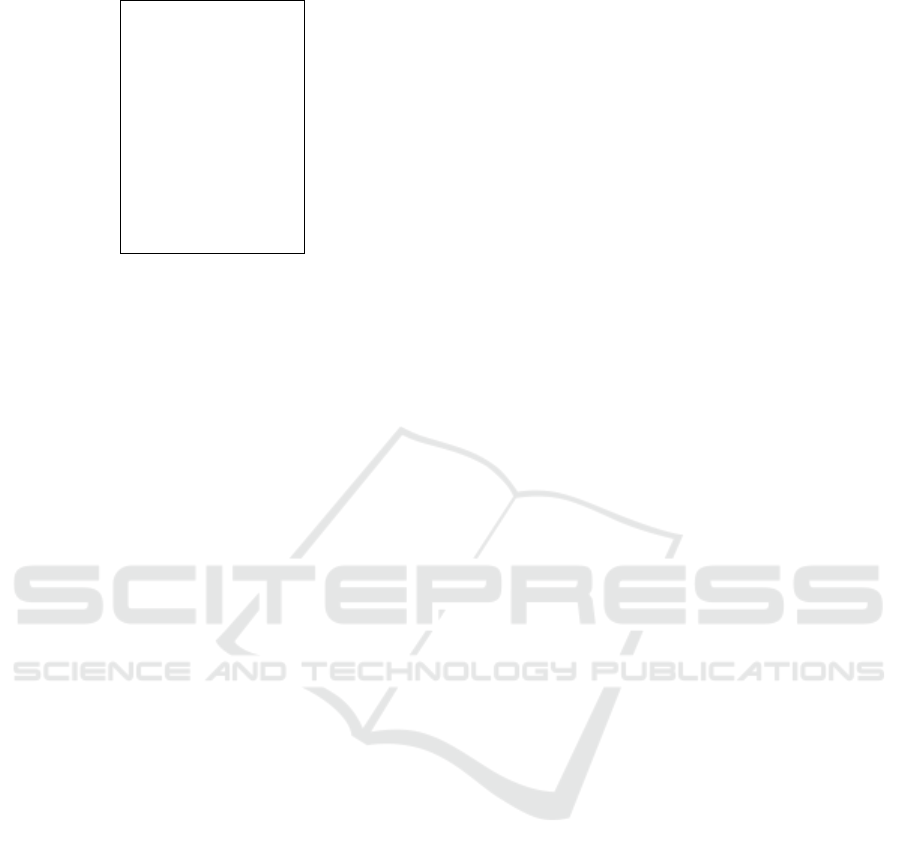
Table 6: Average human rankings of 11 idiom types.
hold fire 3.28
hold horse 3.37
blow whistle 3.16
have word 2.29
give sack 3.33
take hear 3.30
lose head 3.35
make scene 3.02
hit wall 3.19
hit roof 3.34
blow top 3.44
ACKNOWLEDGEMENTS
This material is based upon work supported by
the National Science Foundation under Grant No.
1319846
REFERENCES
Birke, J. and Sarkar, A. (2006). A clustering approach to
the nearly unsupervised recognition of nonliteral lan-
guage. In Proceedings of the 11th Conference of the
European Chapter of the Association for Computa-
tional Linguistics (EACL’06), pages 329–226, Trento,
Italy.
Bojanowski, P., Grave, E., Joulin, A., and Mikolov, T.
(2016). Enriching word vectors with subword infor-
mation. CoRR, abs/1607.04606.
Boukobza, R. and Rappoport, A. (2009). Multi-word ex-
pression identification using sentence surface features.
In Proceedings of the 2009 Conference on Empirical
Methods in Natural Language Processing: Volume 2-
Volume 2, pages 468–477. Association for Computa-
tional Linguistics.
Bu, F., Zhu, X., and Li, M. (2010). Measuring the non-
compositionality of multiword expressions. In Pro-
ceedings of the 23rd International Conference on
Computational Linguistics, pages 116–124. Associa-
tion for Computational Linguistics.
Cook, P., Fazly, A., and Stevenson, S. (2007). Pulling their
weight: Exploiting syntactic forms for the automatic
identification of idiomatic expressions in context. In
Proceedings of the ACL 07 Workshop on A Broader
Perspective on Multiword Expressions, pages 41–48.
Cook, P., Fazly, A., and Stevenson, S. (2008). The VNC-
Tokens Dataset. In Proceedings of the LREC Work-
shop: Towards a Shared Task for Multiword Expres-
sions (MWE 2008), Marrakech, Morocco.
Cordeiro, S., Ramisch, C., Idiart, M., and Villavicencio, A.
(2016). Predicting the compositionality of nominal
compounds: Giving word embeddings a hard time. In
Proceedings of the 54th Annual Meeting of the Associ-
ation for Computational Linguistics (Volume 1: Long
Papers), pages 1986–1997. Association for Computa-
tional Linguistics.
Fazly, A., Cook, P., and Stevenson, S. (2009). Unsuper-
vised Type and Token Identification of Idiomatic Ex-
pressions. Computational Linguistics, 35(1):61–103.
Firth, J. R. (1957). {A synopsis of linguistic theory, 1930-
1955}.
Fukunaga, K. (1990). Introduction to statistical pattern
recognition. Academic Press.
Hearst, M. A. (1992). Automatic acquisition of hyponyms
from large text corpora. In Proceedings of the 14th
Conference on Computational Linguistics - Volume 2,
COLING ’92, pages 539–545, Stroudsburg, PA, USA.
Association for Computational Linguistics.
Katz, G. and Giesbrecht, E. (2006). Automatic Identifi-
cation of Non-compositional Multiword Expressions
using Latent Semantic Analysis. In Proceedings of
the ACL/COLING-06 Workshop on Multiword Expres-
sions: Identifying and Exploiting Underlying Proper-
ties, pages 12–19.
Li, L. and Sporleder, C. (2010). Using gaussian mixture
models to detect figurative language in context. In
Proceedings of NAACL/HLT 2010.
Lubensky, S. (2013). Russian-English Dictionary of Idioms.
Yale University Press.
Mikolov, T., Chen, K., Corrado, G., and Dean, J. (2013a).
Efficient estimation of word representations in vector
space. In Proceedings of Workshop at ICLR.
Mikolov, T., Sutskever, I., Chen, K., Corrado, G., and Dean,
J. (2013b). Distributed representations of words and
phrases and their compositionality. In Proceedings of
NIPS.
Peng, J. and Feldman, A. (2016a). Experiments in idiom
recognition. In Proceedings of the 26th International
Conference on Computational Linguistics (COLING).
Peng, J. and Feldman, A. (2016b). Experiments in idiom
recognition. In COLING, pages 2752–2762.
Peng, J. and Feldman, A. (2016c). In god we trust. all oth-
ers must bring data. — w. edwards deming — using
word embeddings to recognize idioms. In Proceedings
of the 3rd Annual International Symposium on Infor-
mation Management and Big Data — SIMBig, Cusco,
Peru.
Peng, J., Feldman, A., and Jazmati, H. (2014a). Classifying
idiomatic and literal expressions using topic models
and intensity of emotions. In Proceedings of the 2014
Empirical Methods for Natural Language Processing
Conference (EMNLP).
Peng, J., Feldman, A., and Jazmati, H. (2015a). Classi-
fying idiomatic and literal expressions using vector
space representations. In Proceedings of the Recent
Advances in Natural Language Processing (RANLP)
conference 2015, Hissar, Bulgaria.
Peng, J., Feldman, A., and Jazmati, H. (2015b). Classifying
idiomatic and literal expressions using vector space
representations. In RANLP, pages 507–511.
Peng, J., Feldman, A., and Vylomova, E. (2014b). Clas-
sifying idiomatic and literal expressions using topic
models and intensity of emotions. In Proceedings of
the 2014 Conference on Empirical Methods in Natural
Language Processing (EMNLP), pages 2019–2027,
A Distributional Semantics Model for Idiom Detection - The Case of English and Russian
681