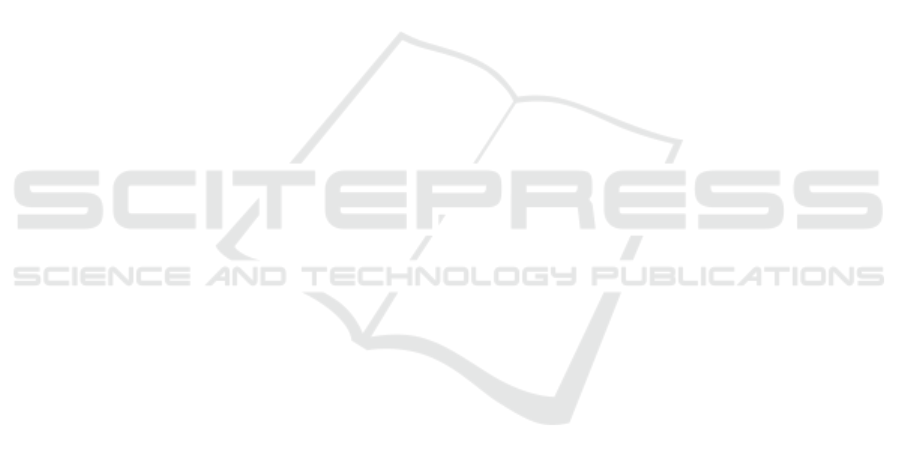
that edge is the best solution in this context. Then,
to validate our framework, we compare the average
response time, processing time and network delay ex-
perienced by the users of our approach with a related
work, where our solution showed a much better per-
formance. It is also clear that, promised benefits of
edge computing can only be achieved when effective
scheduling algorithms that consider its peculiar fea-
tures are implemented.
We believe our work will bring more research and
industry attention to this challenge and leverage the
adoption of edge computing. As future work, we plan
to enhance our algorithm by decentralizing the opti-
mization, adding another decision output for the num-
ber of instances of services, and taking vertical and
horizontal scaling into consideration.
ACKNOWLEDGEMENTS
This work has been supported by the Haley project
(Holistic Energy Efficient Hybrid Clouds) as part of
the TU Vienna Distinguished Young Scientist Award
2011, by the Rucon project (Runtime Control in Multi
Clouds), FWF Y 904 START-Programm 2015, and
by the Italian National Interuniversity Consortium for
Informatics (CINI)
REFERENCES
Aazam, M. and Huh, E.-N. (2015). Fog computing mi-
cro datacenter based dynamic resource estimation and
pricing model for iot. In Advanced Information Net-
working and Applications (AINA), 2015 IEEE 29th In-
ternational Conference on, pages 687–694. IEEE.
Aral, A. and Ovatman, T. (2016). Network-aware em-
bedding of virtual machine clusters onto federated
cloud infrastructure. Journal of Systems and Software,
120:89–104.
Bilal, K. and Erbad, A. (2017). Edge computing for inter-
active media and video streaming. In Fog and Mobile
Edge Computing (FMEC), 2017 Second International
Conference on, pages 68–73. IEEE.
Calheiros, R. N., Ranjan, R., Beloglazov, A., De Rose,
C. A., and Buyya, R. (2011). Cloudsim: a toolkit for
modeling and simulation of cloud computing environ-
ments and evaluation of resource provisioning algo-
rithms. Software: Practice and experience, 41(1).
Dobrian, F., Sekar, V., Awan, A., Stoica, I., Joseph, D., Gan-
jam, A., Zhan, J., and Zhang, H. (2011). Understand-
ing the impact of video quality on user engagement. In
ACM SIGCOMM Computer Communication Review,
volume 41, pages 362–373. ACM.
Duong, T. N. B., Li, X., Goh, R. S. M., Tang, X., and
Cai, W. (2012). Qos-aware revenue-cost optimization
for latency-sensitive services in iaas clouds. In Dis-
tributed Simulation and Real Time Applications (DS-
RT), 2012 IEEE/ACM 16th International Symposium
on, pages 11–18. IEEE.
Guo, X., Singh, R., Zhao, T., and Niu, Z. (2016). An index
based task assignment policy for achieving optimal
power-delay tradeoff in edge cloud systems. In Com-
munications (ICC), 2016 IEEE International Confer-
ence on, pages 1–7. IEEE.
Hu, Y. C., Patel, M., Sabella, D., Sprecher, N., and Young,
V. (2015). Mobile edge computing—a key technology
towards 5g. ETSI White Paper, 11(11):1–16.
Mao, Y., Zhang, J., and Letaief, K. B. (2016). Dynamic
computation offloading for mobile-edge computing
with energy harvesting devices. IEEE Journal on Se-
lected Areas in Communications, 34(12):3590–3605.
Papagianni, C., Leivadeas, A., Papavassiliou, S., Maglaris,
V., Cervello-Pastor, C., and Monje, A. (2013). On the
optimal allocation of virtual resources in cloud com-
puting networks. IEEE Transactions on Computers,
62(6):1060–1071.
Pathan, A.-M. K. and Buyya, R. (2007). A taxonomy and
survey of content delivery networks. Grid Comput-
ing and Distributed Systems Laboratory, University of
Melbourne, Technical Report, 4.
Piao, J. T. and Yan, J. (2010). A network-aware vir-
tual machine placement and migration approach in
cloud computing. In Grid and Cooperative Com-
puting (GCC), 2010 9th International Conference on,
pages 87–92. IEEE.
Shi, W., Cao, J., Zhang, Q., Li, Y., and Xu, L. (2016). Edge
computing: Vision and challenges. IEEE Internet of
Things Journal, 3(5):637–646.
Skarlat, O., Nardelli, M., Schulte, S., and Dustdar, S.
(2017). Towards qos-aware fog service placement. In
Fog and Edge Computing (ICFEC), 2017 IEEE 1st In-
ternational Conference on, pages 89–96. IEEE.
Sonmez, C., Ozgovde, A., and Ersoy, C. (2017). Edge-
cloudsim: An environment for performance evalua-
tion of edge computing systems. In Fog and Mobile
Edge Computing (FMEC), 2017 Second International
Conference on, pages 39–44. IEEE.
Wang, S., Zhang, X., Zhang, Y., Wang, L., Yang, J., and
Wang, W. (2017). A survey on mobile edge networks:
Convergence of computing, caching and communica-
tions. IEEE Access, 5:6757–6779.
Yu, C., Lumezanu, C., Sharma, A., Xu, Q., Jiang, G., and
Madhyastha, H. V. (2015). Software-defined latency
monitoring in data center networks. In International
Conference on Passive and Active Network Measure-
ment, pages 360–372. Springer.
Zeng, L., Veeravalli, B., and Wei, Q. (2014). Space4time:
Optimization latency-sensitive content service in
cloud. Journal of Network and Computer Applica-
tions, 41:358–368.
Zhao, T., Zhou, S., Guo, X., Zhao, Y., and Niu, Z. (2015). A
cooperative scheduling scheme of local cloud and in-
ternet cloud for delay-aware mobile cloud computing.
In Globecom Workshops (GC Wkshps), 2015 IEEE,
pages 1–6. IEEE.
CLOSER 2018 - 8th International Conference on Cloud Computing and Services Science
168