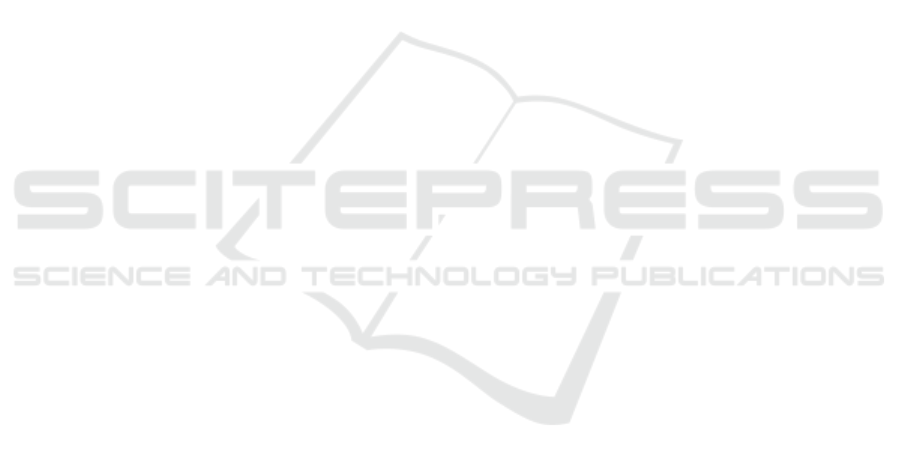
tomers participation and the effects of regulatory fra-
meworks in DR/DSM events. Consequently, Energy
Aggregators can evaluate their impact on the market-
place. This scenario is a long-term planning activity.
Thus, simulations needs monthly or yearly time reso-
lution. This scenario exploits the following modules:
i) Agent-Based Model for Market Impacts, ii) Hou-
sehold Electricity Behaviour , iii) Smart Metering In-
frastructure, iv) Photovoltaic Energy, v) Smart Grid
Simulator and vi) Thermal Building Simulator.
City managers can use MESsi to evaluate the so-
lar potential and its impact on the distribution net-
work considering also load profiles and network con-
strains. This scenario involves the following modules:
i) Photovoltaic Energy to estimate the generation pro-
file for each PV system installed in building rooftops;
ii) Smart Metering Infrastructure to retrieve real load
profiles or Household Electricity Behaviour to gene-
rate realistic energy consumption patterns, iii) Solar
Thermal Collector to simulate the behaviours of so-
lar thermal panels in heating water for domestic use
or for heat pumps. If the simulation scenario inclu-
des building heating systems supplied by solar ther-
mal panels, the Thermal Building Simulator is needed
to evaluate the impact on indoors temperature beha-
viours. The scenarios needs monthly or yearly time
resolution.
MESsi can also be used for testing or validating
already existing algorithms, such as Non-Intrusive
Load Monitoring (NILM). NILM is a signal proces-
sing technique, which discerns the energy consump-
tion of the appliances from the aggregated data acqui-
red from a single point of measurement, i.e. the Smart
Meter (Zoha et al., 2012). In this case, the input nee-
ded by the NILM algorithm are historical households
load profiles retrieved from Smart Metering Infra-
structure. As an alternative, the NILM service can ex-
ploit the Household Electricity Behaviour module to
create realistic electrical consumption patterns. Time
resolution for this scenario ranges from microseconds
to 1 second.
It is worth noting that, thanks to the microservice
design pattern, MESsi is opened to build and run new
simulation scenarios to meet latest requirements from
the end-users.
5 CONCLUSION
In this paper, we presented MESsi, which is a no-
vel distributed infrastructure for modelling and co-
simulating Multi-Energy-Systems in cities. First, we
discussed the motivations and challenges we addres-
sed to design such infrastructure. Then, we intro-
duced our proposed framework that is suitable for
general purpose energy simulations with different
spatio-temporal resolutions. MESsi combines dif-
ferent technologies and correlates heterogeneous in-
formation, also sent in (near-) real-time, to simu-
late multi-energy-flows and to evaluate the impact
of novel policies in cities and distribution networks.
Finally, we discussed possible simulation scenarios
i) for analysing the operational status of energy dis-
tribution systems, ii) for planning and refurbishment
activities, and iii) for testing or validating already ex-
isting algorithms.
REFERENCES
Abrishambaf, O., Faria, P., Gomes, L., Sp
´
ınola, J., Vale, Z.,
and Corchado, J. M. (2017). Implementation of a real-
time microgrid simulation platform based on centrali-
zed and distributed management. Energies, 10(6):806.
Acquaviva, A., Apiletti, D., Attanasio, A., Baralis, E., Bot-
taccioli, L., Castagnetti, F. B., Cerquitelli, T., Chi-
usano, S., Macii, E., Martellacci, D., et al. (2015).
Energy signature analysis: Knowledge at your finger-
tips. In Big Data (BigData Congress), 2015 IEEE In-
ternational Congress on, pages 543–550. IEEE.
Allegrini, J., Orehounig, K., Mavromatidis, G., Ruesch,
F., Dorer, V., and Evins, R. (2015). A review of
modelling approaches and tools for the simulation of
district-scale energy systems. Renewable and Sustai-
nable Energy Reviews, 52:1391–1404.
Anderson, K., Du, J., Narayan, A., and El Gamal, A. (2014).
Gridspice: A distributed simulation platform for the
smart grid. IEEE Transactions on Industrial Informa-
tics, 10(4):2354–2363.
Baetens, R., De Coninck, R., Jorissen, F., Picard, D., Hel-
sen, L., and Saelens, D. (2015). Openideas-an open
framework for integrated district energy simulations.
In Proceedings of Building Simulation 2015.
Bollinger, L. A. and Evins, R. (2015). Facilitating model
reuse and integration in an urban energy simulation
platform. Procedia Computer Science, 51:2127–2136.
Bottaccioli, L., Aliberti, A., Ugliotti, F., Patti, E., Osello,
A., Macii, E., and Acquaviva, A. (2017a). Building
energy modelling and monitoring by integration of iot
devices and building information models. In Com-
puter Software and Applications Conference (COMP-
SAC), 2017 IEEE 41st Annual, volume 1, pages 914–
922. IEEE.
Bottaccioli, L., Estebsari, A., Pons, E., Bompard, E., Macii,
E., Patti, E., and Acquaviva, A. (2017b). A flexible
distributed infrastructure for real-time co-simulations
in smart grids. IEEE Transactions on Industrial Infor-
matics.
Bottaccioli, L., Patti, E., Macii, E., and Acquaviva, A.
(2017c). Gis-based software infrastructure to model
pv generation in fine-grained spatio-temporal domain.
IEEE Systems Journal.
SMARTGREENS 2018 - 7th International Conference on Smart Cities and Green ICT Systems
268