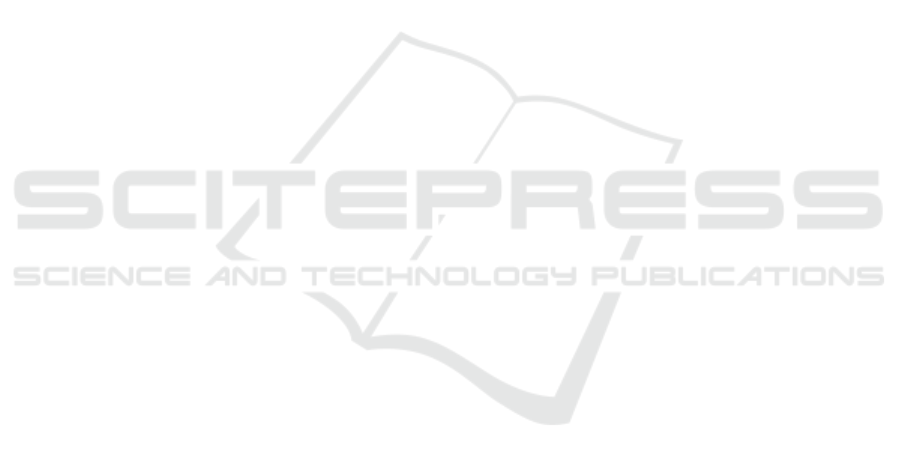
Loetsch, J. and Ultsch, A. (2016). Process pharmacology:
A pharmacological data science approach to drug de-
velopment and therapy. CPT: Pharmacometrics & Sy-
stems Pharmacology, 5(4):192–200.
Luo, G. (2016). A review of automatic selection methods
for machine learning algorithms and hyper-parameter
values. Network Modeling Analysis in Health Infor-
matics and Bioinformatics, 5(1):18.
Maltby, D. (2011). Big data analytics. In 74th Annual Meet-
ing of the Association for Information Science and
Technology (ASIST), pages 1–6.
Marvasti, M. A., Poghosyan, A. V., Harutyunyan, A. N.,
and Grigoryan, N. M. (2015). Ranking and updating
beliefs based on user feedback: Industrial use cases.
In 2015 IEEE International Conference on Autonomic
Computing, pages 227–230.
Matsushita, M., Maeda, E., and Kato, T. (2004). An inte-
ractive visualization method of numerical data based
on natural language requirements. International jour-
nal of human-computer studies, 60(4):469–488.
Medvedev, V., Kurasova, O., Bernatavi
ˇ
cien
˙
e, J., Treigys, P.,
Marcinkevi
ˇ
cius, V., and Dzemyda, G. (2017). A new
web-based solution for modelling data mining proces-
ses. Simulation Modelling Practice and Theory.
Mell, P. and Grance, T. (2011). The NIST definition of
cloud computing. NIST Special Publication, 800:145.
Michalski, S. R., Carbonell, G. J., and Mitchell, M. T.
(1983). Machine learning: An artificial intelligence
approach. Understanding the Nature of Learning,
2:3–26.
Nalchigar, S. and Yu, E. (2017). Conceptual modeling for
business analytics: A framework and potential bene-
fits. In Business Informatics (CBI), 2017 IEEE 19th
Conference on, volume 1, pages 369–378. IEEE.
Naous, D., Schwarz, J., and Legner, C. (2017). Analytics as
a service: Cloud computing and the transformation of
business analytics business models and ecosystems. In
Proceedings of the 25th European Conference on In-
formation Systems (ECIS), Guimares, Portugal, pages
487–501.
Narman, P., Johnson, P., Ekstedt, M., Chenine, M., and Ko-
nig, J. (2009). Enterprise architecture analysis for data
accuracy assessments. In Enterprise Distributed Ob-
ject Computing Conference, 2009. EDOC’09. IEEE
International, pages 24–33. IEEE.
Pruengkarn, R., Wong, K. W., and Fung, C. C. (2016).
Data cleaning using complementary fuzzy support
vector machine technique. In International Confe-
rence on Neural Information Processing, pages 160–
167. Springer.
Pyle, D. (1999). Data preparation for data mining, vo-
lume 1. Morgan Kaufmann.
Ribeiro, M., Grolinger, K., and Capretz, M. A. (2015).
Mlaas: Machine learning as a service. In Machine
Learning and Applications (ICMLA), 2015 IEEE 14th
International Conference on, pages 896–902. IEEE.
Riedemann, C. and Timm, C. (2003). Services for data in-
tegration. Data Science Journal, 2:90–99.
Rong, S., Niu, X., Xiang, E. W., Wang, H., Yang, Q., and
Yu, Y. (2012). A machine learning approach for in-
stance matching based on similarity metrics. In Inter-
national Semantic Web Conference, pages 460–475.
Springer.
Seibold, M. and Kemper, A. (2012). Database as a service.
Datenbank-Spektrum, 12(1):59–62.
Shearer, C. (2000). The crisp-dm model: the new blue-
print for data mining. Journal of data warehousing,
5(4):13–22.
Song, I.-Y., Zhu, Y., Ceong, H., and Thonggoom, O. (2015).
Methodologies for semi-automated conceptual data
modeling from requirements. In International Con-
ference on Conceptual Modeling, pages 18–31. Sprin-
ger.
Sun, Z., Strang, K., and Firmin, S. (2017). Business
analytics-based enterprise information systems. Jour-
nal of Computer Information Systems, 57(2):169–178.
Terzo, O., Ruiu, P., Bucci, E., and Xhafa, F. (2013). Data
as a service (daas) for sharing and processing of large
data collections in the cloud. In Complex, Intelligent,
and Software Intensive Systems (CISIS), 2013 Seventh
International Conference on, pages 475–480. IEEE.
Vargo, S. L. and Lusch, R. F. (2004). Evolving to a new
dominant logic for marketing. Journal of marketing,
68(1):1–17.
Vu, Q. H., Pham, T.-V., Truong, H.-L., Dustdar, S., and
Asal, R. (2012). Demods: A description model for
data-as-a-service. In Advanced Information Networ-
king and Applications (AINA), 2012 IEEE 26th Inter-
national Conference on, pages 605–612. IEEE.
Wagner, M. (2015). Integrating explicit knowledge in the
visual analytics process. Doctoral Consortium on
Computer Vision, Imaging and Computer Graphics
Theory and Applications (DCVISIGRAPP 2015), Sci-
tepress Digital Library.
Webster, J., Kingston, O., and Watson, R. T. (2002). Ana-
lyzing the past to prepare for the future: Writing a
literature review. MIS Quarterly, 26(2):xiii–xxiii.
Xinhua, E., Han, J., Wang, Y., and Liu, L. (2013). Big data-
as-a-service: Definition and architecture. In Commu-
nication Technology (ICCT), 2013 15th IEEE Interna-
tional Conference on, pages 738–742. IEEE.
Xu, D., Wu, D., Xu, X., Zhu, L., and Bass, L. (2015). Ma-
king real time data analytics available as a service. In
Proceedings of the 11th International ACM SIGSOFT
Conference on Quality of Software Architectures, pa-
ges 73–82. ACM.
Yu, L., Wang, S., and Lai, K. K. (2006). An integrated data
preparation scheme for neural network data analysis.
IEEE Transactions on Knowledge and Data Engineer-
ing, 18(2):217–230.
Zorrilla, M. and Garc
´
ıa-Saiz, D. (2013). A service orien-
ted architecture to provide data mining services for
non-expert data miners. Decision Support Systems,
55(1):399–411.
A Data-Science-as-a-Service Model
439