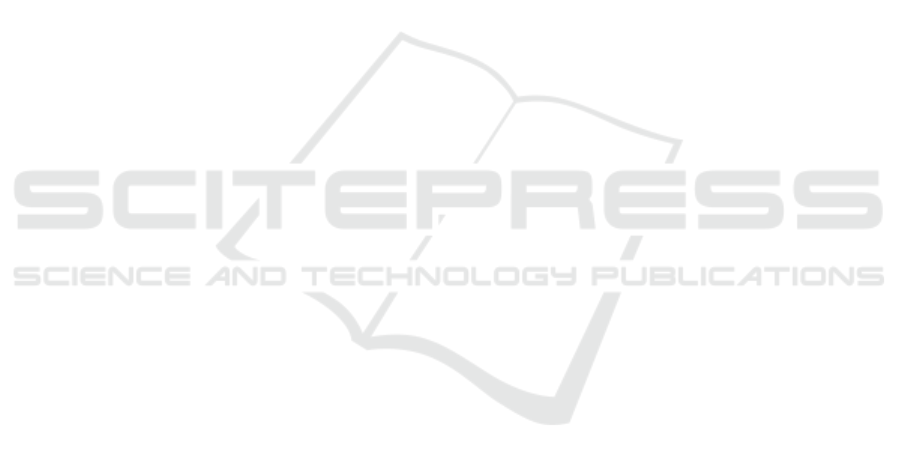
proposed a technique to similar group customers for
forecasting their energy profiles (Albert and Rajago-
pal, 2013).
Queuing-Based energy consumption management
system for residential smart gird is proposed by Yi Liu
et al. (Liu et al., 2016). Mainly there are two types
of demands, essential and flexible demands. Flexible
demands includes delay-sensitive and delay-tolerant
loads. These loads can be controled by residential
smart grid directly and scheduled accordingly. Which
means they need extra resources for controlling these
appliances, for millions of customer it’s not a feasible
solution.
6 CONCLUSION AND FUTURE
WORK
We have introduced SLS to overcome the issues with
conventional DR and DSM techniques and to manage
the variability factor of renewable energy resources.
The main idea behind SLS is to manage the variabi-
lity factor of renewable resources while keeping fair-
ness and customer inconvenience in view. Our future
work involves finding the most suitable balance be-
tween forcing and requesting the customers to shed
their energy usage at the time of need. Moreover,
since SLS depends on the forecasting of individual
customers’ energy profiles, we will be working to im-
prove this forecasting. While consumer level forecas-
ting techniques claim to have 80% accuracy, our goal
is to use contextual information from customers to im-
prove forecasting further. Smart grid testbed (Tushar
et al., 2016) could be use to test our solution and will
help us to imporve our technique.
REFERENCES
Aalami, H., Moghaddam, M. P., and Yousefi, G. (2010).
Demand response modeling considering interrupti-
ble/curtailable loads and capacity market programs.
Applied Energy, 87(1):243–250.
Albert, A. and Rajagopal, R. (2013). Smart meter dri-
ven segmentation: What your consumption says
about you. IEEE Transactions on power systems,
28(4):4019–4030.
Ardakanian, O., Koochakzadeh, N., Singh, R. P., Golab,
L., and Keshav, S. (2014). Computing electricity con-
sumption profiles from household smart meter data. In
EDBT/ICDT Workshops, volume 14, pages 140–147.
Bashir, N., Sharani, Z., Qayyum, K., and Syed, A. A.
(2015). Delivering smart load-shedding for highly-
stressed grids. In Smart Grid Communications
(SmartGridComm), 2015 IEEE International Confe-
rence on, pages 852–858. IEEE.
Chandan, V., Ganu, T., Wijaya, T. K., Minou, M., Stamou-
lis, G., Thanos, G., and Seetharam, D. P. (2014). idr:
Consumer and grid friendly demand response system.
In Proceedings of the 5th international conference on
Future energy systems, pages 183–194. ACM.
Connolly, D., Lund, H., Mathiesen, B., and Leahy, M.
(2011). The first step towards a 100% renewable
energy-system for ireland. Applied Energy, 88(2):502
– 507. The 5th Dubrovnik Conference on Sustainable
Development of Energy, Water and Environment Sys-
tems, held in Dubrovnik September/October 2009.
Cosic, B., Krajacic, G., and Duic, N. (2012). A
100% renewable energy system in the year 2050:
The case of macedonia. Energy, 48(1):80 – 87.
6th Dubrovnik Conference on Sustainable Develop-
ment of Energy Water and Environmental Systems,
{SDEWES} 2011.
Craciun, D., Ichim, S., and Besanger, Y. (2009). A new soft
load shedding: Power system stability with contribu-
tion from consumers. PowerTech, 2009 IEEE Bucha-
rest, pages 1–6.
Ghalehkhondabi, I., Ardjmand, E., Weckman, G. R., and
Young, W. A. (2017). An overview of energy demand
forecasting methods published in 2005–2015. Energy
Systems, 8(2):411–447.
Iyengar, S., Lee, S., Irwin, D., and Shenoy, P. (2016). Ana-
lyzing energy usage on a city-scale using utility smart
meters. In Proceedings of the 3rd ACM Internatio-
nal Conference on Systems for Energy-Efficient Built
Environments, pages 51–60. ACM.
Laghari, J., Mokhlis, H., Bakar, A., and Mohamad, H.
(2013). Application of computational intelligence
techniques for load shedding in power systems: A re-
view. Energy Conversion and Management, 75:130 –
140.
Liu, Y., Yuen, C., Yu, R., Zhang, Y., and Xie, S. (2016).
Queuing-based energy consumption management for
heterogeneous residential demands in smart grid.
IEEE Transactions on Smart Grid, 7(3):1650–1659.
Miettinen, J. J. and Holttinen, H. (2017). Characteristics
of day-ahead wind power forecast errors in nordic
countries and benefits of aggregation. Wind Energy,
20(6):959–972. WE-15-0321.R2.
Mohd, A., Ortjohann, E., Schmelter, A., Hamsic, N., and
Morton, D. (2008). Challenges in integrating distribu-
ted energy storage systems into future smart grid. In
Industrial Electronics, 2008. ISIE 2008. IEEE Inter-
national Symposium on, pages 1627–1632. IEEE.
Roberts, B. P. and Sandberg, C. (2011). The role of energy
storage in development of smart grids. Proceedings of
the IEEE, 99(6):1139–1144.
Tushar, W., Yuen, C., Chai, B., Huang, S., Wood, K. L.,
Kerk, S. G., and Yang, Z. (2016). Smart grid test-
bed for demand focused energy management in end
user environments. IEEE Wireless Communications,
23(6):70–80.
Vytelingum, P., Voice, T. D., Ramchurn, S. D., Rogers,
A., and Jennings, N. R. (2010). Agent-based micro-
storage management for the smart grid. In Procee-
dings of the 9th International Conference on Auto-
nomous Agents and Multiagent Systems: Volume 1-
Volume 1, pages 39–46. International Foundation for
Autonomous Agents and Multiagent Systems.
Soft Load Shedding: An Efficient Approach to Manage Electricity Demand in a Renewable Rich Distribution System
107