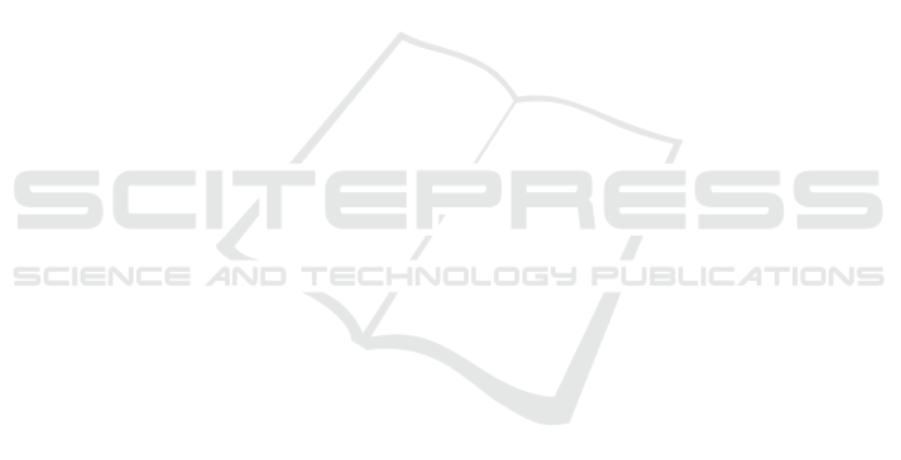
conventional method, and it is possible to prevent to
assign the wrong class label to the large object by
using the appropriate size effectively.
In experiments, our proposed method obtained
87.41% on class average accuracy and about 5%
higher accuracy than conventional method. Our
segmentation method also achieved better accuracy
than the U-net that is one of end-to-end segmentation
deep networks.
However, there are parts that are not segmented
well because the texture of the same object changes
greatly due to road environment such as wetting. We
need to improve this issue in future work.
REFERENCES
Hasegawa, H. Ishiyama, N., 2013, Publication of The digital
maps (basic geospatial Information). Geospatial
Information Authority of Japan, Vol.60, pp.19-24.
Umemura, M., Hotta, K., Nonaka, H., Oda, K., 2016, Image
labeling for LiDAR intensity image using k-nn of
feature obtained by convolutional neural network, Int.
Arch. Photogram. Remote Sens. Spatial Inf. Sci., XLI-
B3, pp. 931-935.
Umemura, M., Hotta, K., Nonaka, H., Oda, K., 2017,
Segmentation of LiDAR Intensity Using CNN feature
Based on Weighted Voting, International Conference
on Image Analysis and Recognition, pp.578-585.
Yan, W. Y., Shaker, A., Habib, A., Kersting, A. P., 2012,
Improving classification accuracy of airborne LiDAR
intensity data by geometric calibration and radiometric
correction. ISPRS journal of photogrammetry and
remote sensing, 67, pp. 35-44.
Ronneberger, O., Fischer, P., Brox, T., 2015, U-net:
Convolutional networks for biomedical image
segmentation. In International Conference on Medical
Image Computing and Computer-Assisted Intervention,
pp. 234-241.
Tighe, J. and Lazebnik, S., 2012, Superparsing, scalable non
parametric image parsing with super pixels. Proc.
European Conference on Computer Vision, pp. 352–
365.
Kohli, P., Osokin, A., Jegelka, S., 2013, A principled deep
random field model for image segmentation. Proc.
IEEE Conference on Computer Vision and Pattern
Recognition pp. 1971-1978.
Hariharan, B., Arbeláez, P., Girshick, R., Malik, J., 2014,
Simultaneous detection and segmentation. Proc.
European Conference on Computer Vision pp. 297-312.
Girshick, R., Donahue, J., Darrell, T.,Malik, J., 2016,
Region-based convolutional networks for accurate
object detection and segmentation. IEEE transactions
on pattern analysis and machine intelligence, Vol.38,
No.1, pp. 142-158.
Jonathan, L., Shelhamer, E., Darrell, T., 2015, Fully
convolutional networks for semantic segmentation.
Proc. IEEE Conference on Computer Vision and
Pattern Recognition, pp. 3431-3440.
Badrinarayanan, V., Kendall, A., Cipolla, R., 2015, Segnet:
A deep convolutional encoder-decoder architecture for
image segmentation. IEEE Transactions on Pattern
Analysis and Machine Intelligence, arXiv 1511.00561.
Jia, Y., Shelhamer, E., Donahue, J., Karayev, S., Long, J.,
Girshick, R., Guadarrama, S. Darrell, T., 2014, Caffe:
Convolutional architecture for fast feature embedding.
Proc. ACM International Conference on Multimedia, pp.
675-678.
Novak, K., 1993, Mobile Mapping System: new tools for
the fast collection of GIS information. Proc. SPIE,
Vol.1943.
Segmentation of Lidar Intensity using Weighted Fusion based on Appropriate Region Size
613