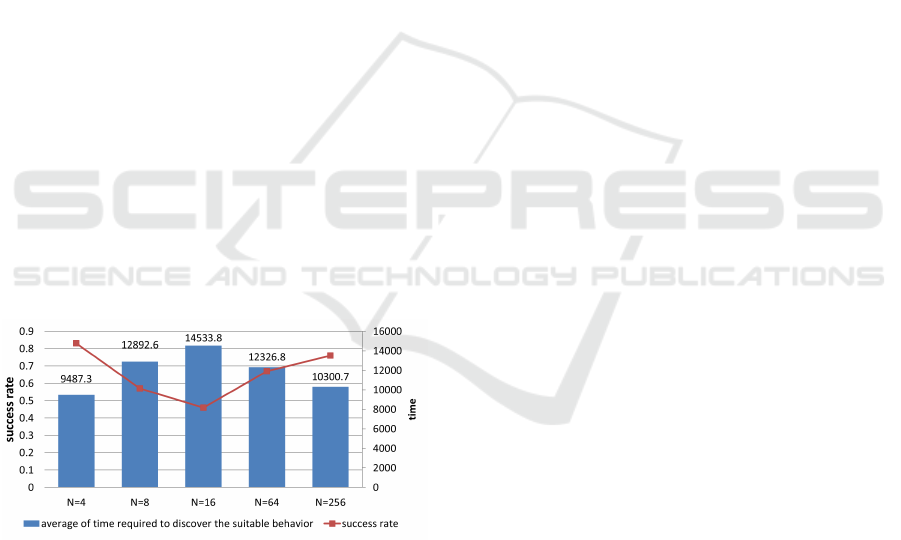
4.3.2 Influence of the Number of Agents N
Here, we make clear how the number of agents in-
fluence the searching ability of the desired behavior.
We set r = 100, a
goal
= a
4
. Assuming that all the
agents selects an agreed behavior at the initial time.
We changed the radius r and counted the cases where
all of the agents were able to select a
4
. Figure 12
shows the results of performing 100 trials for a num-
ber of agents N = 4,8,16,64,256. A trial is termi-
nated at time step 30000.
The vertical axis on the left is the ratio at which
behavior a
4
was successfully agreed (red line), the
vertical axis on the right is average of time required
to do that (blue bar), and the horizontal axis shows
the number of agents N. As can be seen, when the
number of agents is set a very small value (about 4
∼ 16), the success ratio surprisingly decreased with
an increase in N. It is considered that the number of
agents is too small, and there is almost no neighbor.
Therefore, each agent makes decisions without being
affected by other agents. At this time, the probability
of selecting the desired behavior a
4
by all individu-
als could be 1/(3N). Thus, the smaller the number of
agents N is, the higher success ratio could be. How-
erver, when the number of agents is set a bigger value,
the density of agents in the domain increases and the
number of neighbors of each agents increases too.
Therefore, the probability that individual information
is indirectly conveyed to everyone increases. As can
be seen, the success ratio increases when N ≥ 16.
Figure 12: The changing of the success ratio and the aver-
age of time required to reach the desired behavior from an
agreed state in the initial time. N = 4,8, 16,64,256.
5 CONCLUSIONS
In this paper, we introduced the improved BRT al-
gorithm based on Local Information Sharing (BRT-
Lis) and considered how to adjust the local parameters
so that the desired behavior could be obtained opti-
mally. We conducted the experiments that confirm the
behavior switching function of BRT-Lis with a sim-
ple position changing model. We also examined how
the Local Information Sharing influences the search-
ing ability of the desired behavior. The experimen-
tal result revealed that the following implications: (1)
BRT-Lis model can discover the desired behavior in
the same as of BRT model with an suitable radius r
and (2) the extremely low density or high density of
agents in the domain is the condition of high success
ratio of searching the desired behavior.
REFERENCES
Tofts, C. and N. R. Franks, “Doing the right thing: ants,
honeybees and naked molerats”, Trends Ecol, Vol. 7,
pp. 346–349, 1992.
Page, R. E. and S. D. Mitchell, “The genetics of division of
labour in honey beecolonies”, Adv. Ins. Physiol, Vol.
23, pp. 117–169, 1991.
Gordon, D. M., B. C. Goodwin and L. E. H. Trainor,
“A parallel distributed model of the behaviour of ant
colonies”, J. Theor. Biol, Vol. 156, pp. 293–307, 1992.
Eric Bonabeau, Guy Theraulaz and Jean-Louis
Deneubourg, “Fixed Response Thresholds and
the Regulation of Division of Labor in Insect Soci-
eties”, Bulletin of Mathematical Biology, Vol. 60, pp.
753–807, 1998.
E. Castello, T. Yamamoto, F.D. Libera, W. Liu, F. Alan,
T.Wineld, Y.Nakamura, H.Ishiguro, “Adaptive Forag-
ing for Simulated and Real Robotic Swarms: The dy-
namical response threshold approach”, Swarm Intelli-
gence DOI, Vol. 10, pp. 1–31, 2016.
Akira Namatame (In Japanese), “Strategic Decision Mak-
ing”, Asakura Publisher, pp. 119–149, 2001.
Masao Kubo, Nhu Hai Phung, Hiroshi Sato, Akira Na-
matame, “Direction switch behavior to enclose a pack
of targets based on phase transition”, Swarm2015,
2015.
Nhu Hai Phung, Masao Kubo, Hiroshi Sato, Saori Iwanaga,
Akira Namatame, “Agreement algorithm with trial
and error method at macro level”, Swarm2017, 2017.
Wilson, E. O., “The relation between caste ratios and
division of labour in the ant genus Pheidole (Hy-
menoptera: Formicidae)”, Behav. Ecol. Sociobiol,
1984.
HAMT 2018 - Special Session on Human-centric Applications of Multi-agent Technologies
344