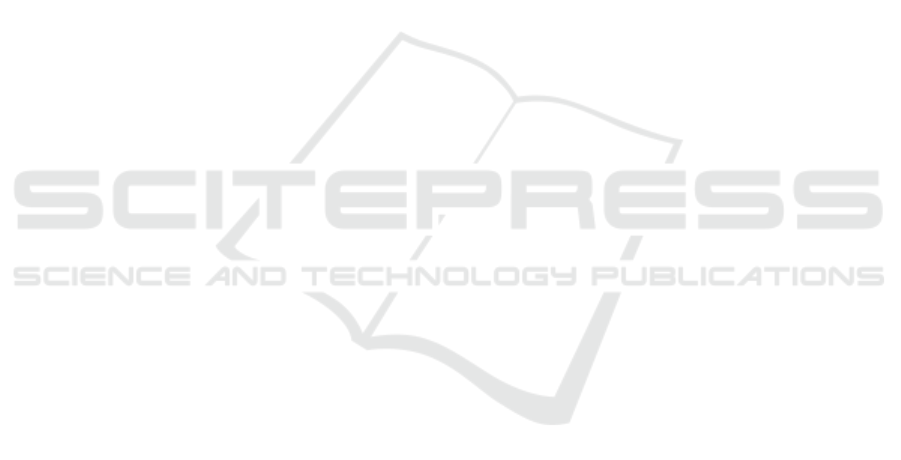
of daily use and depreciation) and the satisfaction of
customers (fares and time spent waiting and travel-
ing). This system and the numerous possible settings
allow the flexible design of a multi-criteria objective
function relevant to any desired optimisation. Next,
the results obtained with the different decentralised
strategies must be compared with the optimal assign-
ment in order to determine the absolute effectiveness
of the proposed approch.
As electric vehicles are becoming more and more
popular, a new dimension similarly becomes essen-
tial. The notable difference between a petrol-powered
vehicle and an electric vehicle is that the latter re-
quires a downtime for its recharging. It is obviously
not desirable for all taxis to recharge at the same time.
A collective energy management policy is thus re-
quired, leading to a modification of individual strate-
gies. A taxi must be able to recharge during empty
periods or with an offset during a full period. This
obviously impacts the fleet size: it becomes more and
more important as the longer the recharging time.
REFERENCES
Badeig, F., Balbo, F., Scemama, G., and Zargayouna, M.
(2008). Agent-based coordination model for design-
ing transportation applications. In IEEE Intelligent
Transportation Systems, 2008., pages 402–407. IEEE.
Barcel
´
o, J., Codina, E., Casas, J., Ferrer, J., and Garc
´
ıa, D.
(2005). Microscopic traffic simulation: A tool for the
design, analysis and evaluation of intelligent transport
systems. Journal of Intelligent and Robotic Systems,
41(2-3):173–203.
Bazzan, A. and Klgl, F. (2014). A review on agent-based
technology for traffic and transportation. The Knowl-
edge Engineering Review, FirstView:1–29.
Bessghaier, N., Zargayouna, M., and Balbo, F. (2012). An
agent-based community to manage urban parking. Ad-
vances in Intelligent and Soft Computing, 155:17–22.
Billhardt, H., Fern
´
andez, A., Lemus, L., Lujak, M., Os-
man, N., Ossowski, S., and Sierra, C. (2014). Dy-
namic coordination in fleet management systems: To-
ward smart cyber fleets. IEEE Intelligent Systems,
29(3):70–76.
Bonhomme, A., Mathieu, P., and Picault, S. (2014). A ver-
satile description framework for modeling behaviors
in traffic simulations. In Papadopoulos, G., editor,
IEEE 26th Int. Conf. on Tools with Artificial Intelli-
gence (ICTAI’2014), pages 937–944. IEEE.
Bonhomme, A., Mathieu, P., and Picault, S. (2016). A ver-
satile multi-agent traffic simulator framework based
on real data. International Journal on Artificial Intel-
ligence Tools, 25(1):20.
Bonte, L., Mathieu, P., and Espi, S. (2006). Modlisation et
simulation des usagers des deux-roues motoriss dans
archisim. In Chevrier, V., editor, JFSMA, pages 31–
44.
Cheikh, S. B. and Hammadi, S. (2014). The alliance be-
tween optimization and multi-agent system for the
management of the dynamic carpooling. In Agent and
Multi-Agent Systems: Technologies and Applications,
pages 193–202. Springer.
Dresner, K. and Stone, P. (2008). A multiagent approach to
autonomous intersection management. J. Artif. Intell.
Res. (JAIR), 31:591–656.
Lacroix, B., Mathieu, P., and Kemeny, A. (2009). Gener-
ating various and consistent behaviors in simulations.
In Demazeau, Y. et al., editors, PAAMS, volume 55 of
Practical Advances in Intelligent and Soft Computing,
pages 110–119.
Lacroix, B., Mathieu, P., and Kemeny, A. (2013). Formaliz-
ing the construction of populations in multi-agent sim-
ulations. J. Eng. App. of AI, 26(1):211–226.
Lioris, E., Cohen, G., and de La Fortelle, A. (2010). Evalu-
ation of collective taxi systems by discrete-event sim-
ulation. In SIMUL, pages 34–39. IEEE.
Maciejewski, M. and Nagel, K. (2013). The influence of
multi-agent cooperation on the efficiency of taxi dis-
patching. In ICPPAM, pages 751–760.
Mandiau, R., Champion, A., Auberlet, J.-M., Espi, S., and
Kolski, C. (2008). Behaviour based on decision matri-
ces for a coordination between agents in a urban traffic
simulation. Appl. Intell., 28(2):121–138.
Manley, E., Cheng, T., Penn, A., and Emmonds, A. (2014).
A framework for simulating large-scale complex ur-
ban traffic dynamics through hybrid agent-based mod-
elling. Computers, Environment and Urban Systems,
pages 27–36.
Smith, R. (1980). The contract net protocol: Highlevel
communication and control in a distributed problem
solver. IEEE Trans. on Computers, C, 29:12.
Tlig, M., Buffet, O., and Simonin, O. (2012). Coopera-
tive behaviors for the self-regulation of autonomous
vehicles in space sharing conflicts. In ICTAI, pages
1126–1132. IEEE.
Yang, Q. (1997). Simulation Laboratory for Evaluating Dy-
namic Traffic Management Systems. Thse de doctorat,
Massachusetts Institute of Technology.
ICAART 2018 - 10th International Conference on Agents and Artificial Intelligence
304