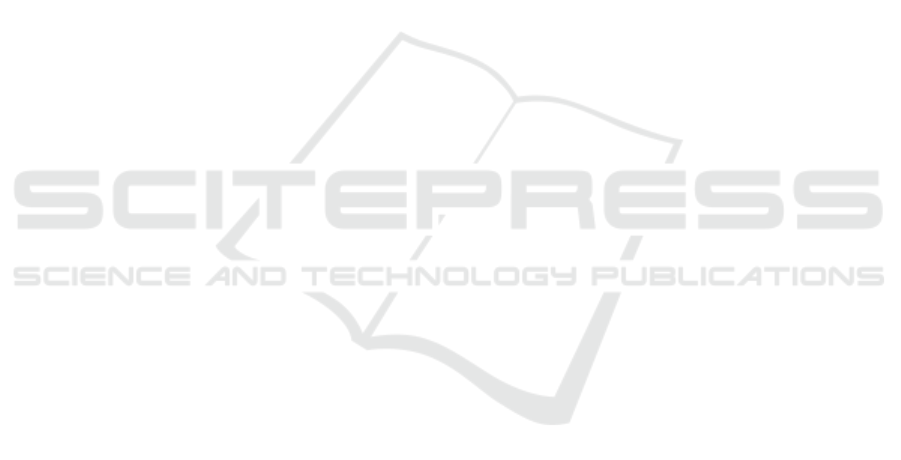
the values of dominant and competitive are nealy 0.
The third image, however, are taken in one compe-
tition so the expression of two students is quite seri-
ous. As a result, the value of competitive is the highest
comparing to the others.
6 CONCLUSION
In this study, the authors show that applying our fa-
cial multi-attribute network can overcome the diffi-
culties of predicting social relation traits from visual
contents. Previous work, which mainly focuses on the
conventional deep neural networks, only capable of
producing generic results of all the relevant attributes
without any evaluation upon the contribution of each
attributes or groups of them to the overall perfor-
mance. Our model has proven its availability towards
different adjustments and feasible application to other
lightweight systems that aim to specific purposes or
specialized datasets. We will explore some feasible
applications including background music recommen-
dation for video based and photo collection clustering
and visualization based on social traits.
REFERENCES
Bettadapura, V. (2012). Face expression recognition and
analysis: The state of the art. Computer Vision and
Pattern Recognition.
Ding, L. and Yilmaz, A. (2010). Learning Relations among
Movie Characters: A Social Network Perspective,
pages 410–423. Springer Berlin Heidelberg, Berlin,
Heidelberg.
Dodge, M. and Kitchin, R. (2007). outlines of a world com-
ing into existence: Pervasive computing and the ethics
of forgetting. Environment and Planning B: Planning
and Design, 34(3):431–445.
Fathi, A., Hodgins, J. K., and Rehg, J. M. (2012). Social
interactions: A first-person perspective. In 2012 IEEE
Conference on Computer Vision and Pattern Recogni-
tion, pages 1226–1233.
Gurrin, C. (2016). A guide to creating and managing lifel-
ogs. In ACM MM 2016 Tutorial, November 15, 2016,
Amsterdam.
Gurrin, C., Smeaton, A. F., and Doherty, A. R. (2014).
Lifelogging: Personal big data. Foundations and
Trends in Information Retrieval, 8(1):1–125.
Kaggle (2017). Challenges in representation learning facial
expression recognition challenge.
Kiesler, D. J. (1983). The 1982 Interpersonal Circle: A
taxonomy for complementarity in human transactions.
Psychological Review.
Koestinger, M., Wohlhart, P., Roth, P. M., and Bischof, H.
(2011). Annotated facial landmarks in the wild: A
large-scale, real-world database for facial landmark
localization. In Proc. First IEEE International Work-
shop on Benchmarking Facial Image Analysis Tech-
nologies.
Lan, T., Sigal, L., and Mori, G. (2012). Social roles in hi-
erarchical models for human activity recognition. In
2012 IEEE Conference on Computer Vision and Pat-
tern Recognition, pages 1354–1361.
Lopes, T., de Aguiar, E., Souza, A. F. D., and Oliveira-
Santos, T. (2016). Facial expression recognition with
convolutional neural networks: Coping with few data
and the training sample order. Pattern Recognition.
Nguyen, V., Le, K., Tran, M., and Fjeld, M. (2016).
NowAndThen: a social network-based photo recom-
mendation tool supporting reminiscence. In Proceed-
ings of the 15th International Conference on Mobile
and Ubiquitous Multimedia, Rovaniemi, Finland, De-
cember 12-15, 2016, pages 159–168.
Nguyen, V., Ngo, T. D., Le, D., Tran, M., Duong, D. A.,
and Satoh, S. (2017). Semantic extraction and object
proposal for video search. In MultiMedia Modeling
- 23rd International Conference, MMM 2017, Reyk-
javik, Iceland, January 4-6, 2017, Proceedings, Part
II, pages 475–479.
Parkhi, O. M., Vedaldi, A., and Zisserman, A. (2015). Deep
face recognition. In British Machine Vision Confer-
ence.
Rothe, R., Timofte, R., and Gool, L. V. (2016). Deep ex-
pectation of real and apparent age from a single im-
age without facial landmarks. International Journal
of Computer Vision (IJCV).
Salvador, A., i Nieto, X. G., Marqus, F., and Satoh, S.
(2016). Faster R-CNN features for instance search. In
2016 IEEE Conference on Computer Vision and Pat-
tern Recognition Workshops (CVPRW), pages 394–
401.
Schroff, F., Kalenichenko, D., and Philbin, J. (2015).
Facenet: A unified embedding for face recognition
and clustering. In The IEEE Conference on Computer
Vision and Pattern Recognition (CVPR).
Szegedy, C., Ioffe, S., and Vanhoucke, V. (2016). Inception-
v4, inception-resnet and the impact of residual con-
nections on learning. Computer Vision and Pattern
Recognition.
Valstar, M. F., Jiang, B., and Mehu, M. (2011). The first
facial expression recognition and analysis challenge.
Automatic Face & Gesture Recognition and Work-
shops (FG 2011).
Wen, Y., Zhang, K., Li, Z., and Qiao, Y. (2016). A Discrim-
inative Feature Learning Approach for Deep Face
Recognition, pages 499–515. Springer International
Publishing, Cham.
Zhang, Z., Luo, P., Loy, C. C., and Tang, X. (2015). Learn-
ing social relation traits from face images. 2015 IEEE
International Conference on Computer Vision.
Zhou, L. M., Gurrin, C., Yang, C., and Qiu, Z. (2013). From
lifelog to diary: a timeline view for memory reminis-
cence. In Irish HCI conference 2013, Ireland, Dun-
dalk.
INDEED 2018 - Special Session on INsights DiscovEry from LifElog Data
674