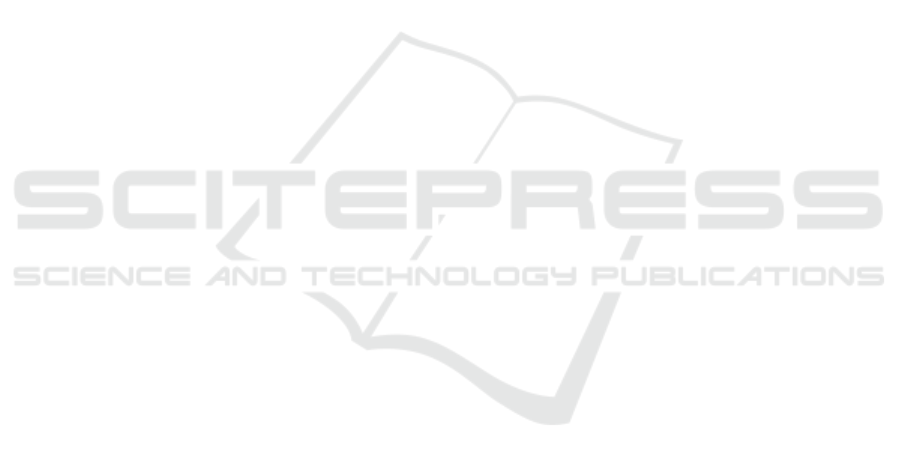
consumers. By tuning the sparsity parameter
properly, sparse PCs derived can indicate the
significant time intervals in a day (that is, peak times),
so as to divide a day into different times
automatically.
Additionally, to study the relationships between
social background and electricity consumption model
of consumers, we use ACORN (CACI, 2010) to
categorize consumers and apply SPCA to analyse
electricity consumption model for each category. The
ACORN provides precise information and an in-
depth understanding of different types of people (that
is, social background of these people). Based on the
experiments, we find that social background of
consumers influences the consumption model but
cannot fully determine it. That reveals that it is
insufficient to consider only social background of
consumers, when adjusting policies.
2 RELATED WORK
Data analysis of daily load data generated by smart
meters can benefit both electricity suppliers and end
consumers. A two-stage clustering based on multi-
level 1D discrete wavelet transform and K-means
algorithm is applied to perform daily load curve
clustering and load pattern clustering (Zigui et al.,
2017). Additionally, to obtain distinct consumer
categories, method of category identification based
on association rule mining and characteristic
similarity is also proposed in the paper. Zigui et al.
study the relationships between the natural types of
consumers and the consumer categorization based on
load pattern similarity; they find that the types cannot
full determine the categorization.
A hybrid fuzzy-stochastic technique proposed by
Yu et al., (2017) develops an interval-fuzzy chance-
constrained programming (IFCCP) method to reflect
multiple uncertainties expressed as interval-fuzzy-
random (integration of interval values, fuzzy sets, and
probability distributions). IFCCP has advantages in
uncertainty reflection and policy analysis, while
avoiding complicated intermediate models with high
computational efficiency. Considering the peak
power demand problem, the developed IFCCP
method is used to plan a regional-scale electric power
system (EPS).
In contrast to dividing a day manually relying on
experience, segmenting automatically by our SPCA
provides more convenience and rationality, since the
peak times and off-peak times derived are consistent
with the real consuming habits of consumers.
3 ANALYSING ELECTRICITY
CONSUMPTION MODEL
The process of electricity consumption model
analysis consists of two stages. First, we impose
clustering to divide consumers into groups according
to their consumption model, and meanwhile,
categorize consumers by their social background for
further study. Then, SPCA is employed to analyse
electricity consumption model for each group.
3.1 Clustering and Categorization
To obtain a better understanding of electricity
consumption model, we impose K-Means clustering
and AP clustering to gather consumers with similar
consumption model. K-Means clustering is a method
of vector quantization, originating from signal
processing, and is popular for cluster analysis in data
mining. It aims to partition n observations into k
clusters where each observation belongs to the cluster
with the nearest centroid. Affinity propagation (AP)
clustering is an algorithm based on the concept of
"message passing" between data points. Unlike K-
Means, Affinity Propagation does not require the
number of clusters to be determined before clustering.
Let denote a data matrix consisting of
electricity time series of consumers. Herein, is the
number of consumers, is the number of time
intervals, and
is the electricity consumption of
the th consumer in the th time interval. Clustering
is applied to divide data matrix into K sub-matrices
in the form of
, where
. Each
sub-matrix is composed of electricity time series of
consumers with similar consumption model. After
that, meaningful results can be obtained when
analysing these sub-matrices by SPCA.
Additionally, to study the relationships between
social background and electricity consumption model
of consumers, we use ACORN to categorize
consumers. Similarly, given a data matrix
that consists of electricity time series of consumers,
we divide it into Q sub-matrices
in the form of
, where
. Each sub-matrix is
composed of electricity time series of consumers in a
same category. Analysing these sub-matrices by
SPCA may reveal another side of the electricity
consumption model of consumers.
Electricity Consumption Model Analysis based on Sparse Principal Components
591