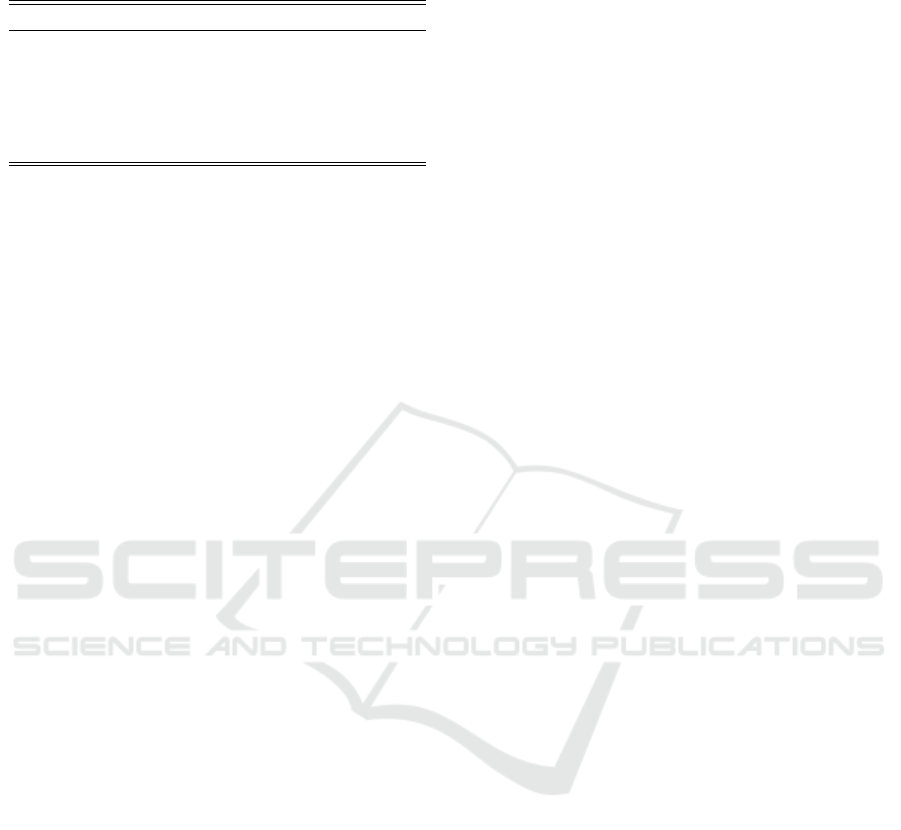
Table 6: Summary results for the numeric attributes of the
BPI 2017 dataset.
Statistics Credit Score Nb. Offer R. Amount
Min. 0.000 1.000 0.00
1st Qu. 0.000 1.000 6000.00
Median 0.000 1.000 12500.00
Mean 436.100 1.363 16210.00
3rd Qu. 901.000 2.000 21000.00
Max. 1145.000 10.000 450000.00
in the process discovery field (Verbeek et al., 2017).
We are still making improvements to our pro-
totype based on interesting demands that should help
to understand the process and to filter cases. As fu-
ture work, we want to incorporate our tool as a ProM
plug-in. We also intend to include Local Affine Mul-
tidimensional Projection (LAMP) (Joia et al., 2011;
Pagliosa et al., 2015) in our prototype, which will
enable us to set a group of control points and dyn-
amically project new instances. Another interesting
idea is to incorporate attribute-level linkage, similar
to the analysis we performed in Section 5, to the pro-
totype itself. This way, an analyst may quickly dis-
cover which attributes contribute to the dataset varia-
bility and, thus, fine-tune the attribute weights for the
dissimilarity metric.
ACKNOWLEDGEMENTS
We thank Conselho Nacional de Desenvolvimento
Cient
´
ıfico e Tecnol
´
ogico (CNPq) and Coordenac¸
˜
ao de
Aperfeic¸oamento de Pessoal de N
´
ıvel Superior (CA-
PES) for partially financing this research.
REFERENCES
De Koninck, P. and De Weerdt, J. (2016). Multi-objective
trace clustering: finding more balanced solutions. In
International Conference on Business Process Mana-
gement, pages 49–60. Springer.
Hunter, J. D. (2007). Matplotlib: A 2d graphics environ-
ment. Computing In Science & Engineering, 9(3):90–
95.
Jaccard, P. (1901).
´
Etude comparative de la distribution flo-
rale dans une portion des alpes et des jura. Bull Soc
Vaudoise Sci Nat, 37:547–579.
Joia, P., Coimbra, D., Cuminato, J. A., Paulovich, F. V., and
Nonato, L. G. (2011). Local affine multidimensional
projection. IEEE Transactions on Visualization and
Computer Graphics, 17(12):2563–2571.
Kaufman, L. and Rousseeuw, P. J. (2009). Finding groups
in data: an introduction to cluster analysis, volume
344. John Wiley & Sons.
Kruskal, J. B. and Wish, M. (1978). Multidimensional Sca-
ling, volume 31.
Levenshtein, V. I. (1966). Binary codes capable of cor-
recting deletions, insertions, and reversals. In Soviet
physics doklady, volume 10, pages 707–710.
Lopes, H. and Barbosa, S. (2015). Learning and Inferring:
Festschrift for Alejandro Frery, chapter Uncertainty
Measures and the concentration of probability density
functions. College Publications.
Low, W. Z., Van der Aalst, W. M., ter Hofstede, A. H.,
Wynn, M. T., and De Weerdt, J. (2017). Change vi-
sualisation: Analysing the resource and timing diffe-
rences between two event logs. Information Systems,
65:106–123.
Maaten, L. v. d. and Hinton, G. (2008). Visualizing data
using t-sne. Journal of Machine Learning Research,
9(Nov):2579–2605.
Mendling, J., Baesens, B., Bernstein, A., and Fellmann, M.
(2017). Challenges of smart business process mana-
gement: An introduction to the special issue.
Mining, P. (2014). Automated process discovery soft-
ware for professionals-fluxicon disco. Adres:
http://fluxicon. com/disco/Eris¸im Tarihi, 11.
Mises, R. and Pollaczek-Geiringer, H. (1929). Praktische
verfahren der gleichungsaufl
¨
osung. ZAMM-Journal
of Applied Mathematics and Mechanics/Zeitschrift f
¨
ur
Angewandte Mathematik und Mechanik, 9(1):58–77.
Pagliosa, P., Paulovich, F. V., Minghim, R., Levkowitz, H.,
and Nonato, L. G. (2015). Projection inspector: As-
sessment and synthesis of multidimensional projecti-
ons. Neurocomputing, 150:599–610.
Pedregosa, F., Varoquaux, G., Gramfort, A., Michel, V.,
Thirion, B., Grisel, O., Blondel, M., Prettenhofer, P.,
Weiss, R., Dubourg, V., et al. (2011). Scikit-learn:
Machine learning in python. Journal of Machine Le-
arning Research, 12(Oct):2825–2830.
Rodrigues, A. M. B., Almeida, C. F. P., Saraiva, D. D. G.,
Moreira, F. B., Spyrides, G. M., Varela, G., Krieger,
G. M., Peres, I. T., Dantas, L. F., Lana, M., Alves,
O. E., Franca, R., Neira, R. A. Q., Gonzalez, S. F.,
Fernandes, W. P. D., Barbosa, S. D. J., Poggi, M., and
Lopes, H. (2017). Stairway to value: mining a loan
application process. Technical report, Pontif
´
ıcia Uni-
versidade Cat
´
olica do Rio de Janeiro.
Rousseeuw, P. J. (1987). Silhouettes: a graphical aid to
the interpretation and validation of cluster analysis.
Journal of computational and applied mathematics,
20:53–65.
Shannon, C. E. and Weaver, W. (1963). A Mathemati-
cal Theory of Communication. University of Illinois
Press, Champaign, IL, USA.
Strehl, A. and Ghosh, J. (2002). Cluster ensembles—
a knowledge reuse framework for combining multi-
ple partitions. Journal of machine learning research,
3(Dec):583–617.
Tiwari, A., Turner, C. J., and Majeed, B. (2008). A re-
view of business process mining: state-of-the-art and
future trends. Business Process Management Journal,
14(1):5–22.
ICEIS 2018 - 20th International Conference on Enterprise Information Systems
48