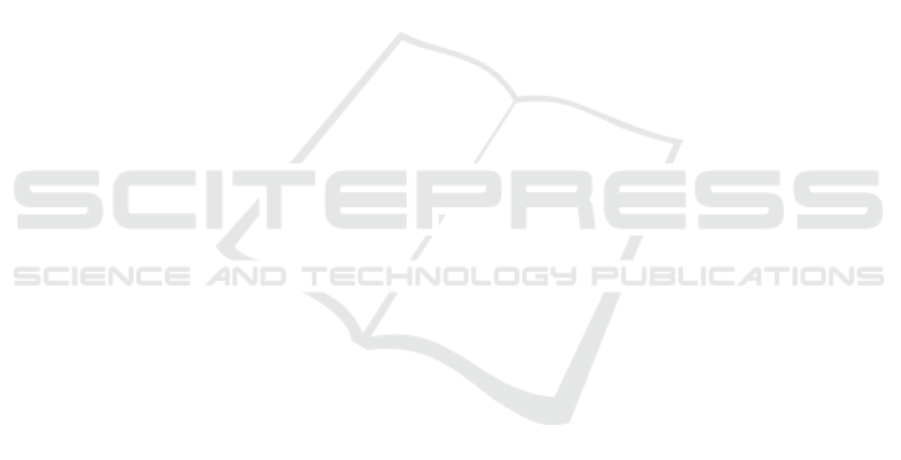
ing based on a coupled IMM-UKF-JPDA filter, which
allows a manoeuvre-aware multi-object tracking un-
der uncertainties in a cluttered environment. More-
over, geometric properties of the tracks are updated
in a post-processing part by means of computationally
low demanding rule-based filtering and the the use of
box frame history.
Finally, the framework is evaluated with the help of es-
tablished MOT16 metrics, which shows that the track-
ing performance is favourable in a variety of pre-
recorded real-world urban scenarios. Since the frame-
work is designed and found to run in real-time (under
100 ms), we expect that our framework is applicable
for autonomous vehicles. However, the performance
of this framework can be increased in future works by
further code optimisation, applying parallel program-
ming techniques and further fitting algorithm for V
and U shape traffic objects.
REFERENCES
Bar-Shalom, Y. and Li, X. R. (1995). Multitarget-
multisensor Tracking: Principles and Techniques.
Yaakov Bar-Shalom.
Bernardin, K. and Stiefelhagen, R. (2008). Evaluating mul-
tiple object tracking performance: The CLEAR MOT
metrics. Eurasip J. Image Video Process., 2008.
Blackman, S. (2004). Multiple hypothesis tracking for multi-
ple target tracking. IEEE Aerosp. Electron. Syst. Mag.,
19(1):5–18.
Chen, T., Dai, B., Liu, D., Fu, H., Song, J., and Wei, C. (2015).
Likelihood-Field-Model-Based Vehicle Pose Estima-
tion with Velodyne. IEEE Conf. Intell. Transp. Syst.
Proceedings, ITSC, 2015-Octob:296–302.
Choi, J., Ulbrich, S., Lichte, B., and Maurer, M. (2013).
Multi-Target Tracking using a 3D-Lidar sensor for au-
tonomous vehicles. IEEE Conf. Intell. Transp. Syst.
Proceedings, ITSC, (Itsc):881–886.
Djouadi, M., Sebbagh, A., and Berkani, D. (2005). IMM-
UKF algorithm and IMM-EKF algorithm for track-
ing highly maneuverable target: a comparison. Proc.
7th WSEAS Int. Conf. Autom. Control. Model. Simul.,
pages 283–288.
Freeman, H. and Shapira, R. (1975). Determining the
minimum-area encasing rectangle for an arbitrary
closed curve. Commun. ACM, 18(7):409–413.
Gao, L., Xing, J., Ma, Z., Sha, J., and Meng, X. (2012). Im-
proved IMM algorithm for nonlinear maneuvering tar-
get tracking. Procedia Eng., 29:4117–4123.
Geiger, a., Lenz, P., Stiller, C., and Urtasun, R. (2013). Vi-
sion meets robotics: The KITTI dataset. Int. J. Rob.
Res., 32(11):1231–1237.
Genovese, A. F. (2001). The interacting multiple model
algorithm for accurate state estimation of maneuvering
targets. Johns Hopkins APL Tech. Dig. (Applied Phys.
Lab., 22(4):614–623.
Himmelsbach, M., von Hundelshausen, F., and Wuensche,
H. (2010). Fast segmentation of 3D point clouds for
ground vehicles. Iv, pages 560–565.
Himmelsbach, M. and Wuensche, H. J. (2012). Tracking and
classification of arbitrary objects with bottom-up/top-
down detection. IEEE Intell. Veh. Symp. Proc., pages
577–582.
Kaestner, R., Maye, J., Pilat, Y., and Siegwart, R. (2012).
Generative object detection and tracking in 3D range
data. 2012 IEEE Int. Conf. Robot. Autom., pages 3075–
3081.
Leal-Taix
´
e, L., Milan, A., Reid, I., Roth, S., and Schindler,
K. (2017). MOT17 Results.
Levinson et al. (2011). Towards fully autonomous driving:
Systems and algorithms. IEEE Intell. Veh. Symp. Proc.,
(Iv):163–168.
Luo, Z., Habibi, S., and Mohrenschildt, M. (2016). Li-
DAR Based Real Time Multiple Vehicle Detection and
Tracking. 10(6):1083–1090.
Milan, A., Leal-Taixe, L., Reid, I., Roth, S., and Schindler,
K. (2016). MOT16: A Benchmark for Multi-Object
Tracking. pages 1–12.
Pfaltz, J. L. (1966). Sequential Operations in Digital Picture
Processing. J. ACM, 13(4):471–494.
Piao, S., Sutjaritvorakul, T., and Berns, K. (2016). Compact
Data Association in Multiple Object Tracking: Pedes-
trian Tracking on Mobile Vehicle as Case Study. 9th
IFAC Symp. Intell. Auton. Veh., 49(15):175–180.
Rubio, D. O., Lenskiy, A., and Ryu, J. H. (2013). Connected
components for a fast and robust 2D lidar data segmen-
tation. Proc. - Asia Model. Symp. 2013 7th Asia Int.
Conf. Math. Model. Comput. Simulation, AMS 2013,
(September 2015):160–165.
Schreier, M. (2017). Bayesian environment representation,
prediction, and criticality assessment for driver assis-
tance systems. PhD thesis.
Schreier, M., Willert, V., and Adamy, J. (2016a). Compact
Representation of Dynamic Driving Environments for
ADAS by Parametric Free Space and Dynamic Object
Maps. IEEE Trans. Intell. Transp. Syst., 17(2):367–
384.
Schreier, M., Willert, V., and Adamy, J. (2016b). Compact
Representation of Dynamic Driving Environments for
ADAS by Parametric Free Space and Dynamic Object
Maps. IEEE Trans. Intell. Transp. Syst., 17(2):367–
384.
Schueler, K., Weiherer, T., Bouzouraa, M. E., and Hofmann,
U. (2012). 360 Degree multi sensor fusion for static
and dynamic obstacles. 2012 IEEE Intell. Veh. Symp.,
pages 692–697.
Spinello, L., Luber, M., and Arras, K. O. (2011). Tracking
people in 3D using a bottom-up top-down detector.
Proc. - IEEE Int. Conf. Robot. Autom., pages 1304–
1310.
Velodyne (2007). Velodyne’s HDL-64E: A High Definition
Lidar Sensor for 3-D Applications. 2007. White Pap.,
page 7.
Wang, D. Z., Posner, I., and Newman, P. (2015). Model-
free detection and tracking of dynamic objects with
2D lidar. Int. J. Rob. Res., 34(7):1039–1063.
VEHITS 2018 - 4th International Conference on Vehicle Technology and Intelligent Transport Systems
166