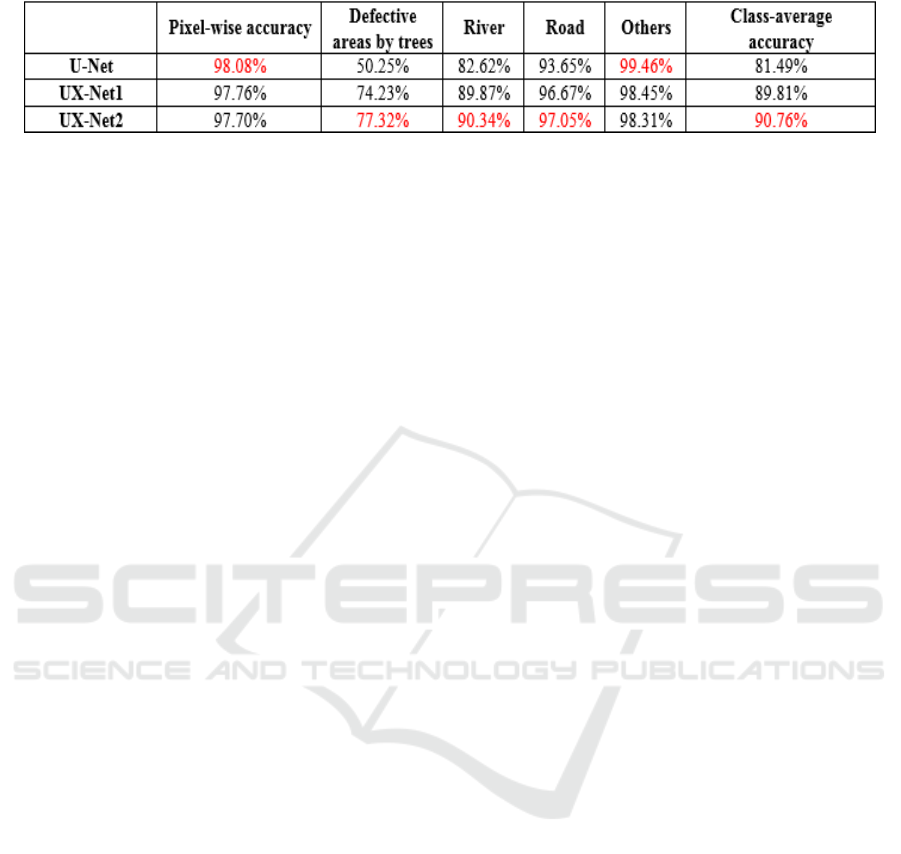
Table 1: Accuracy of the proposed method and U-Net.
However, the size of feature maps of shallow
layer at encoder part and that of beginning layer at
decoder part is different. Thus, we use pooling to be
the same size. Similarly, since the size of deep layer
at encoder part and that of final layer at decoder part
is different, we use unpooling to be the same size.
We use batch normalization (Ioffe and Szegedy,
2015) at each layer though original U-net did not use
it. Class balancing (Badrinarayanan et al., 2016) is
also used to improve the segmentation accuracy of
objects with small area.
3 EXPERIMENTS
We show experimental results on semantic
segmentation in Red Relief Image Map. At first, we
explain the dataset that we use in the following
experiments in section 3.1. Comparison methods are
explained in section 3.2. Experimental results are
shown in section 3.3.
3.1 Dataset
In this paper, we use eleven Red Relief Image Maps.
Five images are used for training images and
remaining six images are used for test. Since some
quantity of training images are necessary for training
deep learning, we crop a local region of 256 x 256
pixels with overlapped ratio 0.7 from Red Relief
Image Map of 1,500 x 2,000 pixels. In addition, we
rotate those cropped regions at the interval of 90
degrees to enlarge the number of training images. As
a result, the number of training images is 7,344. Test
regions of 256 x 256 pixels are cropped without
overlap from the original six images. The total
number of test regions is 185.
3.2 Comparison Methods
We compare our method with some networks
including the original U-net. The first method is the
U-Net. The second method is our proposed method.
When we concatenate the feature maps of different
resolution, the size of each feature map is changed
by pooling and convolution or unpooling and
deconvolution. We call this method “UX-Net1”.
The third method is also our method but we do
not use convolution and deconvolution when we
change the size of feature map. Only pooling and
unpooling are used to change the size of feature
maps. We call this network “UX-Net2”.
3.3 Experimental Results
We show the experimental results of all methods. As
evaluation measure, we use the pixel-wise accuracy
and class average accuracy. Pixel-wise accuracy is
the accuracy in all pixels. This is influenced by
objects of large area such as background. Class-
average accuracy is the average accuracy of each
class. This is influenced by objects of small area
such as defective areas by trees, road and river. In this
paper, class average accuracy is more important than
pixel-wise accuracy because we want to segment
defective areas by trees, road and river well.
We show the segmentation results of all methods
in Figure 3 and 4. The first row shows input image
and ground truth label. The second rows show the
result by U-Net and UX-Net1. The bottom row
shows the result by UX-Net2.
We show the pixel-wise accuracy and the class-
average accuracy of each method in Table 1. The
best result at each class is shown in red.
We found that our proposed UX-Net has higher
accuracy for defective areas by trees, road and river
than the original U-Net. The pixel-wise accuracy of
the proposed method is worse than the U-net
because the pixel-wise accuracy is influenced by the
background which is not the main target.
Note that our proposed method can improve the
accuracy of defective areas by trees that are hard to
segment by the U-net. This is because we use the
“X-path” that the fine information obtained at
shallow layer is used in deep layer and semantic
information obtained at deep layer is used to general
the final segmentation result. When we compare
UX-Net1 with UX-Net2, UX-Net2 gave better result
than UX-Net1. The main difference is how to
change the feature map. Experimental results show
that only pooling and unpooling is effective to
change the size. When we use pooling and
Semantic Segmentation in Red Relief Image Map by UX-Net
599