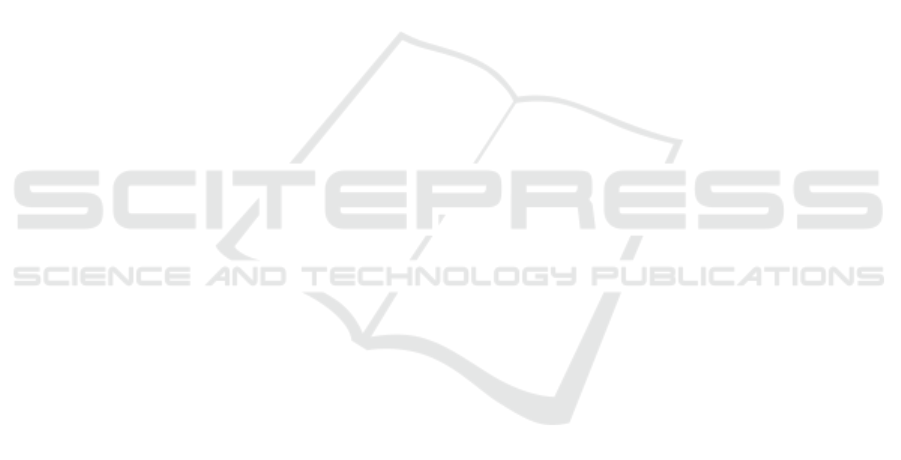
training and test processes the proposed method takes
is less than SRC takes, but more than CRC, NRS and
JCR take. In Table 2, the more accurate a method is,
the more computation time is generally required. This
demonstrates that accuracy comes at the cost of
increasing computational efforts. It is time
consuming to separately find out the most similar
training images for each test image and the most
frequent training images for every class with two
sub-dictionaries. The process occupies most of the
running time of the proposed method.
5 CONCLUSION
In this paper, experimental results clearly show that
the proposed method obtains the best classification
performance. It means the idea of training
dictionaries at two steps is promising, and encourages
me further to explore the direction. From Figure 4(a),
there still are many disturbances (for example,
estimated construction coefficients in class 8, 17 and
19). Effective methods for extracting discriminative
information of different classes should be explored to
decrease and even eliminate these disturbances.
Besides, time consuming on sub-dictionaries is also a
problem. To find out a way to reduce computing time
is necessary. Parallel computing can be thought as an
ideal direction in the future work.
REFERENCES
Chen, S., Tian, Y., 2015. Pyramid of spatial relatons for
scene-level land use classification. IEEE Transactions
on Geoscience and Remote Sensing 53, 1947-1957.
Dalal, N., Triggs, B., 2005. Histograms of oriented
gradients for human detection, Computer Vision and
Pattern Recognition, 2005. CVPR 2005. IEEE
Computer Society Conference on. IEEE, pp. 886-893.
Guo, J., Zhou, H., Zhu, C., 2013. Cascaded classification of
high resolution remote sensing images using multiple
contexts. Information Sciences 221, 84-97.
Hu, F., Xia, G.-S., Hu, J., Zhang, L., 2015. Transferring
deep convolutional neural networks for the scene
classification of high-resolution remote sensing
imagery. Remote Sensing 7, 14680-14707.
Huang, X., Lu, Q., Zhang, L., 2014. A multi-index learning
approach for classification of high-resolution remotely
sensed images over urban areas. ISPRS Journal of
Photogrammetry and Remote Sensing 90, 36-48.
Kobayashi, T., Otsu, N., 2008. Image feature extraction
using gradient local auto-correlations, European
conference on computer vision. Springer, pp. 346-358.
Li, W., Du, Q., 2014. Joint within-class collaborative
representation for hyperspectral image classification.
IEEE Journal of Selected Topics in Applied Earth
Observations and Remote Sensing 7, 2200-2208.
Li, W., Tramel, E.W., Prasad, S., Fowler, J.E., 2014.
Nearest regularized subspace for hyperspectral
classification. IEEE Transactions on Geoscience and
Remote Sensing 52, 477-489.
Mekhalfi, M.L., Melgani, F., Bazi, Y., Alajlan, N., 2015.
Land-use classification with compressive sensing
multifeature fusion. IEEE Geoscience and Remote
Sensing Letters 12, 2155-2159.
Nosaka, R., Ohkawa, Y., Fukui, K., 2011. Feature
extraction based on co-occurrence of adjacent local
binary patterns, Pacific-Rim Symposium on Image and
Video Technology. Springer, pp. 82-91.
Olshausen, B.A., Field, D.J., 1997. Sparse coding with an
overcomplete basis set: A strategy employed by V1?
Vision research 37, 3311-3325.
Wright, J., Yang, A.Y., Ganesh, A., Sastry, S.S., Ma, Y.,
2009. Robust face recognition via sparse
representation. IEEE transactions on pattern analysis
and machine intelligence 31, 210-227.
Yang, J., Yu, K., Gong, Y., Huang, T., 2009. Linear spatial
pyramid matching using sparse coding for image
classification, Computer Vision and Pattern
Recognition, 2009. CVPR 2009. IEEE Conference on.
IEEE, pp. 1794-1801.
Yang, Y., Newsam, S., 2010. Bag-of-visual-words and
spatial extensions for land-use classification,
Proceedings of the 18th SIGSPATIAL international
conference on advances in geographic information
systems. ACM, pp. 270-279.
Zhang, L., Yang, M., Feng, X., 2011. Sparse representation
or collaborative representation: Which helps face
recognition?, Computer vision (ICCV), 2011 IEEE
international conference on. IEEE, pp. 471-478.
Zhang, L., Yang, M., Feng, X., Ma, Y., Zhang, D., 2012.
Collaborative representation based classification for
face recognition. arXiv preprint arXiv:1204.2358.
Zhang, L., Zhang, J., Wang, S., Chen, J., 2015. Residential
area extraction based on saliency analysis for high
spatial resolution remote sensing images. Journal of
Visual Communication and Image Representation 33,
273-285.
Zhao, L.-J., Tang, P., Huo, L.-Z., 2014. Land-use scene
classification using a concentric circle-structured
multiscale bag-of-visual-words model. IEEE Journal of
Selected Topics in Applied Earth Observations and
Remote Sensing 7, 4620-4631.
Land-use Classification for High-resolution Remote Sensing Image using Collaborative Representation with a Locally Adaptive Dictionary
95