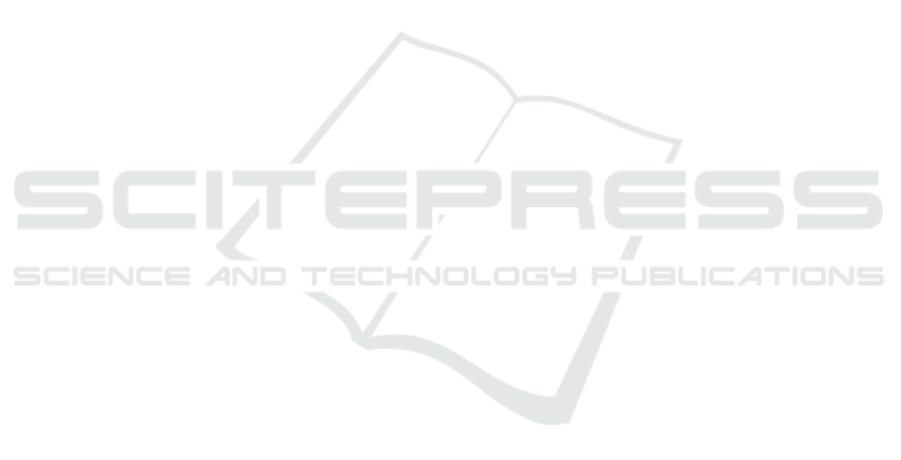
Given the traffic congestion and pollution, it is imper-
ative to explore ways in which commutes using pri-
vate vehicles can be reduced. A sizeable segment of
the citizenry would take to buses if their quality of
service could be improved. Large schedule variance
and unpredictable arrival times have had a negative
impact on commuters seeking predictable transits. In
2016, BMTC, which is Bengaluru's public bus trans-
port provider, invested in an ITS platform to capture
large volumes of AVL and ETM data generated by its
fleet of 6,400 buses. The paper throws light on how
this data is stored and processed in a cluster of ma-
chines that are enabled by big data technologies such
as Spark/Hadoop/Python/R.
For our study, we select a popular route (356R) of
BMTC, and examine Trip 3 on the schedule, which
operates during the peak hours of the morning. By
querying the AVL and ETM data, we ascertain run
times for this trip over a 4 month period. Our analysis
exposes a problem of schedule variance. To suggest
remedies to this problem, we undertake a time series
analysis of trip run times, and finalise on a seasonal
ARIMA model.
Weekly forecasts based on our time series analy-
sis can be of utility to schedule planners. By piecing
together the forecasts for different trips, planners will
be able to design schedules for better compliance by
drivers, who are rushing to complete trips.
Our next mission would be to help planners ratio-
nalise the 6,100 schedules maintained by BMTC; this
will requires strong computational support. Revised
schedules based on credible forecast numbers have
the potential to remedy some of the problems faced
by transit operators. Efforts are underway to display
the expected time of arrival (ETA) of different buses
at a bus stop; this step is predicted to have a significant
positive impact on the ridership numbers.
Besides consuming time and resources, there is a
risk of committing mistakes while reconstructing the
trip information from two disparate sources, namely
the VTU and ETM. This problem may be addressed
by a simple mechanism onboard the bus, such as a
mobile listening device, that rolls up this data in real
time, and relays the combination of device ID, sched-
ule, trip number, geo-location and timestamp to the
ITS. For this to work, the VTU and ETM may have to
be suitably modified to communicate with the device
using a short-range protocol such as Bluetooth.
ACKNOWLEDGEMENTS
The authors owe their gratitude to senior BMTC offi-
cials Dr.Ekroop Caur (MD) and Mr.Bishwajit Mishra
(Director, IT). We have received substantial technical
assistance from Dibya Ranjan Jena of Trimax IT, and
are deeply thankful for the same. The research is sup-
ported by a generous grant from the Indian Institute of
Management Bangalore (IIMB). The Data Centre and
Analytics Lab (DCAL) at IIMB houses the Hadoop
cluster that has been critical to drive computations and
results.
REFERENCES
Badami, M. G. and Haider, M. (2007). An analysis
of public bus transit performance in indian cities.
Transportation Research Part A: Policy and Practice,
41(10):961–981.
Furth, P. G., Hemily, B., Muller, T., and Strathman, J. G.
(2003). Uses of archived avl-apc data to improve tran-
sit performance and management: Review and poten-
tial. TCRP Web Document, 23.
Hanumappa, D., Ramachandran, P., Sitharam, T., Lak-
shamana, S., and Mulangi, R. H. (2016). Perfor-
mance evaluation of premium services in bangalore
metropolitan transport corporation (bmtc). Trans-
portation Research Procedia, 17:184–192.
Hyndman, R. J. and Athanasopoulos, G. (2014). Forecast-
ing: principles and practice. OTexts.
T
´
etreault, P. R. and El-Geneidy, A. M. (2010). Estimat-
ing bus run times for new limited-stop service using
archived avl and apc data. Transportation Research
Part A: Policy and Practice, 44(6):390–402.
Vanajakshi, L., Subramanian, S. C., and Sivanandan, R.
(2009). Travel time prediction under heterogeneous
traffic conditions using global positioning system data
from buses. IET intelligent transport systems, 3(1):1–
9.
VEHITS 2018 - 4th International Conference on Vehicle Technology and Intelligent Transport Systems
410