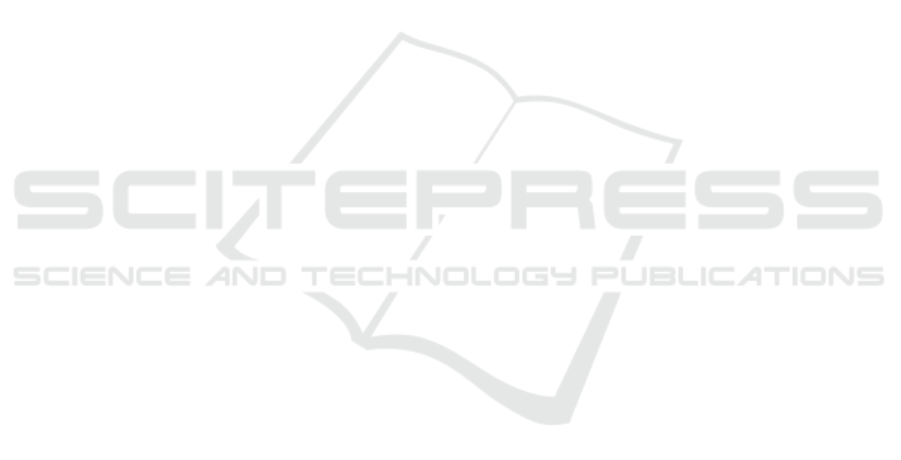
Caron, E., Desprez, F., Glatard, T., Ketan, M., Montag-
nat, J., and Reimert, D. (2010). Workflow-based
comparison of two distributed computing infrastruc-
tures. In Workflows in Support of Large-Scale Science
(WORKS10), New Orleans. In Conjunction with Su-
percomputing 10 (SC’10), IEEE. hal-00677820.
Chai, L. E., Loh, S. K., Low, S. T., Mohamad, M. S., Deris,
S., and Zakaria, Z. (2014). A review on the compu-
tational approaches for gene regulatory network con-
struction. Comput Biol Med, 48:55–65.
Couvares, P., Kosar, T., Roy, A., Weber, J., and Wenger, K.
(2007). Workflow Management in Condor. In Taylor,
I., Deelman, E., Gannon, D., and Shields, M., editors,
Workflows for e-Science, pages 357–375. Springer.
Das, A. K., Koppa, P. K., Goswami, S., Platania, R., and
Park, S. J. (2017). Large-scale parallel genome assem-
bler over cloud computing environment. J Bioinform
Comput Biol, 15(3):1740003.
Deelman, E., Singh, G., Su, M.-H., Blythe, J., Gil, Y.,
Kesselman, C., Mehta, G., Vahi, K., Berriman, G. B.,
Good, J., Laity, A., Jacob, J., and Katz, D. (2005). Pe-
gasus: a Framework for Mapping Complex Scientific
Workflows onto Distributed Systems. Scientific Pro-
gramming Journal, 13(3):219–237.
Heinis, T., Pautasso, C., and Alonso, G. (2005). Design
and evaluation of an autonomic workflow engine. In
Autonomic Computing, 2005. ICAC 2005. Proceed-
ings. Second International Conference on, pages 27–
38. IEEE.
Herbach, U., Bonnaffoux, A., Espinasse, T., and Gan-
drillon, O. (2017). Inferring gene regulatory net-
works from single-cell data: a mechanistic approach.
https://arxiv.org/abs/1705.03407.
Ilyushkin, A., Ali-Eldin, A., Herbst, N., Papadopoulos,
A. V., Ghit, B., Epema, D., and Iosup, A. (2017).
An experimental performance evaluation of autoscal-
ing policies for complex workflows. In Binder, W.,
Cortellessa, V., Koziolek, A., Smirni, E., and Poess,
M., editors, ICPE, pages 75–86. ACM.
Kailasam, S., Gnanasambandam, N., Dharanipragada, J.,
and Sharma, N. (2010). Optimizing service level
agreements for autonomic cloud bursting schedulers.
In Parallel Processing Workshops (ICPPW), 2010
39th International Conference on, pages 285–294.
IEEE.
Kephart, J. O. and Chess, D. M. (2003). The vision of auto-
nomic computing. Computer, 36(1):41–50.
Kreutz, C. and Timmer, J. (2009). Systems biology: exper-
imental design. FEBS J, 276(4):923–42.
Lee, W. P., Hsiao, Y. T., and Hwang, W. C. (2014). De-
signing a parallel evolutionary algorithm for inferring
gene networks on the cloud computing environment.
BMC Syst Biol, 8:5.
Londo
˜
no-Pel
´
aez, J. M. and Florez-Samur, C. A. (2013). An
autonomic auto-scaling controller for cloud based ap-
plications. International Journal of Advanced Com-
puter Science and Applications(IJACSA), 4(9).
Mao, M. and Humphrey, M. (2013). Scaling and schedul-
ing to maximize application performance within bud-
get constraints in cloud workflows. In Parallel & Dis-
tributed Processing (IPDPS), 2013 IEEE 27th Inter-
national Symposium on, pages 67–78. IEEE.
Mao, M., Li, J., and Humphrey, M. (2010). Cloud auto-
scaling with deadline and budget constraints. In Grid
Computing (GRID), 2010 11th IEEE/ACM Interna-
tional Conference on, pages 41–48. IEEE.
Nikravesh, A. Y., Ajila, S. A., and Lung, C.-H. (2015).
Towards an autonomic auto-scaling prediction system
for cloud resource provisioning. In Inverardi, P. and
Schmerl, B. R., editors, 10th IEEE/ACM International
Symposium on Software Engineering for Adaptive and
Self-Managing Systems, SEAMS 2015, Florence, Italy,
May 18-19, 2015, pages 35–45. IEEE Computer Soci-
ety.
Pandey, S., Voorsluys, W., Niu, S., Khandoker, A., and
Buyya, R. (2012). An autonomic cloud environment
for hosting ecg data analysis services. Future Gener-
ation Computer Systems, 28(1):147–154.
Peccoud, J. and Ycart, B. (1995). Markovian modelling of
gene product synthesis. Theoretical population biol-
ogy, 48:222–234.
Pina, C., Fugazza, C., Tipping, A. J., Brown, J., Soneji, S.,
Teles, J., Peterson, C., and Enver, T. (2012). Inferring
rules of lineage commitment in haematopoiesis. Nat
Cell Biol, 14(3):287–94.
Richard, A., Boullu, L., Herbach, U., Bonnafoux, A.,
Morin, V., Vallin, E., Guillemin, A., Papili Gao,
N., Gunawan, R., Cosette, J., Arnaud, O., Kupiec,
J. J., Espinasse, T., Gonin-Giraud, S., and Gandrillon,
O. (2016). Single-cell-based analysis highlights a
surge in cell-to-cell molecular variability preceding
irreversible commitment in a differentiation process.
PLoS Biol, 14(12):e1002585.
Xiao, X., Zhang, W., and Zou, X. (2015). A
new asynchronous parallel algorithm for inferring
large-scale gene regulatory networks. PLoS One,
10(3):e0119294.
Yu, J., Blom, J., Sczyrba, A., and Goesmann, A. (2017).
Rapid protein alignment in the cloud: Hamond com-
bines fast diamond alignments with hadoop paral-
lelism. J Biotechnol.
Zheng, G., Xu, Y., Zhang, X., Liu, Z. P., Wang, Z., Chen,
L., and Zhu, X. G. (2016). Cmip: a software pack-
age capable of reconstructing genome-wide regulatory
networks using gene expression data. BMC Bioinfor-
matics, 17(Suppl 17):535.
CLOSER 2018 - 8th International Conference on Cloud Computing and Services Science
516