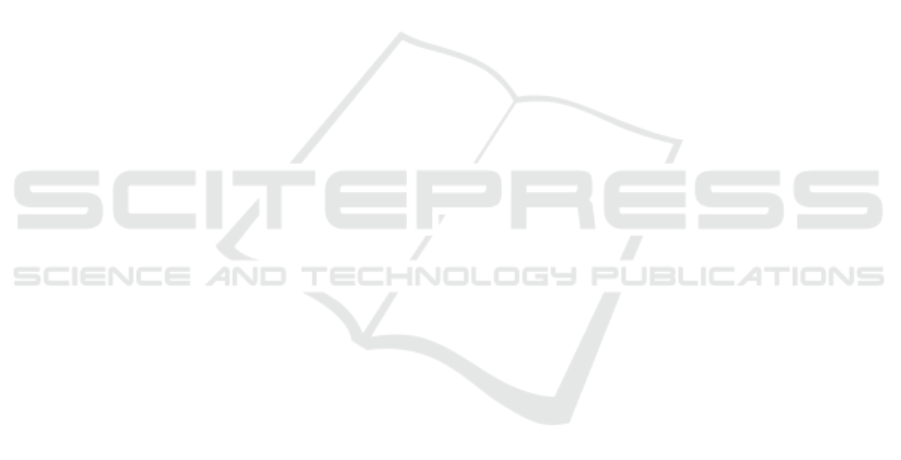
ACKNOWLEDGEMENTS
We thank National Council for Scientific and Techno-
logical Development (CNPq), National Council for
the Improvement of Higher Education (CAPES) and
Sao Paulo Research Foundation (FAPESP) for finan-
cial support.
REFERENCES
Amaral, B. F. d., Gonc¸alves, R., Romani, L., Sousa, E. P.
M. d., et al. (2014). Improving the semi-supervised
classification of time series extracted from satellite
images (in portuguese: Aprimorando a classificac¸
˜
ao
semissupervisionada de s
´
eries temporais extra
´
ıdas de
imagens de sat
´
elite). In Symposium on Knowledge
Discovery, Mining and Learning, 2th. Sociedade Bra-
sileira de Computac¸
˜
ao-SBC, KDD.
Bank, W. (2017). Databank - brazil. The World Bank
(IBRD - IDA) - https://data.worldbank.org/country/
brazil?locale=pt. Accessed: 2017-09-15.
Boughrara, H., Chtourou, M., and Amar, C. B. (2017). MLP
neural network using constructive training algorithm:
application to face recognition and facial expression
recognition. IJISTA, 16(1):53–79.
Brasil, G. (2016). Agribusiness may increase of 2% in 2017
(in portuguese: Agroneg
´
ocio deve ter crescimento de
2% em 2017). http://www.wpcentral.com/ie9-
windows-phone-7-adobe-flash-demos-and-
development-videos. Accessed: 2017-09-13.
Chen, T. and He, T. (2015). Xgboost: extreme gradient
boosting. R package version 0.4-2.
Cover, T. and Hart, P. (1967). Nearest neighbor pattern clas-
sification. IEEE transactions on information theory,
13(1):21–27.
da Silva, W. L., Gonc¸alves, R. R. V., Siqueira, A. S., Zullo,
J., and Neto, F. A. M. G. (2011). Feature extraction
for ndvi avhrr/noaa time series classification. In 2011
6th International Workshop on the Analysis of Multi-
temporal Remote Sensing Images (Multi-Temp), pages
233–236.
do Valle Gonc¸alves, R. R., Zullo, J., Romani, L. A. S.,
do Amaral, B. F., and Sousa, E. P. M. (2017). Agri-
cultural monitoring using clustering techniques on sa-
tellite image time series of low spatial resolution. In
2017 9th International Workshop on the Analysis of
Multitemporal Remote Sensing Images (MultiTemp),
pages 1–4, Brugge, Belgium.
Esling, P. and Agon, C. (2012). Time-series data mining.
ACM Computing Surveys (CSUR).
Jo
˜
ao, R. S., Mpinda, S. T. A., Vieira, A. P. B., Jo
˜
ao, R. S.,
Romani, L. A. S., and Ribeiro, M. X. (2018). A New
Approach to Classify Sugarcane Fields Based on As-
sociation Rules, pages 475–483. Springer Internatio-
nal Publishing, Cham.
Julea, A., Meger, N., Bolon, P., Rigotti, C., Doin, M. P.,
Lasserre, C., Trouve, E., and Lazarescu, V. N.
(2011). Unsupervised spatiotemporal mining of satel-
lite image time series using grouped frequent sequen-
tial patterns. IEEE Transactions on Geoscience and
Remote Sensing, 49(4):1417–1430.
Kim, S., Park, S., and Chu, W. W. (2001). An index-
based approach for similarity search supporting time
warping in large sequence databases. In Data Engi-
neering, 2001. Proceedings. 17th International Confe-
rence on, pages 607–614, Heidelberg, Germany, Ger-
many. International Conference on Data Engineering
(IEEE).
Kyrgyzov, I. O., Maitre, H., and Campedel, M. (2007). A
method of clustering combination applied to satellite
image analysis. In 14th International Conference on
Image Analysis and Processing (ICIAP 2007), pages
81–86.
Larsen, K. (2005). Generalized naive bayes classifiers.
SIGKDD Explorations, 7(1):76–81.
Maimon, O. and Rokach, L. (2005). The Data Mining and
Knowledge Discovery Handbook. Springer.
Mao, R., Zhang, P., Li, X., Liu, X., and Lu, M. (2016).
Pivot selection for metric-space indexing. Internati-
onal Journal of Machine Learning and Cybernetics,
7(2):311–323.
Matthews, B. W. (1975). Comparison of the predicted and
observed secondary structure of t4 phage lysozyme.
Biochimica et Biophysica Acta (BBA)-Protein Struc-
ture, 405(2):442–451.
Mitsa, T. (2010). Temporal data mining. CRC Press.
Price, J. C. (1993). Estimating leaf area index from satellite
data. IEEE Transactions on Geoscience and Remote
Sensing, 31(3):727–734.
Ratanamahatana, C. A. and Keogh, E. (2004). Everything
you know about dynamic time warping is wrong. In
Third Workshop on Mining Temporal and Sequential
Data, pages 22–25. Citeseer.
Romani, L. A. S., Gonc¸alves, R. R. V., Amaral, B. F., Chino,
D. Y. T., Zullo, J., Traina, C., Sousa, E. P. M., and
Traina, A. J. M. (2011). Clustering analysis applied to
ndvi/noaa multitemporal images to improve the moni-
toring process of sugarcane crops. In 2011 6th Inter-
national Workshop on the Analysis of Multi-temporal
Remote Sensing Images (Multi-Temp), pages 33–36.
Scrivani, R., Zullo, J., and Romani, L. A. S. (2017). Sits
for estimating sugarcane production. In 2017 9th In-
ternational Workshop on the Analysis of Multitempo-
ral Remote Sensing Images (MultiTemp), pages 1–4,
Brugge, Belgium.
Service, F. A. (2017). Sugar: World markets and
trade. United States Department of Agriculture -
https://apps.fas.usda.gov/psdonline/circulars/sugar.pdf.
Accessed: 2017-10-10.
Tanha, J., van Someren, M., and Afsarmanesh, H. (2017).
Semi-supervised self-training for decision tree clas-
sifiers. Int. J. Machine Learning & Cybernetics,
8(1):355–370.
Classification Analysis of NDVI Time Series in Metric Spaces for Sugarcane Identification
169