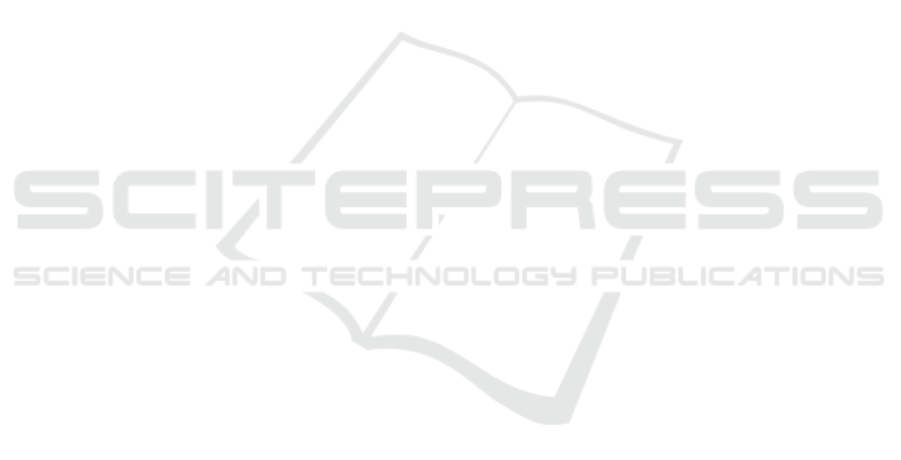
5 FUTURE WORK
The EDA signal is controlled by the limbic system
(Fausett, 1994), which also generates the approval
and disapproval responses, defining our choices and
actions (Boucsein, 2012b). Proper modelling of this
behaviour can generate interesting solutions for
people with such severe physical limitations that they
cannot express their needs and feelings. Since the
electronic circuit used in this work has a low cost, the
use of EDA can, more quickly than conventional BCI
using EEG, generate solutions that reach a larger part
of the population.
Other studies found in the literature related the use
of the EDA signal to correct the commands generated
by the EEG signal (Boucsein, 2012a), but we have
shown in this work that the training time of a BCI
application can be reduced by using the EDA signal
instead of the EEG. In addition, the technology
developed by our research group, which included the
design and development of a custom acquisition
circuit, can reduce the cost of this type of BCI
application, opening possibilities for its use in other
fields of research. While a wifi EEG headset plus
electrodes could cost almost U$800.00, an EDA
detector can be bought by only U$10.00.
The choice for the ANN paradigm for signal
recognition was also a good decision. As we
predefine the network architecture and training
parameters, and parameterize the training process,
potential users of our BCI system do not need any
technical knowledge to learn how to use it.
For a future work, it will be interesting to explore
the limits of the EDA signal applied to BCI, such as
collecting EDA signals from more than one region,
for example, from the right and left hand at the same
time. The combination of these signals could increase
the variety of responses and, consequently, the
number of possible BCI applications. Tsukahana
(2002) presents another approach for electrodermal
signal codification, generating more than one binary
signal to increase the choices of movements for the
user.
REFERENCES
Amaral, J. R. Homepage, http://www.cerebromente.org.br/
n05/mente/limbic.htm, last accessed in 10/21/2016,
2016.
Blain, S.; Mihailidis, Al.; Chau, T. Assessing the Potential
of EDA Activity as an Alternative Access Pathway. In
Medical Engineering Physics, pp 498-505, 2008.
Boucsein, W. Principles of Electrodermal Phenomena in:
Eletrodermal Activity. Second Edition: New York:
Springer, pp. 1-76, 2012a.
Boucsein, W. EDA Activity, Second Edition. In: Springer
Science+Business Media, 2012b.
Fausett, L. Fundamentals of Neural Networks –
Arquitetures, Algorithms and applications, 1nd Edition,
Prentice-Hall, pp. 1-37, 59-76, 1994.
Iacoviello, D.: A Real-time Classification Algorithm for
EEG - Based BCI driven by Self-induced emotions. In:
Computer Methods and Programs in Biomedicine, vol.
122, pp. 293–303, 2015.
Jun, J.; et al.: A novel Morse code inspired method for
multiclass motor imagery brain–computer interface
(BCI) design. In: Computers in Biology and Medicine,
pp. 11–19, 2015.
Leskov, I. F., Lopes, H.: Amplificador e Filtros de Baixo
Custo para Sinais de EEG (Eletroencefalografia). In:
Simpósio Internacional de Iniciação Científica da USP,
São Carlos. XVII Congresso de Iniciação Científica e
Tecnológica em Engenharia, 2000.
Lin, J. S., Hsieh, A.: A Wireless BCI-Controlled Integration
System in Smart Living Space for Patients. In: CH.
Wireless Pers Commun, vol. 88: 395. doi:10.1007/
s11277-015-3129-0, 2016.
Mathworks. Homepage: https://www.mathworks.com/
help/nnet/ug/multilayer-neural-network-
architecture.html, last accessed in 2016/08/15.
Najarian, Kayvan; Splinter, Robert. (s.d.).
Electroencephalogram in: Biomedical Signal and
Image Processing, CRC Press – Taylor & Francis
Group, pp 197-216, 2006.
Narendra, Kumpati S. Adaptative Control Using Neural
Networks. In: SUTTON, Richard S.; WERBOS, Paul
J.; MILLER III, W. Thomas,“Neural Networks for
Control”, MIT Press, vol. 211996. pp.115–142.
Nishida, S. M.: Neurobiologia das emoções - SISTEMA
LÍMBICO, Homepage, http://www.ibb.unesp.
br/Home/Departamentos/Fisiologia/Neuro/aula27.siste
ma_limbico_silvia.pdf, last accessed in 10/28/2016,
2016.
Noteboom, J. T.: Activation of the arousal response can
impair performance on a simple motor task. In: Journal
of Applied Physiology, vol. 91, pp.:821-831, 2001.
Oliveira, K. S., Homepage: http://astro.if.ufrgs.br/
med/imagens/fourier.htm, last accessed 06/28/2017,
2017.
Plonsey, J. M.: Bioelectromagnetism. In: J. Malmivuo,
Bioelectromagnetism - Principles and Applications of
Bioelectric and Biomagnetic Fields, New York: Oxford
University Press, cap 13, 1995.
Spliter, K. N. In: Biomedical Signal and Image Processing,
CRC Press - Taylor & Francis Group, pp 79-99, 2006.
Tsukahana, Reiko, Aoki, Hisashi. Skin potential response
in letter recognition task as an alternative
communication channel for individuals with severe
motor disability. Clinical Neurophysiology 2002, pp
1723–1733.
ICEIS 2018 - 20th International Conference on Enterprise Information Systems
538