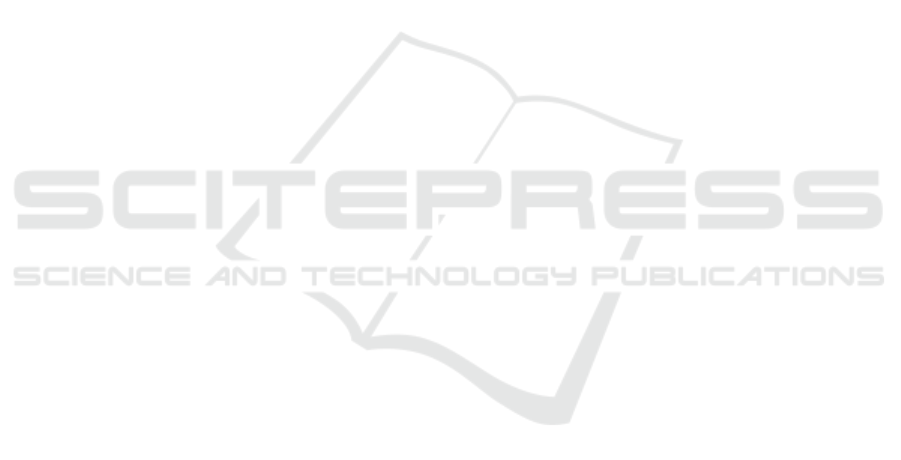
assess the ergonomics of in-vehicle information sys-
tems. In 2012 15th International IEEE Conference on
Intelligent Transportation Systems, pages 1809–1814.
Gonc¸alves, J. S. V., Rossetti, R. J. F., Jacob, J., Gonc¸alves,
J., Olaverri-Monreal, C., Coelho, A., and Rodrigues,
R. (2014). Testing advanced driver assistance sys-
tems with a serious-game-based human factors anal-
ysis suite. In 2014 IEEE Intelligent Vehicles Sympo-
sium Proceedings, pages 13–18.
Gupta, S., Vasardani, M., and Winter, S. (2016). Con-
ventionalized gestures for the interaction of people in
traffic with autonomous vehicles. In Proceedings of
the 9th ACM SIGSPATIAL International Workshop on
Computational Transportation Science, IWCTS ’16,
pages 55–60, New York, NY. ACM.
Hospedales, T., Gong, S., and Xiang, T. (2012). Video be-
haviour mining using a dynamic topic model. Inter-
national Journal of Computer Vision, 98(3):303–323.
Koo, J., Kwac, J., Ju, W., Steinert, M., Leifer, L., and
Nass, C. (2015). Why did my car just do that? ex-
plaining semi-autonomous driving actions to improve
driver understanding, trust, and performance. Interna-
tional Journal on Interactive Design and Manufactur-
ing (IJIDeM), 9(4):269–275.
Laznyi, K. and Marczi, G. (2017). Dispositional trust
x2014; do we trust autonomous cars? In 2017 IEEE
15th International Symposium on Intelligent Systems
and Informatics (SISY), pages 000135–000140.
Lehsing, C., Benz, T., and Bengler, K. (2016a). Insights
into interaction - effects of human-human interaction
in pedestrian crossing situations using a linked simu-
lator environment. IFAC-PapersOnLine, 49(19):138–
143.
Lehsing, C., Fleischer, M., and Bengler, K. (2016b). On the
track of social interaction - a non-linear quantification
approach in traffic conflict research. In 2016 IEEE
19th International Conference on Intelligent Trans-
portation Systems (ITSC), pages 2046–2051.
Lundgren, V., Habibovic, A., Andersson, J., Lagstrm, T.,
Nilsson, M., Sirkka, A., Fagerlnn, J., Fredriksson, R.,
Edgren, C., Krupenia, S., and Salur, D. (2017). Will
there be new communication needs when introducing
automated vehicles to the urban context? Advances in
Intelligent Systems and Computing, 484:485–497.
Macedo, J., Kokkinogenis, Z., Soares, G., Perrotta, D., and
Rossetti, R. J. F. (2013). A hla-based multi-resolution
approach to simulating electric vehicles in simulink
and sumo. In 16th Int. IEEE Conf. on Intelligent
Transportation Systems (ITSC), pages 2367–2372.
Morton, J., Wheeler, T. A., and Kochenderfer, M. J. (2017).
Analysis of recurrent neural networks for probabilistic
modeling of driver behavior. IEEE Transactions on
Intelligent Transportation Systems, 18(5):1289–1298.
Munigety, C. R. and Mathew, T. V. (2016). Towards behav-
ioral modeling of drivers in mixed traffic conditions.
Transportation in Developing Economies, 2(1):6.
Nagahama, A., Yanagisawa, D., and Nishinari, K. (2017).
Dependence of driving characteristics upon follower-
leader combination. Physica A: Statistical Mechanics
and its Applications, 483:503 – 516.
NHTSA (2012). Traffic safety facts 2010: A compila-
tion of motor vehicle crash data from the fatality
analysis reporting system and the general estimates
system. Technical report, National Highway Traffic
Safety Administration, Washington, DC.
Oh, C., Choi, J., and Park, S. (2017). In-depth understand-
ing of lane changing interactions for in-vehicle driving
assistance systems. International Journal of Automo-
tive Technology, 18(2):357–363.
Pereira, J. L. F. and Rossetti, R. J. F. (2012). An integrated
architecture for autonomous vehicles simulation. In
Proceedings of the 27th Annual ACM Symposium on
Applied Computing, SAC ’12, pages 286–292, New
York, NY, USA. ACM.
Perrotta, D., Macedo, J. L., Rossetti, R. J., de Sousa,
J. F., Kokkinogenis, Z., Ribeiro, B., and Afonso, J. L.
(2014). Route planning for electric buses: A case
study in oporto. Procedia - Social and Behavioral Sci-
ences, 111:1004 – 1014. Transportation: Can we do
more with less resources? 16th Meeting of the Euro
Working Group on Transportation Porto 2013.
Perrotta, D., Ribeiro, B., Rossetti, R. J., and Afonso, J. L.
(2012). On the potential of regenerative braking of
electric buses as a function of their itinerary. Procedia
- Social and Behavioral Sciences, 54:1156 – 1167.
Rossetti, R. J. F., Almeida, J. E., Kokkinogenis, Z., and
Gonc¸alves, J. (2013). Playing transportation seri-
ously: Applications of serious games to artificial
transportation systems. IEEE Intelligent Systems,
28(4):107–112.
Rothenbucher, D., Li, J., Sirkin, D., Mok, B., and Ju,
W. (2016). Ghost driver: A field study investigat-
ing the interaction between pedestrians and driver-
less vehicles. 25th IEEE International Symposium on
Robot and Human Interactive Communication, RO-
MAN 2016, pages 795–802.
Sucha, M., Dostal, D., and Risser, R. b. (2017). Pedestrian-
driver communication and decision strategies at
marked crossings. Accident Analysis and Prevention,
102:41–50.
United Kingdom Government. Tinted vehicle windows: the
law. https://www.gov.uk/tinted-vehicle-window-rules.
Last accessed: 2018-02-06.
Verberne, F. M. F., Ham, J., and Midden, C. J. H. (2012).
Trust in smart systems: Sharing driving goals and
giving information to increase trustworthiness and ac-
ceptability of smart systems in cars. Human Factors,
54(5):799–810. PMID: 23156624.
Xiaorui, W. and Hongxu, Y. (2013). A lane change model
with the consideration of car following behavior. Pro-
cedia Soc Behav Sci, 96:2354–2361.
VEHITS 2018 - 4th International Conference on Vehicle Technology and Intelligent Transport Systems
430