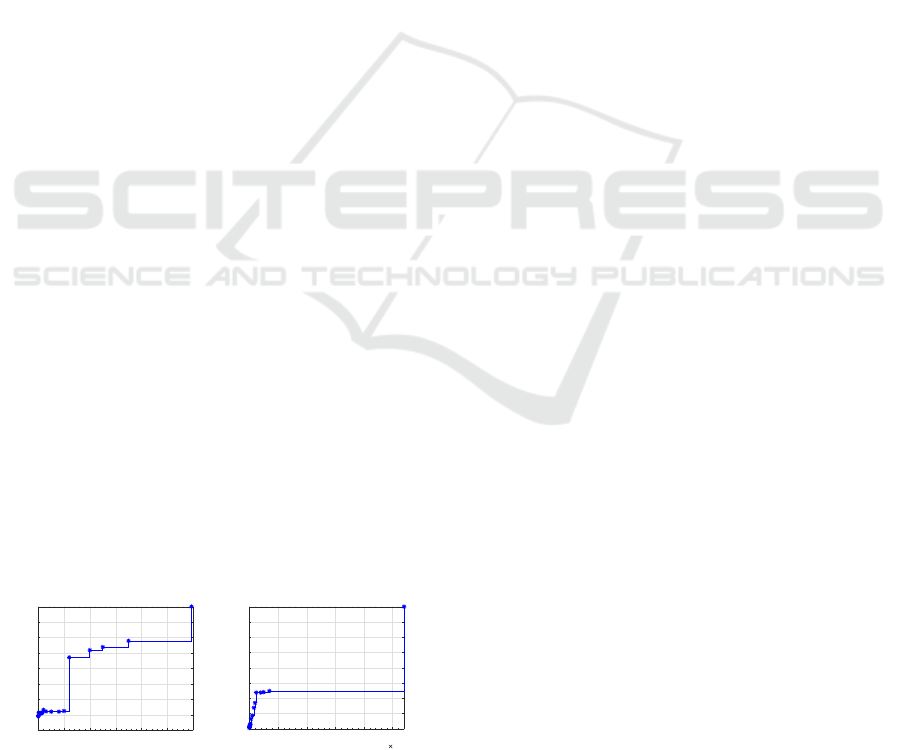
ploy KPCA to gain higher recognition rate with ex-
pense of the higher processing time. This is due to
KPCA is more time consuming than traditional PCA
because of its mapping kernel function, as well as op-
timization of the kernel parameters.
6 CONCLUSIONS AND FUTURE
WORK
At this paper we compared the performances of
the most popular fundamental dimensional reduction
methods, PCA, KPCA, and Isomap, on person re-
identification data sets. The comparison is done
through the experiments conducted by using two des-
criptors on two benchmark data sets. The experi-
mental results evidenced that generated features by
these descriptors might be not well-optimum. PCA
and KPCA outperformed the original CMC curve on
LOMO and gBiCov descriptors on VIPeR and i-LIDS
data sets. This was apparent also from their error es-
timation of projected feature space using two descrip-
tors on two data sets. Both these reduction methods
achieve better performances rather than Isomap met-
hod in person re-identification task. The reason relies
in the fact that PCA and KPCA can explore higher
order information of the original inputs than Isomap.
It is worth to point out that, at this work, PCA was
better than others in terms of the computational cost,
while KPCA was more time consuming with respect
to the other two reduction methods. It therefore can be
stated that PCA achieved promising performance for
handling of optimization of raw data and projection of
it to low-dimensional feature space. We studied this
only for the descriptors with fixed-size feature vector.
Finally, we point out that the optimization of the di-
mensional reduction methods analyzed in this paper
is computationally and numerically practical in real-
time applications. As the future work, we aim at ca-
refully study the behavior of these feature reduction
methods by concerning on some analytical terms, and
visualize the projected data on the actual feature space
to get better prospective on those behaviours.
2 1000 2000 3000 4000 5000 6000
r
0
0.005
0.01
0.015
0.02
0.025
0.03
0.035
0.037
t
M
Maching processing time of gBiCov
0 0.5 1 1.5 2 2.5
r
10
4
0
0.005
0.01
0.015
0.02
0.025
0.03
0.035
0.04
t
M
Maching processing time of LOMO
Figure 4: Average processing time t
M
(in sec.) for com-
puting a matching score for a single probe image and one
template, for each of the two descriptors with different fea-
ture sizes r.
ACKNOWLEDGMENT
We gratefully acknowledge Prof. Giuseppe Rodri-
guez of Dipartimento di Matematica e Informatica at
University of Cagliari, for his initial analysis on the
data and experimental evaluation of this paper.
REFERENCES
Balasubramanian, M. and Schwartz, E. L. (2002). The
isomap algorithm and topological stability. Science,
295(5552):7–7.
Bedagkar-Gala, A. and Shah, S. K. (2014). A survey of ap-
proaches and trends in person re-identification. Image
and Vision Computing, 32(4):270–286.
Cheng, D. S., Cristani, M., Stoppa, M., Bazzani, L., and
Murino, V. (2011). Custom pictorial structures for re-
identification. In BMVC, page 6.
Diamantaras, K. I. and Kung, S. Y. (1996). Principal
component neural networks: theory and applications.
John Wiley & Sons, Inc.
Dijkstra, E. W. (1959). A note on two problems in connex-
ion with graphs. Numerische mathematik, 1(1):269–
271.
Dikmen, M., Akbas, E., Huang, T. S., and Ahuja, N. (2010).
Pedestrian recognition with a learned metric. In Com-
puter Vision–ACCV 2010, pages 501–512. Springer.
Dutra, C. R., Schwartz, W. R., Souza, T., Alves, R.,
and Oliveira, L. (2013). Re-identifying people
based on indexing structure and manifold appea-
rance modeling. In Graphics, Patterns and Images
(SIBGRAPI), 2013 26th SIBGRAPI-Conference on,
pages 218–225. IEEE.
Farenzena, M., Bazzani, L., Perina, A., Murino, V., and
Cristani, M. (2010). Person re-identification by
symmetry-driven accumulation of local features. In
Computer Vision and Pattern Recognition (CVPR),
2010 IEEE Conference on, pages 2360–2367. IEEE.
Fisher, R. A. (1936). The use of multiple measurements
in taxonomic problems. Annals of human genetics,
7(2):179–188.
Gray, D. and Tao, H. (2008). Viewpoint invariant pedestrian
recognition with an ensemble of localized features. In
Computer Vision–ECCV 2008, pages 262–275. Sprin-
ger.
Hotelling, H. (1933). Analysis of a complex of statistical
variables into principal components. Journal of edu-
cational psychology, 24(6):417.
Khedher, M. I. and El Yacoubi, M. A. (2015). Two-stage
filtering scheme for sparse representation based inte-
rest point matching for person re-identification. In Ad-
vanced Concepts for Intelligent Vision Systems, pages
345–356. Springer.
Lavi, B., Fumera, G., and Roli, F. (2016). A multi-stage
approach for fast person re-identification. In Joint
IAPR International Workshops on Statistical Techni-
ques in Pattern Recognition (SPR) and Structural and
ICPRAM 2018 - 7th International Conference on Pattern Recognition Applications and Methods
620