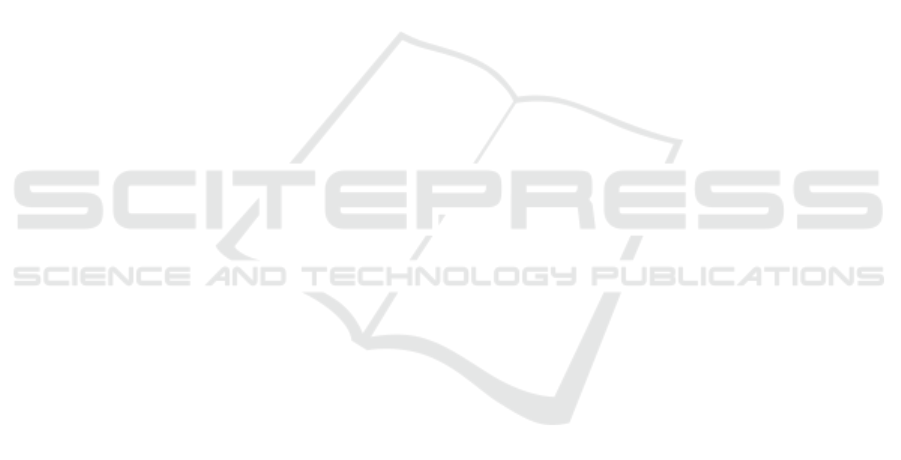
tional cost of their method is really large and cannot
be employed in real time. Although GPU with high
performance can deal with this problem, the price of
GPU is really expensive and not suitable for small de-
vices. Furthermore, resizing a tiny image into a large
image really does not get more information of an im-
age. Additionally, training a large network takes a lot
of time and requires the hardware to be really power-
ful enough.
A good solution for this problem is to keep the size
of the image and to build a network with fewer param-
eters but it still has the ability to recognize with high
accuracy. On this basis, we propose a new method
to employ very deep CNN called Lightweight Deep
Convolutional Network for Tiny Object Recognition
(DCTI). Our proposed network has not only fewer pa-
rameters but also high performance on the tiny im-
age. It has both good accuracy and minimal com-
putational cost. Through experiments, we achieved
some good results which are quite effective for multi-
purposes. This is the motivations for us to continue
to develop our method and build many systems which
make use of object recognition such as understanding
image systems, image search engine systems.
Contributions. In our work, we consider in tiny
images with size 32 × 32. We focus on exploiting
local features with small convolutional filters size.
Therefore, we use convolutional filters size 3 × 3. It
fits with tiny images and helps to extract local fea-
tures. Besides that, it helps reducing parameters and
to push network going deeper.
In traditional approaches, the last layers use fully
connected layers to feed feature maps to feature vec-
tors. However, it increases more parameters and leads
to over-fitting. Our network proposes using global av-
erage pooling (Lin et al., 2013) instead of fully con-
nected layers. The purpose of this work is to help the
network directly project significant feature maps into
the feature vectors. Additionally, global average pool-
ing layers do not employ parameters. So it has fewer
parameters and over-fitting is avoided.
In deep networks, small changes can amplify layer
by layer. It leads to change distribution each layer.
This problem is called Internal Covariate Shift. To
tackle this problem, we use Batch-Normalization pro-
posed by Ioffe et. al. (Ioffe and Szegedy, 2015).
Once again, through experiments, we prove batch-
normalization is potential and efficient. It also helps
faster learning.
Additionally, to prevent over-fitting, we use
dropout. In common, dropout is put after fully con-
nected layers. But in our network, we put it after
convolutional layers. Through experiment, this work
helps improving accuracy and to avoid over-fitting.
We also use data augmentation and whitening
data to improve accuracy. Our method only uses
21.33% number of parameters than the state-of-the-
art method (Zagoruyko and Komodakis, 2016). How-
ever, we achieve the accuracy up to 94.34% and
73.65% on CIFAR-10 and CIFAR-100. With our re-
sult we achieved, it proves that our method not only
gets high accuracy but also reduce parameters signif-
icantly.
The rest of this paper is organized as follows. Sec-
tion 2 presents related works. The proposed architec-
ture of our network is presented in Section 3. Section
4 presents our experimental configuration on CIFAR-
10 and CIFAR-100. We compare our results to other
methods in section 5. Finally, Section 6 concludes the
paper.
2 RELATED WORKS
The earlier method for object recognition named Con-
volutional Neural Networks is proposed by Yann Le-
cun et. al. (LeCun et al., 1989). It demonstrates
high performance on MNIST Dataset. Many current
architectures used for object recognition are based
on Convolutional Neural Networks (Graham, 2014),
(Krizhevsky et al., 2017a), (Zeiler and Fergus, 2013).
Very Deep Convolutional Neural Networks: a
method proposed by Andrew Zisserman et. al. (Si-
monyan and Zisserman, 2014). It has good perfor-
mance on ImageNet Dataset. Very deep convolu-
tional neural networks have two main architectures
are VGG-16 and VGG-19. VGG-16 and VGG-19
mean that there are 16 layers and 19 layers having
parameters. The main contribution of its paper is a
thorough evaluation of networks of increasing depth
using an architecture with very small (3 × 3) convo-
lution filters, which shows that a significant improve-
ment on the prior-art configurations can be achieved
by pushing the depth to 16-19 weight layer.
Network In Network: notice the limitations of
using the fully connected layer, a novel network struc-
ture called Network In Network (NIN) to enhance the
model discriminability for local receptive fields (Lin
et al., 2013). Global average pooling is used in this
network instead of fully connected layer. The pur-
pose of this work is to reduce parameters and enforc-
ing correspondences between feature maps and cate-
gories. It continues improving by Batch-normalized
Maxout and has good performance on CIFAR-10
dataset (Chang and Chen, 2015). In our work, we
also use global average pooling approach.
Deep Residual Learning for Image Recogni-
tion: one of the limitations when the network has
INDEED 2018 - Special Session on INsights DiscovEry from LifElog Data
676