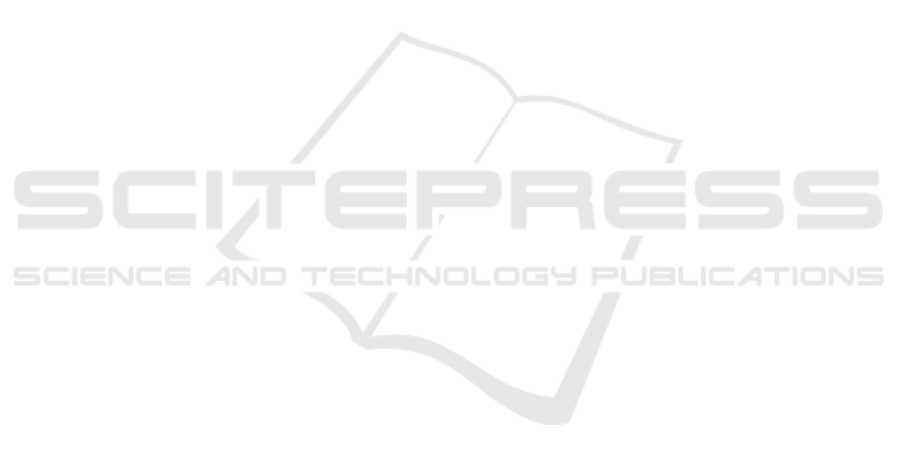
6 CONCLUSION
This article presented the SelfElastic model as an ad-
vance in the current state of research by offering the
aforementioned features both in terms of application
and parameter writing. SelfElastic offers hybrid elas-
ticity through the Live Thresholding technique, so
self-organizing threshold values and resource alloca-
tion to offer a competitive solution at performance
and cost levels. Although being developed for pa-
rallel applications, SelfElastic can be easily extended
to address elasticity adaptivity on Web-based servi-
ces including e-commerce and electronic funds trans-
fer. The results are encouraging in favor of using
Live Thresholding since LT presents performance and
costs very close or even better than static thresholds.
REFERENCES
Bing, H., Ying-lan, F., and e bai, L. Y. (2009). Research
and improvement of congestion control algorithms ba-
sed on tcp protocol. In Software Engineering, 2009.
WCSE ’09. WRI World Congress on, volume 1, pages
440–443.
Dustdar, S., Gambi, A., Krenn, W., and Nickovic, D.
(2015). A pattern-based formalization of cloud-based
elastic systems. In Proceedings of the Seventh In-
ternational Workshop on Principles of Engineering
Service-Oriented and Cloud Systems, PESOS ’15, pa-
ges 31–37, Piscataway, NJ, USA. IEEE Press.
Farokhi, S., Jamshidi, P., Brandic, I., and Elmroth, E.
(2015). Self-adaptation challenges for cloud-based
applications : A control theoretic perspective. In
10th International Workshop on Feedback Computing
(Feedback Computing 2015). ACM.
Galante, G. and Bona, L. C. E. D. (2015). A programming-
level approach for elasticizing parallel scientific appli-
cations. Journal of Systems and Software, 110:239 –
252.
Ghanbari, H., Simmons, B., Litoiu, M., and Iszlai, G.
(2011). Exploring alternative approaches to im-
plement an elasticity policy. In Cloud Computing
(CLOUD), 2011 IEEE International Conference on,
pages 716–723.
Herbst, N. R., Huber, N., Kounev, S., and Amrehn, E.
(2013). Self-adaptive workload classification and fo-
recasting for proactive resource provisioning. In Pro-
ceedings of the 4th ACM/SPEC International Confe-
rence on Performance Engineering, ICPE ’13, pages
187–198, New York, NY, USA. ACM.
Herbst, N. R., Kounev, S., Weber, A., and Groenda, H.
(2015). Bungee: An elasticity benchmark for self-
adaptive iaas cloud environments. In Proceedings of
the 10th International Symposium on Software Engi-
neering for Adaptive and Self-Managing Systems, SE-
AMS ’15, pages 46–56, Piscataway, NJ, USA. IEEE
Press.
Jamshidi, P., Ahmad, A., and Pahl, C. (2014). Auto-
nomic resource provisioning for cloud-based soft-
ware. In Proceedings of the 9th International Sym-
posium on Software Engineering for Adaptive and
Self-Managing Systems, SEAMS 2014, pages 95–104,
New York, NY, USA. ACM.
Lorido-Botran, T., Miguel-Alonso, J., and Lozano, J.
(2014). A review of auto-scaling techniques for elastic
applications in cloud environments. Journal of Grid
Computing, 12(4):559–592.
Moore, L. R., Bean, K., and Ellahi, T. (2013). Transfor-
ming reactive auto-scaling into proactive auto-scaling.
In Proceedings of the 3rd International Workshop on
Cloud Data and Platforms, CloudDP ’13, pages 7–12,
New York, NY, USA. ACM.
Netto, M. A. S., Cardonha, C., Cunha, R. L. F., and As-
suncao, M. D. (2014). Evaluating auto-scaling stra-
tegies for cloud computing environments. In IEEE
22nd International Symposium on Modelling, Analy-
sis & Simulation of Computer and Telecommunication
Systems, MASCOTS 2014, Paris, France, September
9-11, 2014, pages 187–196. IEEE.
Nikolov, V., K
¨
achele, S., Hauck, F. J., and Rautenbach,
D. (2014). Cloudfarm: An elastic cloud platform
with flexible and adaptive resource management. In
Proceedings of the 2014 IEEE/ACM 7th Internatio-
nal Conference on Utility and Cloud Computing, UCC
’14, pages 547–553, Washington, DC, USA. IEEE
Computer Society.
Nikravesh, A. Y., Ajila, S. A., and Lung, C.-H. (2015).
Towards an autonomic auto-scaling prediction system
for cloud resource provisioning. In Proceedings of the
10th International Symposium on Software Engineer-
ing for Adaptive and Self-Managing Systems, SEAMS
’15, pages 35–45, Piscataway, NJ, USA. IEEE Press.
Niu, S., Zhai, J., Ma, X., Tang, X., and Chen, W. (2013).
Cost-effective cloud hpc resource provisioning by
building semi-elastic virtual clusters. In Proceedings
of the International Conference on High Performance
Computing, Networking, Storage and Analysis, SC
’13, pages 56:1–56:12, New York, NY, USA. ACM.
Righi, R. R., Costa, C. A., Rodrigues, V. F., and Rostirolla,
G. (2016). Joint-analysis of performance and energy
consumption when enabling cloud elasticity for syn-
chronous hpc applications. Concurrency and Compu-
tation: Practice and Experience, 28(5):1548–1571.
Righi, R. R., Rodrigues, V. F., Costa, C. A., Galante, G.,
Bona, L., and Ferreto, T. (2015a). Autoelastic: Auto-
matic resource elasticity for high performance appli-
cations in the cloud. Cloud Computing, IEEE Tran-
sactions on, PP(99):1–1.
Righi, R. R., Rodrigues, V. F., Costa, C. A., Kreutz, D., and
Heiss, H.-U. (2015b). Towards cloud-based asynchro-
nous elasticity for iterative hpc applications. Journal
of Physics: Conference Series, 649(1):012006.
Triantaphyllou, E. (2000). Multi-Criteria Decision Making
Methodologies: A Comparative Study, volume 44 of
Applied Optimization. Springer, Dordrecht.
IoTBDS 2018 - 3rd International Conference on Internet of Things, Big Data and Security
268