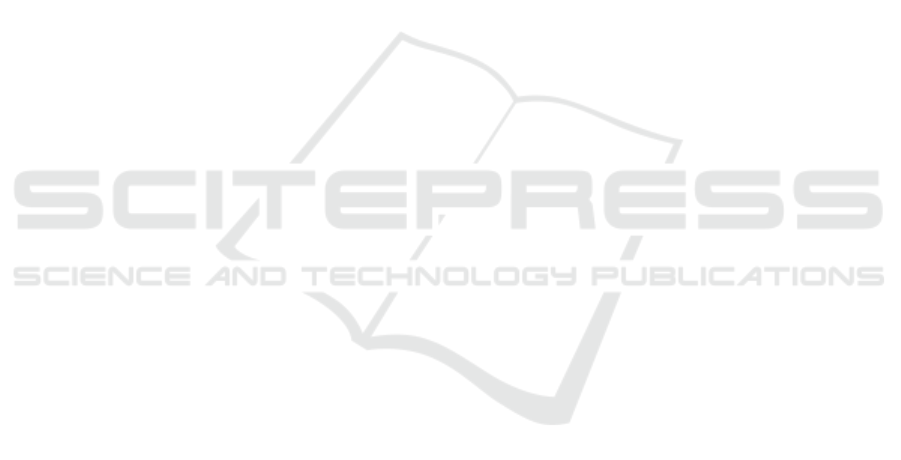
bility of the results, the choice of the thresholds and
the choice of the right features is critical but hard to
achieve in general. While one reason might be the
absence of ground truth information another reason
might be the diversity of the consumption patterns of
the households.
This work sets the starting point for holiday detec-
tion and raises a number of technical issues for future
work: modeling and removal of background appli-
ances, choice of thresholds, feature selection, proper
modeling and smoothing of the day-dependent night
distributions, inclusion of other predictive variables
like day of the week and of course evaluation for la-
beled datasets.
Considering the privacy perspective it would be
interesting to investigate possible privacy conse-
quences apart from the detection of secondary resi-
dences.
ACKNOWLEDGEMENTS
The financial support by the Austrian Federal Min-
istry of Science, Research and Economy, the Aus-
trian National Foundation for Research, Technology
and Development and the Federal State of Salzburg is
gratefully acknowledged.
REFERENCES
Akbar, A., Nati, M., Carrez, F., and Moessner, K. (2015).
Contextual occupancy detection for smart office by
pattern recognition of electricity consumption data. In
IEEE International Conference on Communications,
pages 561–566.
Becker, V. and Kleiminger, W. (2017). Exploring zero-
training algorithms for occupancy detection based on
smart meter measurements. Computer Science - Re-
search and Development, pages 1–12.
Buescher, N., Boukoros, S., Bauregger, S., and Katzen-
beisser, S. (2017). Two Is Not Enough: Privacy As-
sessment of Aggregation Schemes in Smart Meter-
ing. Proceedings on Privacy Enhancing Technologies,
2017(4):118–134.
Chen, D., Barker, S., Subbaswamy, A., Irwin, D., and
Shenoy, P. (2013). Non-Intrusive Occupancy Moni-
toring using Smart Meters. In Proceedings of the 5th
ACM Workshop on Embedded Systems For Energy-
Efficient Buildings - BuildSys’13, pages 1–8.
Eibl, G. and Engel, D. (2015). Influence of Data Granularity
on Smart Meter Privacy. IEEE Transactions on Smart
Grid, 6(2):930–939.
Hart, G. W. (1992). Nonintrusive appliance load monitor-
ing. Proceedings of the IEEE, 80(12):1870–1891.
Hattori, S. and Shinohara, Y. (2017). Actual Consumption
Estimation Algorithm for Occupancy Detection using
Low Resolution Smart Meter Data. In Proceedings of
the 6th International Conference on Sensor Networks
- SENSORNETS, pages 39–48.
Jin, M., Jia, R., and Spanos, C. (2017). Virtual Occu-
pancy Sensing: Using Smart Meters to Indicate Your
Presence. IEEE Transactions on Mobile Computing,
16(11):3264–3277.
Kim, H., Marwah, M., Arlitt, M. F., Lyon, G., and Han,
J. (2011). Unsupervised Disaggregation of Low Fre-
quency Power Measurements. In The 11th SIAM In-
ternational Conference on Data Mining, pages 747–
758.
Kleiminger, W., Beckel, C., and Santini, S. (2015). House-
hold Occupancy Monitoring Using Electricity Meters.
In Proceedings of the 2015 ACM International Joint
Conference on Pervasive and Ubiquitous Computing,
pages 975–986.
Kleiminger, W., Beckel, C., Staake, T., and Santini, S.
(2013). Occupancy Detection from Electricity Con-
sumption Data. In Proceedings of the 5th ACM
Workshop on Embedded Systems For Energy-Efficient
Buildings - BuildSys’13, pages 1–8.
Lisovich, M. A. and Wicker, S. B. (2008). Privacy Concerns
in Upcoming Residential and Commercial Demand-
Response Systems. In Clemson Power Systems Con-
ference.
Tang, G., Wu, K., Lei, J., and Xiao, W. (2015). The meter
tells you are at home! Non-intrusive occupancy detec-
tion via load curve data. In 2015 IEEE International
Conference on Smart Grid Communications, (Smart-
GridComm), pages 897–902.
Zoha, A., Gluhak, A., Imran, M. A., and Rajasegarar, S.
(2012). Non-intrusive Load Monitoring approaches
for disaggregated energy sensing: A survey. Sensors
(Switzerland), 12(12):16838–16866.
ICISSP 2018 - 4th International Conference on Information Systems Security and Privacy
486