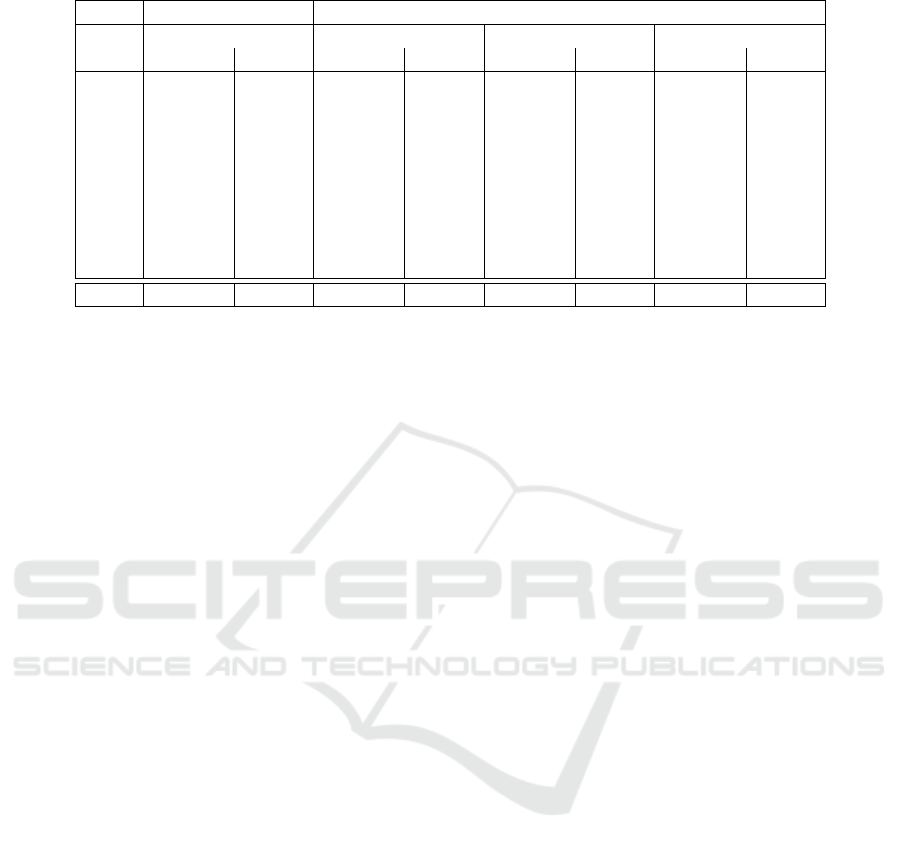
Table 2: Comparison: Replicator Neural Network vs. Isolation Forest.
τ = Q
95
(S(X))
AUC γ Recall Precision
ReplNN Iforest ReplNN Iforest ReplNN Iforest ReplNN Iforest
K1 1.0000 1.0000 0.2762 0.0444 1.0000 1.0000 0.0167 0.0270
K2 1.0000 1.0000 0.0912 0.1347 1.0000 1.0000 0.0909 0.0769
K3 1.0000 1.0000 0.5634 0.1773 1.0000 1.0000 0.0182 0.1429
K4 1.0000 0.9895 0.0699 0.0103 1.0000 1.0000 0.0400 0.3333
K5 0.7500 0.9356 0.1249 0.0833 0.7500 0.5833 0.6000 0.7778
K6 0.8126 0.8813 0.1192 0.0266 0.8182 0.5455 0.1765 0.5455
K7 0.8600 1.0000 0.6716 0.0693 1.0000 1.0000 0.3846 0.4545
K8 0.6690 0.5267 0.0998 0.0372 0.3590 0.1282 0.1458 0.4167
K9 0.8065 0.8387 0.1138 0.0430 1.0000 1.0000 0.0476 0.0556
mean 0.8776 0.9080 0.2367 0.0696 0.8808 0.8063 0.1689 0.3145
pages 311–322, Cham. Springer International Pub-
lishing.
Fawcett, T. (2006). An introduction to roc analysis. Pattern
Recogn. Lett., 27(8):861–874.
Gadatsch, A. (2017). Big Data – Datenanalyse als Ein-
trittskarte in die Zukunft, pages 1–10. Springer
Fachmedien Wiesbaden, Wiesbaden.
Goldstein, M. and Uchida, S. (2016). A comparative eval-
uation of unsupervised anomaly detection algorithms
for multivariate data. PLoS ONE, 11(4):1–31.
Hawkins, D. (1980). Identification of Outliers. Springer
Netherlands.
Hawkins, S., He, H., Williams, G., and Baxter, R. (2002).
Outlier detection using replicator neural networks. In
Kambayashi, Y. and Winiwarter, Werner, e. a., editors,
4th International Conference on Data Warehousing
and Knowledge Discovery, pages 170–180, Berlin,
Heidelberg. Springer Berlin Heidelberg.
Liu, F. T., Ting, K. M., and Zhou, Z.-H. (2008). Isolation
forest. In IEEE International Conference on Data
Mining 2008, ICDM ’08, pages 413–422, Washing-
ton, DC, USA. IEEE Computer Society.
Liu, F. T., Ting, K. M., and Zhou, Z.-H. (2012). Isolation-
based anomaly detection. ACM Trans. Knowl. Discov.
Data, 6(1):3:1–3:39.
Pahuja, D. and Yadav, R. (2013). Outlier detection for dif-
ferent applications:review. International Journal of
Engineering Research & Technology, 2.
Pimentel, M. A. F., Clifton, D. A., Clifton, L., and
Tarassenko, L. (2014). Review: A review of novelty
detection. Signal Process., 99:215–249.
Sch
¨
olkopf, B., Williamson, R. C., Smola, A. J., Shawe-
Taylor, J., and Platt, J. C. (2000). Support vector
method for novelty detection. In Solla, S. A., Leen,
T. K., and M
¨
uller, K., editors, Advances in Neural
Information Processing Systems 12, pages 582–588.
MIT Press.
Tax, D. M. J. and Duin, R. P. W. (2004). Support vector data
description. Mach. Learn., 54(1):45–66.
T
´
oth, L. and Gosztolya, G. (2004). Replicator neural net-
works for outlier modeling in segmental speech recog-
nition. In Yin, F.-L., Wang, J., and Guo, C., editors,
Advances in Neural Networks – ISNN 2004, pages
996–1001, Berlin, Heidelberg. Springer.
Winzker, M. (2017). Bussysteme in der Automobiltech-
nik, pages 175–179. Springer Fachmedien Wiesbaden,
Wiesbaden.
Isolation Forest for Anomaly Detection in Raw Vehicle Sensor Data
415