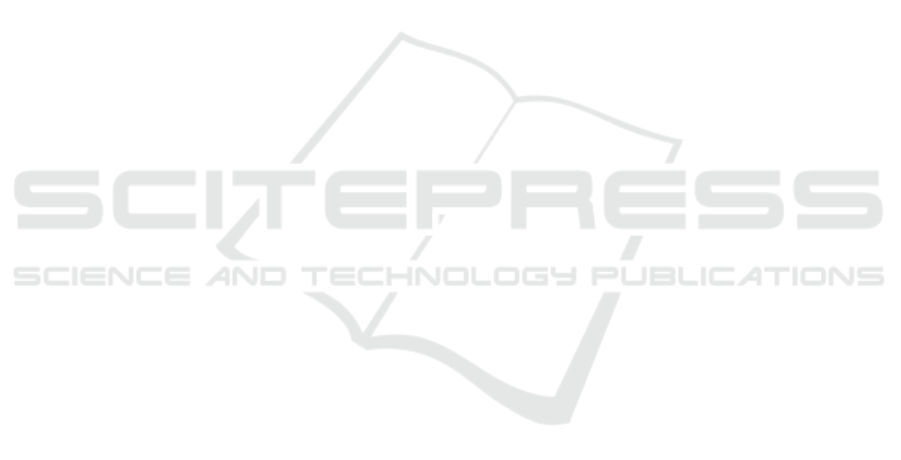
Hierarchical Electricity Demand Forecasting by Exploring the
Electricity Consumption Patterns
Yue Pang
1
, Chaoyi Jin
1
, Xiangdong Zhou
1
, Naiwang Guo
2
and Yong Zhang
2
1
School of Computer Science, Fudan University, Shanghai, China
2
State Grid Shanghai Municipal Electric Power Company, Shanghai, China
Keywords: Hierarchical Forecasting, Aggregate Constraints, Consumption Pattern.
Abstract: Accurate electricity demand forecasting is necessary to develop an efficient and sustainable power system.
Total demand of the whole region can be disaggregated at different levels, thus producing a hierarchical
structure. In the hierarchical demand forecasting, the prediction accuracy and aggregate consistency between
levels are two important issues, however in the previous works the prediction accuracy is often affected by
conducting the aggregate consistency. In this work, we propose a novel pattern-based hierarchical time series
forecasting (PHF) method which consists of two aggregation stages. In the first aggregation stage, by
exploring the electricity consuming patterns with clustering method, the bottom level electricity demand
forecasting is improved, and in the second stage the region level aggregation is conducted to achieve the
whole level forecasting. The experiments are conducted on the Energy Demand Research Project (EDRP)
datasets, and the experimental results show that compared with the previous state-of-the-art methods, our
method improves the prediction accuracy in all hierarchical levels with keeping aggregation consistency.
1 INTRODUCTION
Due to the existing problem of inconvenience
electricity storage, excess electricity would cause
unnecessary waste. Accurate forecasting is helpful to
guide the electric power companies to make decision.
Thus, electricity demand forecasting is one of the
most important problems in the field of electric power
management. With the rapid growth of smart grid,
more and more meter data are becoming available,
which brings potential of improving the prediction of
the power demand with more delicacy.
Recently, hierarchical electricity demand
forecasting attracts more and more research attentions
(Taieb et al., 2017). Total consumption in the whole
geographic region can be geographically
disaggregated into several sub-regions, and these sub-
regions can be further disaggregated into regions at
lower level. For example, electricity consumption in
countries can be disaggregated into provinces, cities,
districts, etc. That is, electricity time series can be
represented in a hierarchical structure. From top
down, the structure contains series at top level, high
level, low level and bottom level. According to the
above geographic disaggregate strategy, the time
series in different levels must obey the aggregation
constraints, i.e. the demand in different levels should
be summed consistently. Most of the state-of-the-art
hierarchical predication methods estimate initial
forecasts and then reconcile them to ensure aggregate
constraints. However, it is noticed that the regional
aggregation consistency cannot improve the whole
level prediction accuracy (Hyndman et al., 2011).
The electricity consuming pattern can be found by
clustering analysis on the time series of electricity
usage with the similarity measurements. Figure 1
illustrates the aggregation time series of electricity
consumption by clustering and random selection. We
notice that in this Figure the aggregated time series
obtained by clustering of similar time series shows
more stable and regular than the series aggregated by
randomly selected ones. The experience and some
previous work (Wijaya et al., 2015) show that the
stable and regular time series are very good (or ideal)
for prediction or regression. Therefore, we are
motivated to exploit the time series clustering to
improve the bottom level electricity demand
prediction and manage to improve the regional
hierarchal predication accuracy.
In this paper we propose a novel pattern-based
hierarchical demand forecasting (PHF) method which
consists of two aggregation stages. The proposed
576
Pang, Y., Jin, C., Zhou, X., Guo, N. and Zhang, Y.
Hierarchical Electricity Demand Forecasting by Exploring the Electricity Consumption Patterns.
DOI: 10.5220/0006715005760581
In Proceedings of the 7th International Conference on Pattern Recognition Applications and Methods (ICPRAM 2018), pages 576-581
ISBN: 978-989-758-276-9
Copyright © 2018 by SCITEPRESS – Science and Technology Publications, Lda. All rights reserved