. (2013).
A practice-oriented review of learning objects. IEEE
Transactions on Learning Technologies, 6(2):177–
192.
Tian, X. and Yu, W. (2016). Color image segmentation
based on watershed transform and feature clustering.
In 2016 IEEE Advanced Information Management,
Communicates, Electronic and Automation Control
Conference (IMCEC), pages 1830–1833.
Tsai, C.-F., McGarry, K., and Tait, J. (2006). Claire: A mod-
ular support vector image indexing and classification
system. ACM Trans. Inf. Syst., 24(3):353–379.
Wiley, D. A. (2002). The instructional use of learn-
ing objects: Online version. http://www.test.org/doe/
acessed in 2017/10/15.
Xu, J., Han, J., Nie, F., and Li, X. (2017). Re-weighted
discriminatively embedded k -means for multi-view
clustering. IEEE Transactions on Image Processing,
26(6):3016–3027.
Xu, R. and Wunsch, D. (2005). Survey of clustering al-
gorithms. IEEE Transactions on Neural Networks,
16(3):645–678.
CSEDU 2018 - 10th International Conference on Computer Supported Education
266