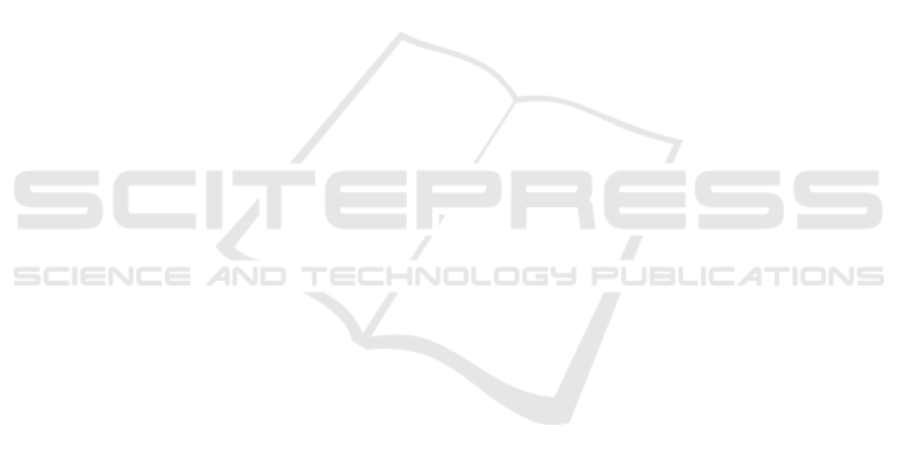
We have compared the performance of several classi-
fiers. The best results were achieved using GB classi-
fier w = 60,r = 0.75 and DW = 15 (DW Duration of
285s) which yields ROC AUC of 98.7%. Our results
show that even with poorly annotated data and only
use of vehicle sensor data it is possible to accurately
detect distracted driving events. In future work intra-
subject models should be evaluated. It will be also of
interest to see how the proposed mechanism performs
on a more granular dataset, with more accurate labels.
ACKNOWLEDGEMENTS
The authors would like to thank Dr. H
¨
useyn Abut
and VPALAB of Sabanci University for providing the
data-set used for this study.
REFERENCES
Abut, H., Erdo
˘
gan, H., Erc¸il, A., C¸
¨
ur
¨
ukl
¨
u, A. B., Koman,
H. C., Tas, F., Arguns¸ah, A.
¨
O., Akan, B., Karabalkan,
H., C¸
¨
okelek, E., et al. (2007). Data collection with”
uyanik”: too much pain; but gains are coming.
Berka, C., Levendowski, D. J., Lumicao, M. N., Yau,
A., Davis, G., Zivkovic, V. T., Olmstead, R. E.,
Tremoulet, P. D., and Craven, P. L. (2007). EEG Cor-
relates of Task Engagement and Mental Workload in
Vigilance, Learning, and Memory Tasks.
Dietterich, T. G. (2002). Machine learning for sequential
data: A review. In Joint IAPR International Work-
shops on Statistical Techniques in Pattern Recognition
(SPR) and Structural and Syntactic Pattern Recogni-
tion (SSPR), pages 15–30. Springer.
Dong, Y., Hu, Z., Uchimura, K., and Murayama, N. (2011).
Driver inattention monitoring system for intelligent
vehicles: A review. IEEE Transactions on Intelligent
Transportation Systems, 12(2):596–614.
Engstr
¨
om, J., Monk, C. A., Hanowski, R. J., Horrey, W. J.,
Lee, J. D., McGehee, D. V., Regan, M. a., Stevens, A.,
Traube, E., Tuukkanen, M., Victor, T., and Yang, D.
(2013). A Conceptual Framework and Taxonomy for
Understanding and Categorizing Driver Inattention.
Farneb
¨
ack, G. (2003). Two-Frame Motion Estimation
Based on Polynomial Expansion. Springer.
Fridman, L., Brown, D. E., Angell, W., Abdi
´
c, I., Reimer,
B., and Noh, H. Y. (2016). Automated synchro-
nization of driving data using vibration and steering
events. Pattern Recogn. Lett., 75(C):9–15.
Harbluk, J. L., Noy, Y. I., Trbovich, P. L., and Eizenman, M.
(2007). An on-road assessment of cognitive distrac-
tion: Impacts on drivers’ visual behavior and brak-
ing performance. Accident Analysis and Prevention,
39(2):372–379.
Jafarnejad, S., Castignani, G., and Engel, T. (2017). To-
wards a real-time driver identification mechanism
based on driving sensing data. In 20th Interna-
tional Conference on Intelligent Transportation Sys-
tems (ITSC). IEEE.
Jin, L., Niu, Q., Hou, H., Xian, H., Wang, Y., and Shi, D.
(2012). Driver cognitive distraction detection using
driving performance measures. Discrete Dynamics in
Nature and Society.
Klauer, S. G., Guo, F., Sudweeks, J., and Dingus, T. A.
(2010). An analysis of driver inattention using a case-
crossover approach on 100-car data. Technical report.
Lee, J., Young, K., and Regan, M. (2009). Defining driver
distraction, pages 31 – 40. CRC Press, Australia.
Liang, Y. and Lee, J. D. (2010). Combining cognitive and
visual distraction: Less than the sum of its parts. Ac-
cident Analysis and Prevention, 42(3):881–890.
Miyajima, C., Kusakawa, T., Nishino, T., Kitaoka, N., Itou,
K., and Takeda, K. (2009). On-going data collection
of driving behavior signals. In In-Vehicle Corpus and
Signal Processing for Driver Behavior. Springer.
¨
Ozt
¨
urk, E. and Erzin, E. (2012). Driver Status Identification
from Driving Behavior Signals, pages 31–55. Springer
NY, New York, NY.
Pedregosa, F., Varoquaux, G., Gramfort, A., Michel, V.,
Thirion, B., Grisel, O., Blondel, M., Prettenhofer,
P., Weiss, R., Dubourg, V., Vanderplas, J., Passos,
A., Cournapeau, D., Brucher, M., Perrot, M., and
Duchesnay, E. (2011). Scikit-learn: Machine learning
in Python. Journal of Machine Learning Research,
12:2825–2830.
Rantanen, E. M. and Goldberg, J. H. (1999). The effect
of mental workload on the visual field size and shape.
Ergonomics, 42(6):816–834.
Savitzky, A. Golay, M. J. E. (1964). Smoothing and dif-
ferentiation of data by simplified least squares proce-
dures. Anal. Chem., 36.
Tango, F. and Botta, M. (2013). Real-time detection system
of driver distraction using machine learning. IEEE
Transactions on Intelligent Transportation Systems,
14(2):894–905.
Wesley, A., Hoffman Hall, P. G., Shastri, D., and Pavlidis,
I. (2010). A Novel Method to Monitor Driver’s Dis-
tractions CHI 2010: Work-in-Progress.
W
¨
ollmer, M., Blaschke, C., Schindl, T., Schuller, B.,
F
¨
arber, B., Mayer, S., and Trefflich, B. (2011). Online
Driver Distraction Detection Using Long Short-Term
Memory. IEEE Transactions on Intelligent Trans-
portation Systems, 12(2).
Zhou, H., Itoh, M., and Inagaki, T. (2008). Influence of cog-
nitively distracting activity on driver’s eye movement
during preparation of changing lanes. Proceedings of
the SICE Annual Conference, pages 866–871.
VEHITS 2018 - 4th International Conference on Vehicle Technology and Intelligent Transport Systems
186